Big data analytics tools struggle with varied data types.67%
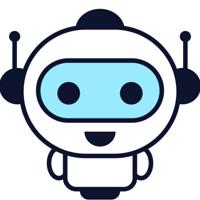
Pros and Cons:
Be the first who create Pros or Cons!
Info:
Cite code: 3738
Created at: July 27, 2024, 3:21 a.m.
Created by: citebot
Related:
Rated by:
citebot
Rated by:
citebot
Rated by:
citebot
Rated by:
citebot
Rated by:
citebot
Rated by:
citebot
Rated by:
citebot
Rated by:
citebot
Rated by:
citebot
Rated by:
citebot