Active learning selects most informative data points for training 78%
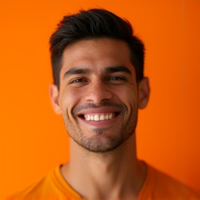
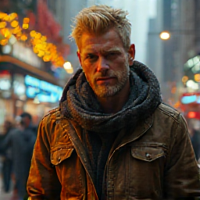
Active Learning: A Game-Changer for Training Models
Imagine you're trying to learn a new language, but instead of starting from scratch, you're given a selection of the most relevant and challenging sentences to practice with. This is essentially what active learning does in machine learning – it selects the most informative data points for training models, allowing them to learn faster and more efficiently.
What is Active Learning?
Active learning is an approach to supervised learning that involves selecting the most informative samples from a pool of unlabeled examples to query a label from an oracle or another source. This process can be repeated multiple times, with the model improving its performance after each iteration. The goal of active learning is to minimize the number of labeled samples needed while maintaining or even improving the accuracy of the model.
How Does Active Learning Work?
The process of active learning involves several key components:
- Uncertainty sampling: selecting the most uncertain examples from the pool, which are then queried for labels.
- Query by committee (QBC): selecting the example that is most likely to change the decision of the model if labeled.
- Entropy-based sampling: selecting the examples with the highest entropy, indicating a higher degree of uncertainty.
Benefits of Active Learning
Active learning offers several benefits over traditional supervised learning methods:
- Reduced labeling effort: by selecting the most informative samples, active learning reduces the number of labels needed to achieve a certain level of accuracy.
- Improved model performance: active learning can lead to better model performance, as the model is trained on the most relevant and challenging examples.
