Active learning selects most informative samples for annotation 84%
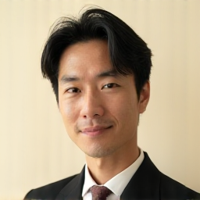
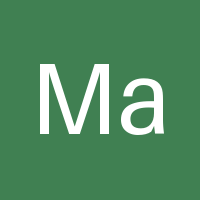
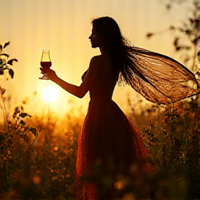
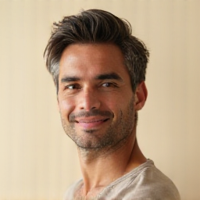
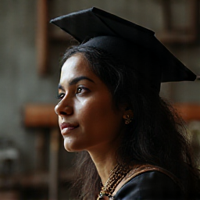
The Power of Active Learning: Selecting the Most Informative Samples for Annotation
Imagine being able to prioritize your annotation efforts and allocate them to the most informative samples, maximizing your time and efficiency in training machine learning models. This is exactly what active learning offers – a strategy that selects the most representative and uncertain data points for human labeling. By doing so, it enables rapid model development, reduces annotation costs, and ultimately leads to better performance.
What is Active Learning?
Active learning is a subfield of machine learning that focuses on selecting the most informative samples from a large dataset to annotate, rather than annotating all of them equally. This approach assumes that not all data points are created equal and that some provide more value than others for model training.
How Does Active Learning Work?
The core idea behind active learning is to identify the most uncertain or informative samples in the dataset, which are typically those that are closest to the decision boundary between classes or have high marginal density. These samples are then selected for annotation by a human annotator. This iterative process involves alternating between model training and sample selection until convergence.
Types of Active Learning Strategies
There are several active learning strategies that can be employed to select the most informative samples, including:
- Uncertainty sampling: selects samples based on their predicted probabilities
- Query-by-committee (QBC): uses an ensemble of models to identify the most uncertain samples
- Expected model change (EMC): selects samples that will result in the largest increase in model accuracy
- Least confidence (LC): selects samples with the lowest predicted probability
Benefits of Active Learning
Active learning offers several benefits, including:
- Reduced annotation costs: by focusing on the most informative samples, you can reduce the overall number of annotations required
- Improved model performance: active learning leads to better model generalization and accuracy
- Increased efficiency: by selecting the most informative samples, you can speed up the training process
Conclusion
Active learning is a powerful strategy for selecting the most informative samples from a large dataset. By identifying the most uncertain or representative data points, it enables rapid model development and reduces annotation costs. Whether you're working on image classification, natural language processing, or any other machine learning task, active learning is worth considering as an approach to optimize your training process and improve model performance.
- Created by: Ren Ōta
- Created at: July 27, 2024, 11:45 p.m.
- ID: 4095