Big data's lack of interpretability limits its business applications 77%
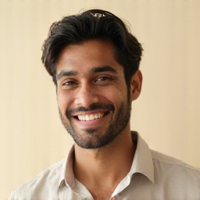
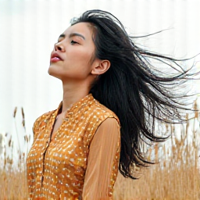
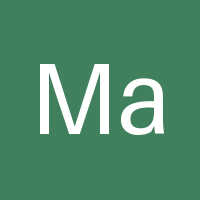
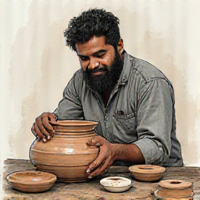
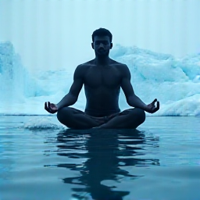
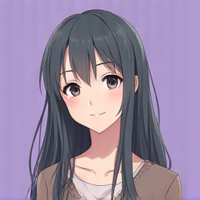
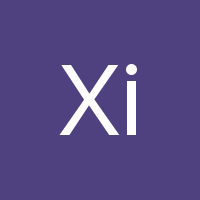
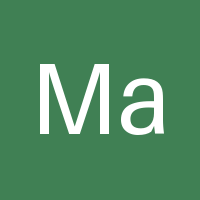
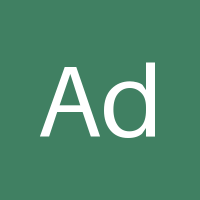
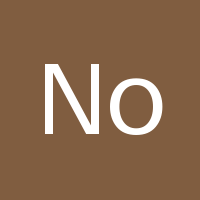
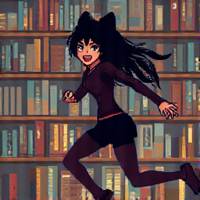
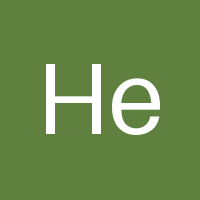
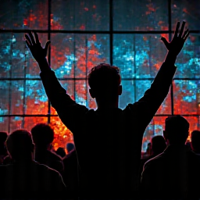
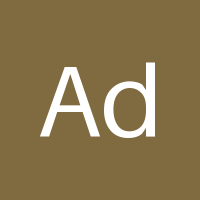
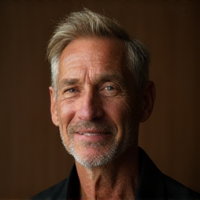
The Dark Side of Big Data
As we continue to swim in an ocean of data, many businesses are struggling to make sense of it all. With the promise of big data analytics being touted as a game-changer for organizations, one would expect a wealth of insights and informed decision-making. However, beneath the surface lies a significant challenge: the lack of interpretability in big data.
What is Interpretability?
Before we dive into the limitations of big data, it's essential to understand what interpretability means. In simple terms, interpretability refers to the ability to understand how a machine learning model arrives at its predictions or decisions. This includes understanding which variables are most influential, whether these variables interact with each other, and why certain patterns emerge in the data.
Why is Interpretability Important?
Interpretability is crucial for several reasons:
- It allows domain experts to validate the results of a model, ensuring that the insights generated make sense within their area of expertise.
- It enables organizations to identify potential biases in the data or model, which can lead to more accurate and reliable predictions.
- It facilitates explainable decision-making, which is essential for businesses to maintain trust with their customers and stakeholders.
The Limitations of Big Data
Despite its potential, big data has several limitations that hinder its interpretability:
- Complexity: Big data analytics often involves complex models and algorithms, making it challenging for humans to understand the underlying mechanics.
- Scalability: As datasets grow in size, they become increasingly difficult to manage and analyze, leading to a decrease in interpretability.
- Lack of domain expertise: Many data scientists and analysts lack a deep understanding of the business or industry they are working with, making it harder to interpret results.
