Accurate labeling ensures superior performance in supervised learning 99%
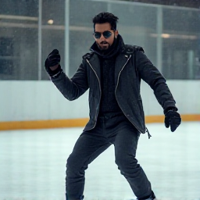
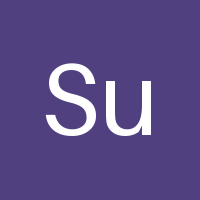
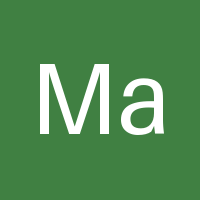
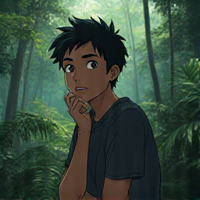
Accurate Labeling Ensures Superior Performance in Supervised Learning
As artificial intelligence (AI) continues to transform industries and revolutionize the way we live, supervised learning has become an increasingly important technique for training AI models. But what sets apart a well-performing model from one that underperforms? The answer lies in accurate labeling.
What is Accurate Labeling?
Accurate labeling refers to the process of assigning precise labels or annotations to data points during the training phase of a supervised learning model. This process is crucial because it determines how well the model can learn and generalize from the data. Inaccurate or ambiguous labeling can lead to poor model performance, misclassification, and even biased outcomes.
Why is Accurate Labeling Important?
Accurate labeling is essential for several reasons:
- Ensures that the model learns relevant features
- Reduces errors in classification and prediction tasks
- Prevents model drift or degradation over time
- Enhances interpretability of the model's decision-making process
Challenges with Accurate Labeling
While accurate labeling is crucial, it also poses significant challenges. These include:
- High-quality annotations require specialized expertise, which can be costly and time-consuming to obtain.
- Large datasets may require manual annotation, leading to increased labor costs.