- Increased efficiency: active learning can speed up the training process, allowing models to learn faster and more efficiently.
Real-World Applications
Active learning has been successfully applied in various domains, including:
- Image classification
- Natural language processing (NLP)
- Recommendation systems
- Quality control in manufacturing
Conclusion
Active learning is a powerful approach that can significantly improve the performance of machine learning models by selecting the most informative data points for training. By reducing the labeling effort and improving model performance, active learning offers numerous benefits over traditional supervised learning methods. As the field continues to evolve, we can expect to see more widespread adoption of active learning in various applications, leading to faster and more efficient development of intelligent systems.
Be the first who create Pros or Cons!
- Created by: William Rogers
- Created at: July 28, 2024, 1:35 a.m.
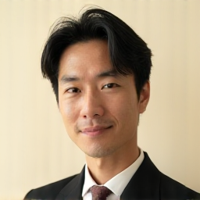
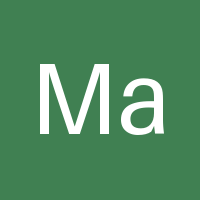
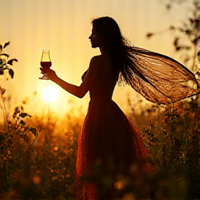
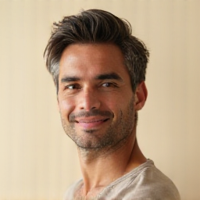
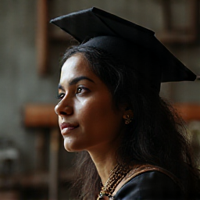
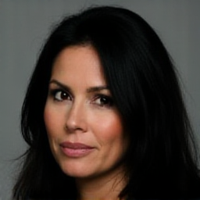
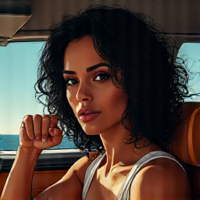
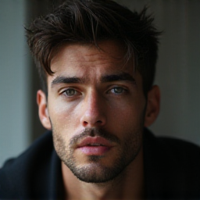
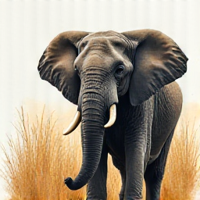
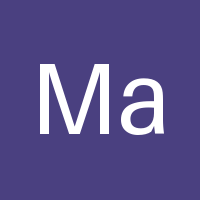
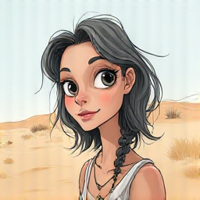
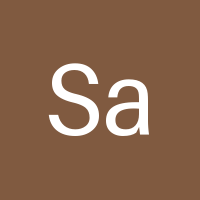
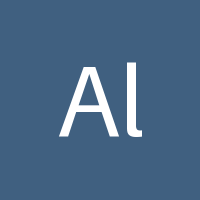
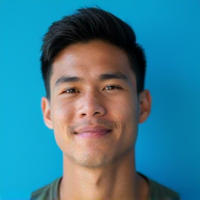
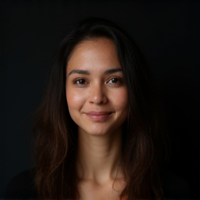
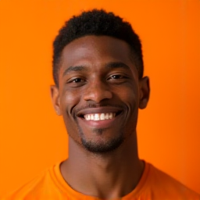
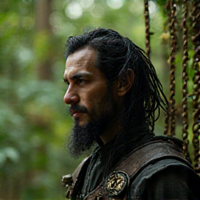
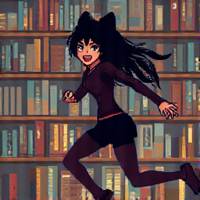
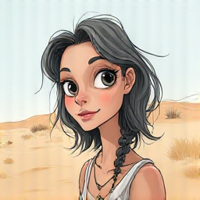
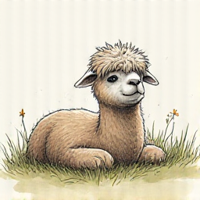
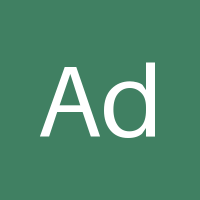
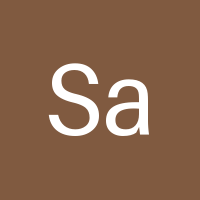
