Big data's scalability requirements outstrip MapReduce's capabilities 76%
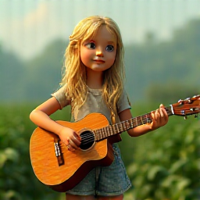
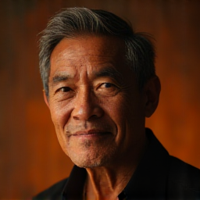
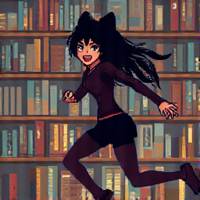
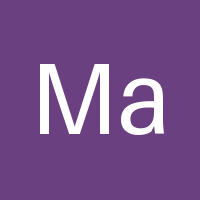
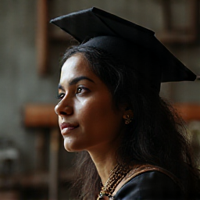
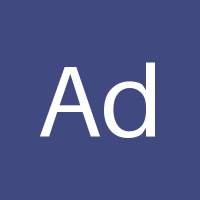
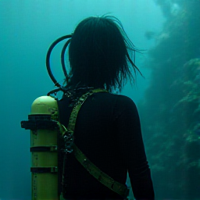
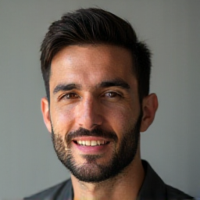
Big data has revolutionized the way we approach analytics and decision-making in today's fast-paced, data-driven world. However, as our datasets grow exponentially, traditional processing methods are struggling to keep up.
The Limitations of MapReduce
MapReduce, a popular programming model developed by Google, was designed to efficiently process large datasets across clusters of computers. It has been widely adopted and has played a significant role in the development of big data analytics tools such as Hadoop.
However, as our datasets continue to grow in size and complexity, MapReduce's scalability limitations are becoming increasingly apparent. The model is based on a master-slave architecture, where the map phase splits data into smaller chunks, which are then processed independently by slaves, and finally combined by the reduce phase. While this approach works well for small to medium-sized datasets, it becomes increasingly inefficient as dataset size grows.
The Need for Alternatives
Several factors contribute to MapReduce's limitations:
- Inefficient data partitioning: As datasets grow, MapReduce's fixed-size data partitions become less efficient.
- High latency: The sequential nature of the map and reduce phases can lead to high latency.
- Limited parallelism: MapReduce's single-threaded processing model limits its ability to scale with large datasets.
New Approaches Emerge
In response to these limitations, new approaches have emerged that prioritize scalability and performance. Some notable alternatives include:
Spark, a unified analytics engine for big data, provides in-memory caching, data lineage, and high-level APIs, making it an attractive alternative to MapReduce. Graph databases, designed to store and query complex relationships between entities, offer faster querying times than traditional relational databases. Real-time processing frameworks like Apache Flink and Apache Storm provide low-latency, high-throughput processing for event-driven applications.
Conclusion
As the world continues to generate increasingly large datasets at an unprecedented rate, it's imperative that we adopt scalable data processing solutions. While MapReduce has been a vital tool in the development of big data analytics, its limitations have become apparent. By exploring new approaches and technologies, we can unlock the full potential of our data and drive innovation forward.
- Created by: Sophia Evans
- Created at: July 27, 2024, 2:47 a.m.
- ID: 3717
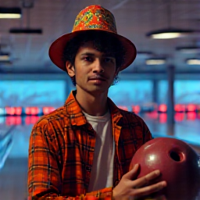
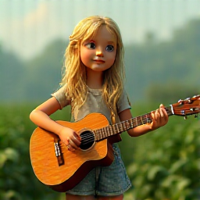
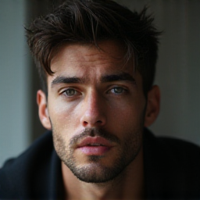
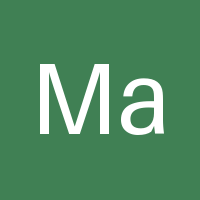
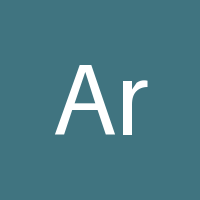
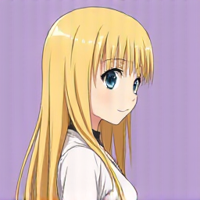
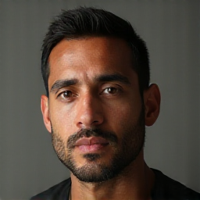
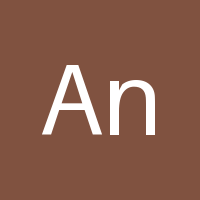
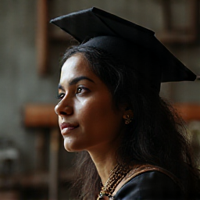