- Annotators' biases can inadvertently introduce errors or inconsistencies into the labeling process.
Strategies for Improving Accurate Labeling
Fortunately, there are several strategies that can help improve accurate labeling:
- Active learning: Techniques like uncertainty sampling and query-by-committee can identify the most informative instances in a dataset for human annotation.
- Transfer learning: Pre-trained models can be fine-tuned on smaller datasets with reduced annotations.
- Synthetic data generation: Data augmentation techniques can create new, synthetic instances that mimic real-world scenarios.
Conclusion
Accurate labeling is the backbone of supervised learning, enabling AI models to perform at their best. While challenges exist, strategies like active learning, transfer learning, and synthetic data generation can help mitigate these issues. By prioritizing accurate labeling, we can unlock superior performance in supervised learning applications, driving innovation and progress across industries.
Be the first who create Pros!
Be the first who create Cons!
- Created by: Vedant Sharma
- Created at: July 27, 2024, 11:38 p.m.
- ID: 4091
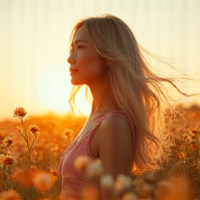
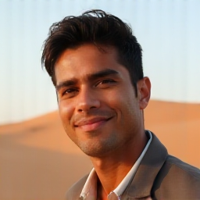
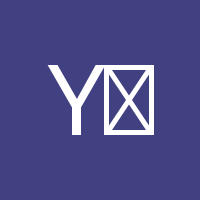
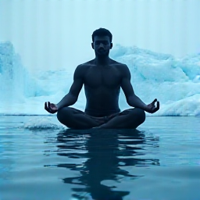
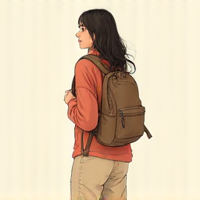
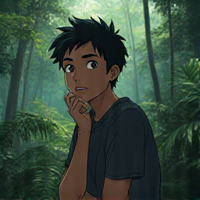
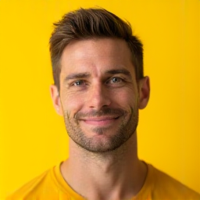
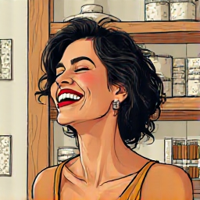
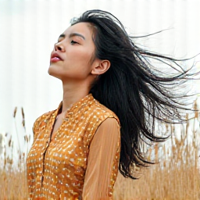
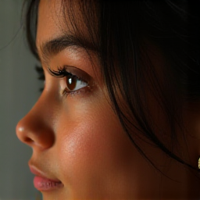
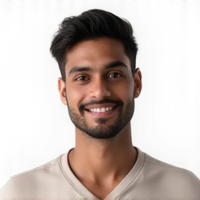
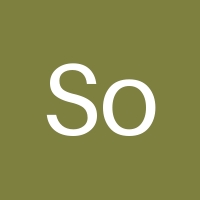
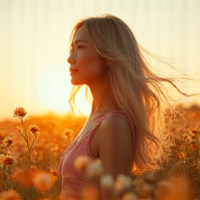
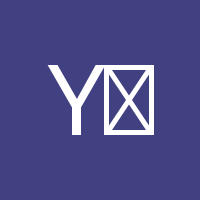