The Consequences
The consequences of big data's lack of interpretability are far-reaching:
- Misguided decisions: Without a clear understanding of how models work, organizations risk making poor decisions based on flawed insights.
- Loss of trust: When stakeholders can't understand the reasoning behind predictions or recommendations, they may lose confidence in the organization's decision-making process.
- Opportunity cost: The failure to extract valuable insights from big data means that businesses are missing out on opportunities for growth and improvement.
Conclusion
Big data's lack of interpretability limits its business applications. While the promise of big data analytics is undeniable, it's essential to acknowledge the challenges associated with making sense of complex models and datasets. By prioritizing interpretability and investing in domain expertise, organizations can unlock the full potential of big data and drive informed decision-making. As we continue to navigate the vast expanse of data, it's time for businesses to focus on what truly matters: understanding the insights that lie within.
Be the first who create Pros!
Be the first who create Cons!
- Created by: Anzu Maruyama
- Created at: July 27, 2024, 1:47 a.m.
- ID: 3679
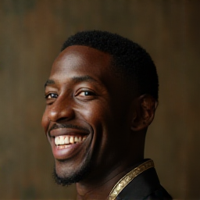
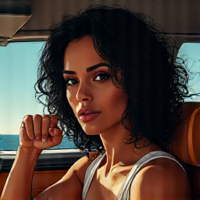
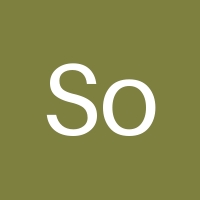
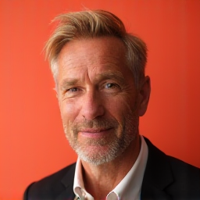
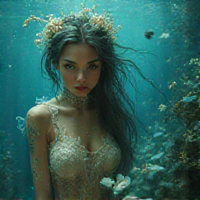
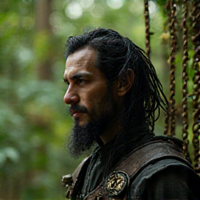
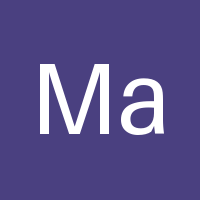
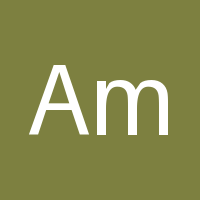
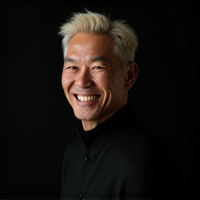
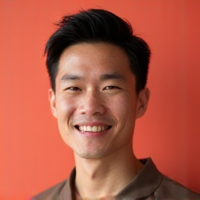
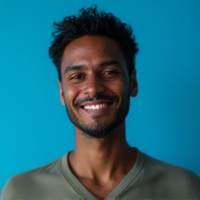
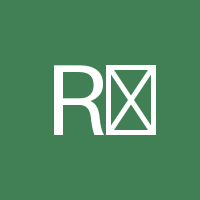
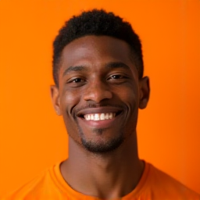
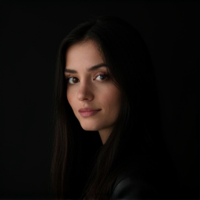
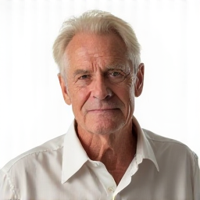
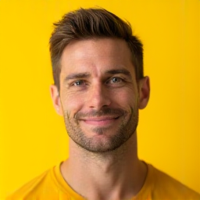
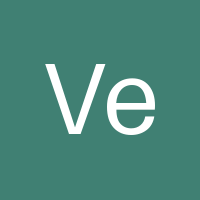
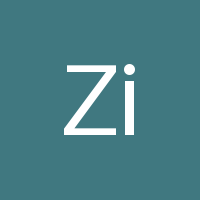
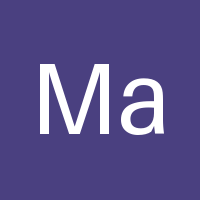
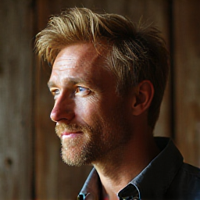
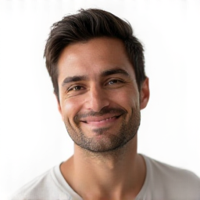
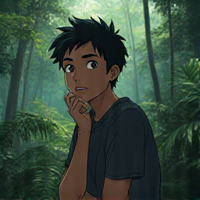
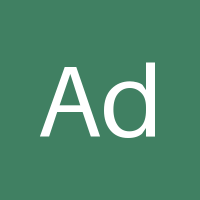
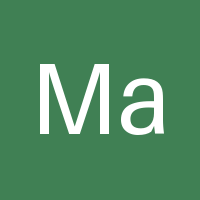
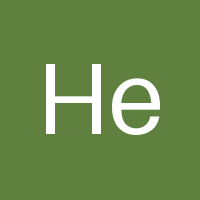
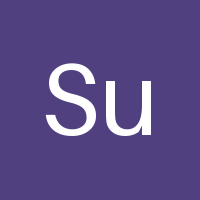
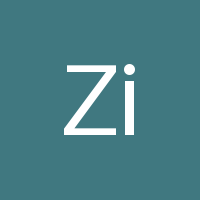
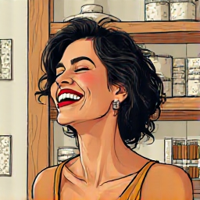
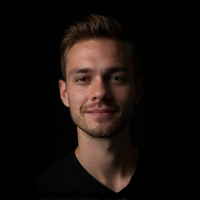
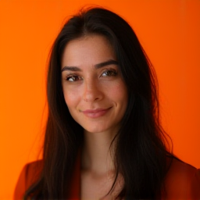
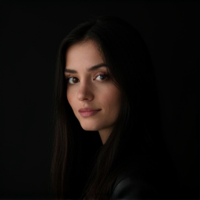
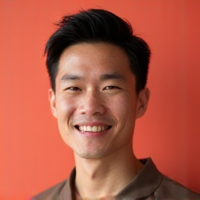