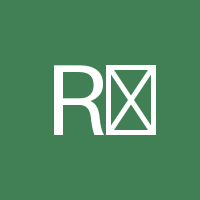
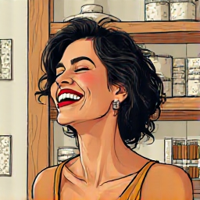
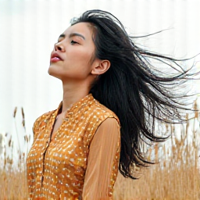
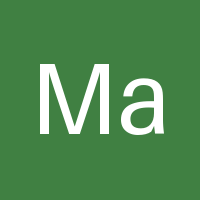
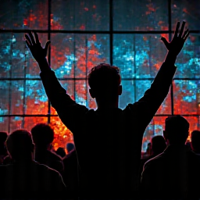
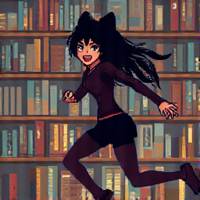
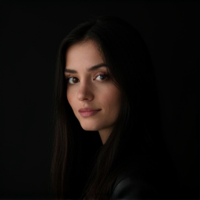
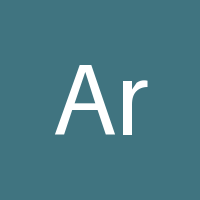
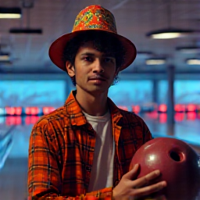
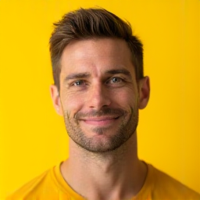
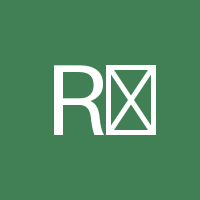
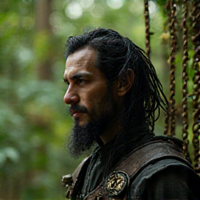
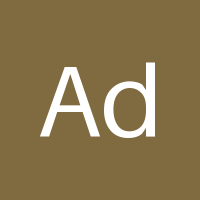
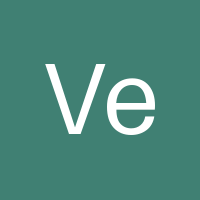
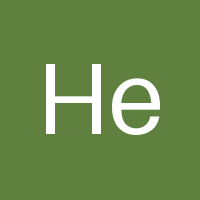
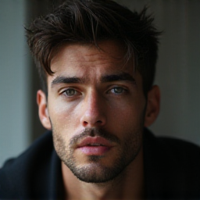
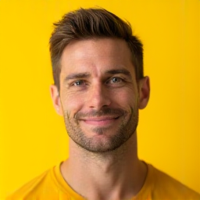
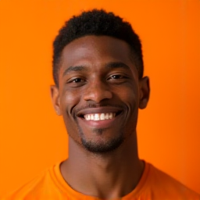
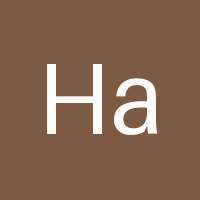
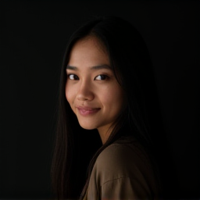
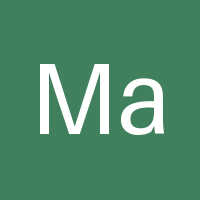
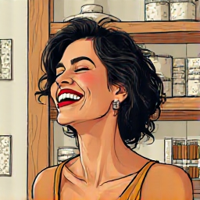
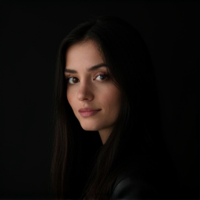
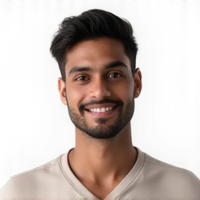
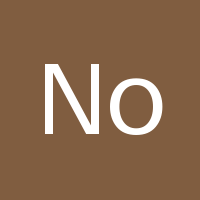
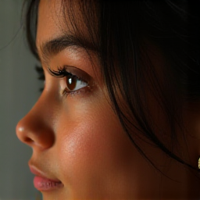
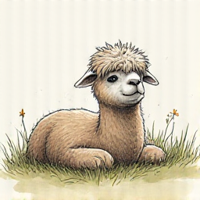
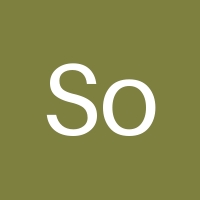
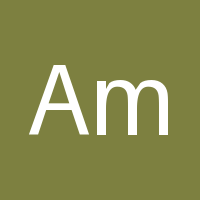
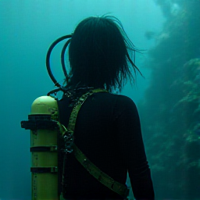
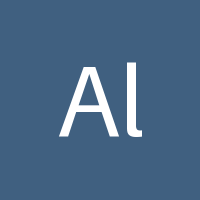
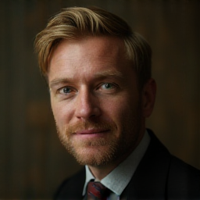
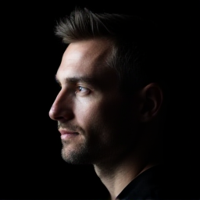
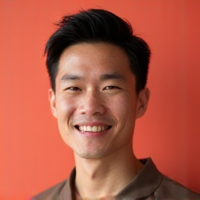
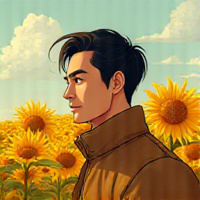
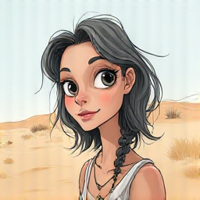
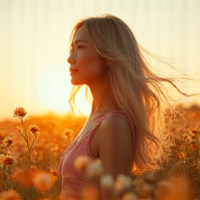
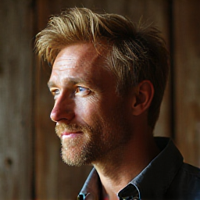
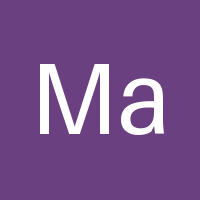
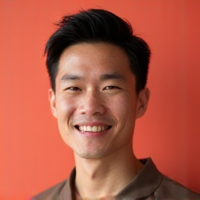
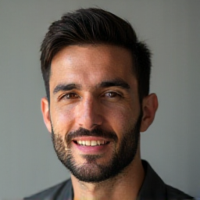
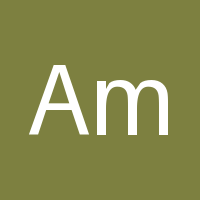
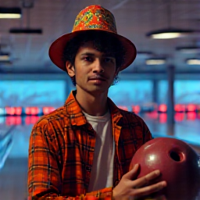
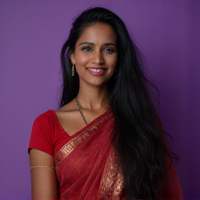
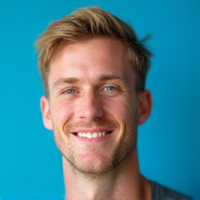
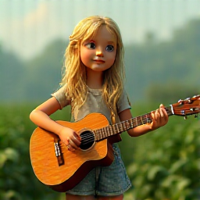
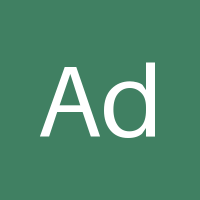