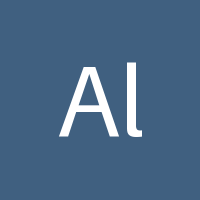
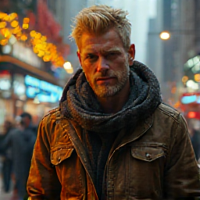
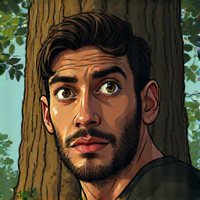
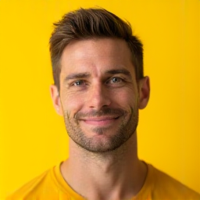
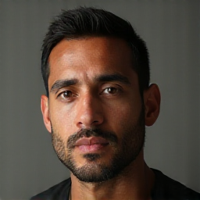
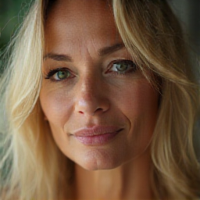
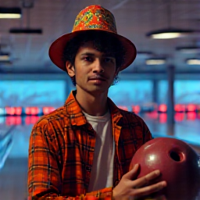
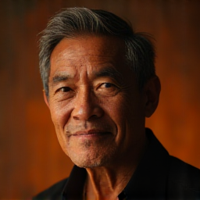
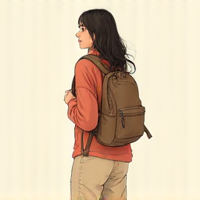
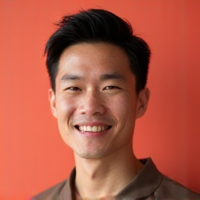
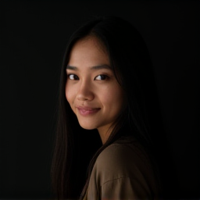
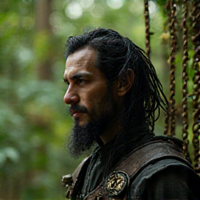
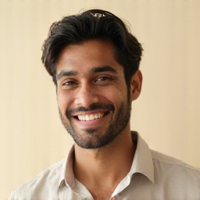
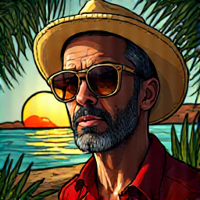
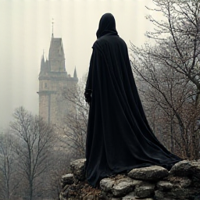
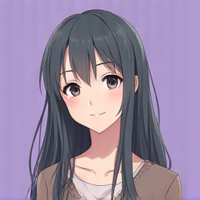
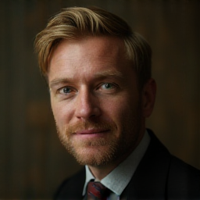
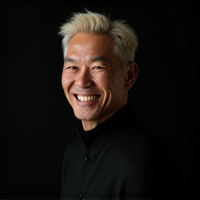
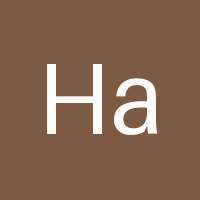
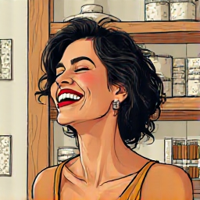
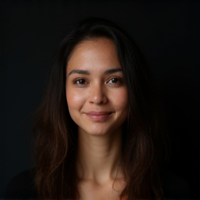
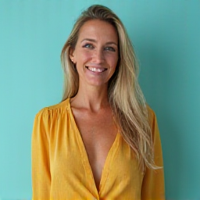
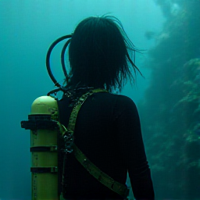
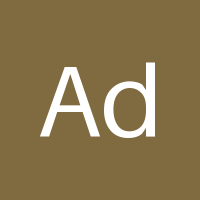
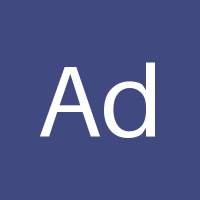
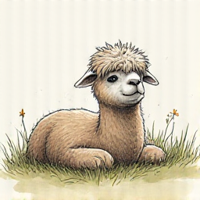
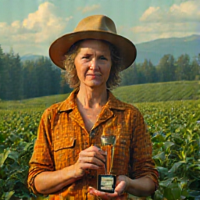
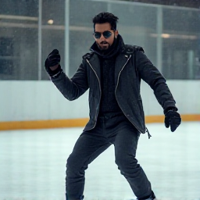
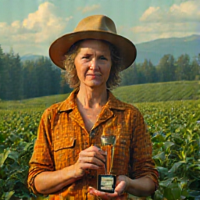
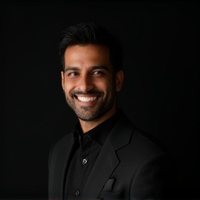
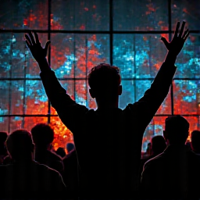
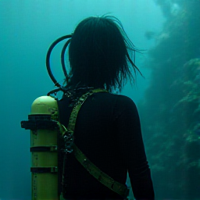
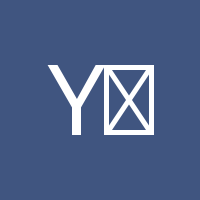
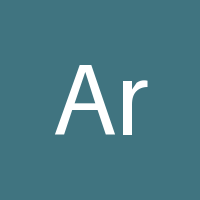
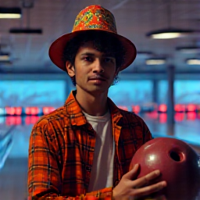
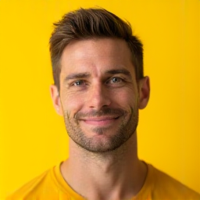
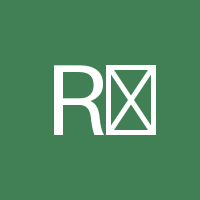
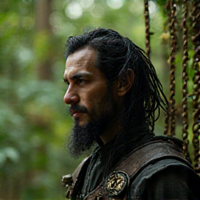
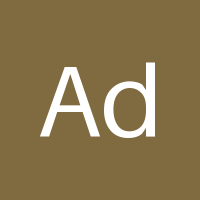
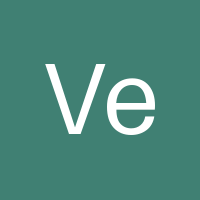
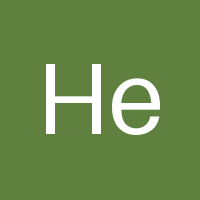