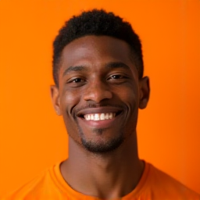
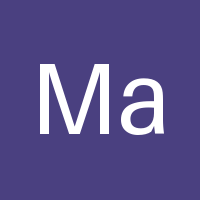
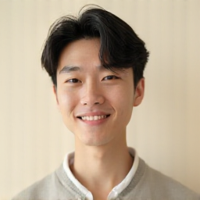
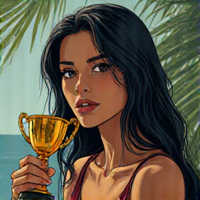
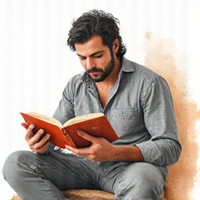
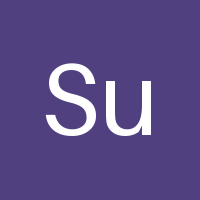
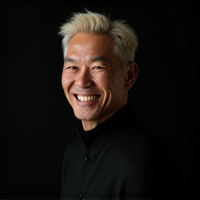
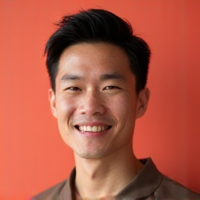
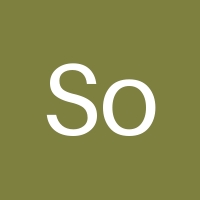
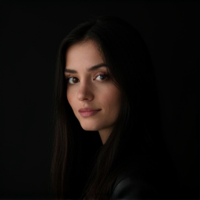
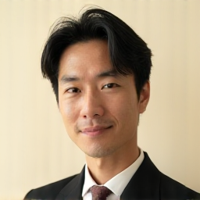
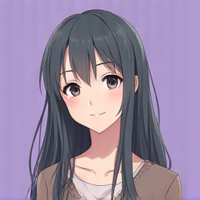
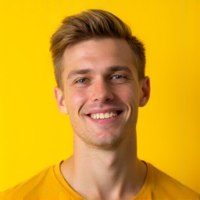
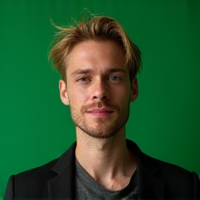
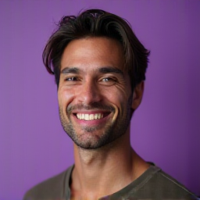
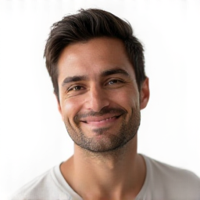
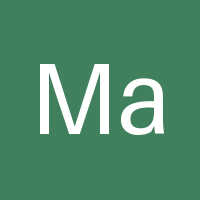
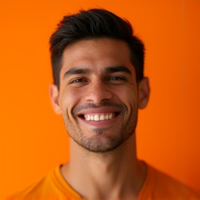
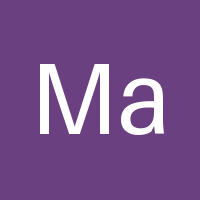
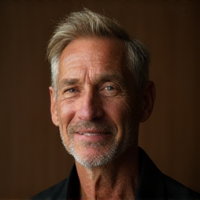
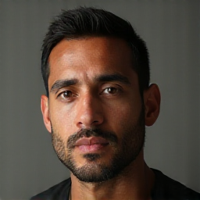
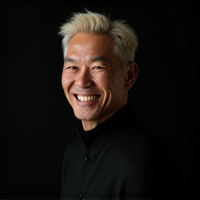
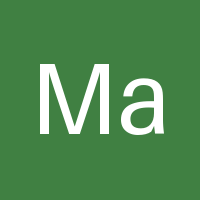
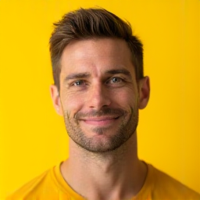
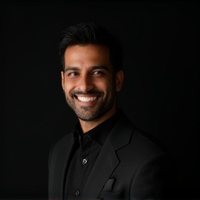
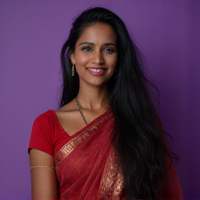
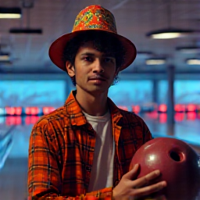
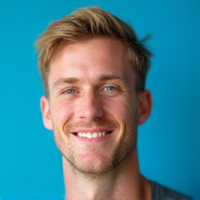
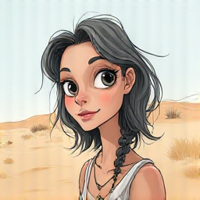
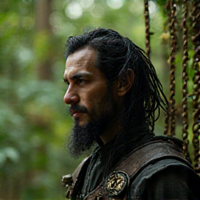
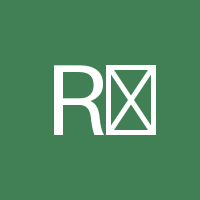
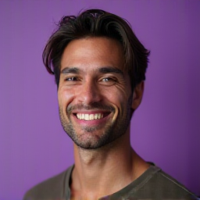