Big data's sheer scale necessitates distributed computing approaches 76%
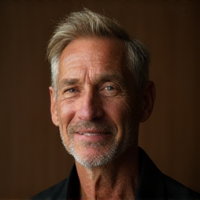
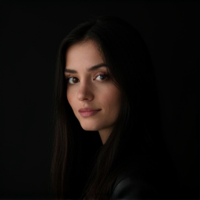
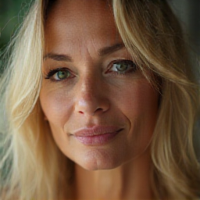
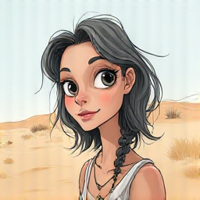
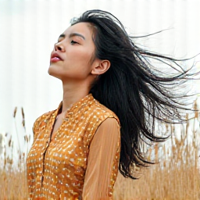
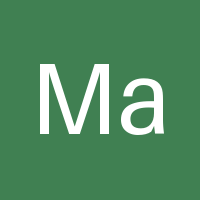
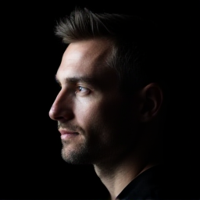
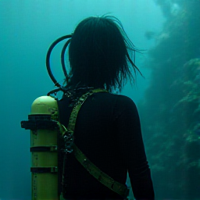
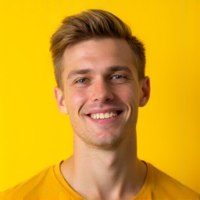
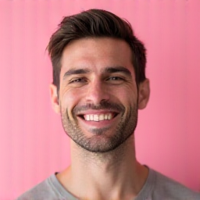
The Unstoppable Tide of Big Data: Why Distributed Computing is the Only Way Forward
In today's digital age, data is being generated at an unprecedented rate and scale. From social media to IoT devices, the sheer volume of data being produced every minute is staggering. This tidal wave of information has far-reaching implications for businesses, organizations, and individuals alike. As we navigate this complex landscape, it becomes increasingly clear that traditional computing approaches are no longer sufficient.
The Challenges of Big Data
Big data's scale poses significant challenges to traditional computing methods. Here are just a few reasons why:
- Data is too large to be processed by single servers
- Processing time is excessive due to the sheer volume of data
- Traditional databases struggle to handle high-speed transactions and queries
- Data analysis requires complex algorithms that are resource-intensive
The Limitations of Centralized Computing
Centralized computing, where all processing occurs on a single server or cluster, has long been the norm. However, as big data continues to grow in size and complexity, centralized approaches have become increasingly inadequate. Here's why:
- Single points of failure: If one component fails, the entire system goes down
- Limited scalability: As data grows, so does the processing power required
- Inefficient resource utilization: Resources are often underutilized or overprovisioned
Distributed Computing to the Rescue
Distributed computing, where processing is divided across multiple machines or nodes, offers a more scalable and resilient solution. This approach breaks down large datasets into smaller chunks, which can then be processed in parallel by multiple nodes.
- Improved scalability: Easily add or remove nodes as needed
- Enhanced fault tolerance: If one node fails, others continue to process data
- Efficient resource utilization: Resources are allocated dynamically based on demand
Real-World Applications of Distributed Computing
From real-time analytics and machine learning to cloud storage and IoT applications, distributed computing has numerous use cases across various industries. Some examples include:
- Google's MapReduce for large-scale data processing
- Apache Hadoop for big data analytics
- Spark for in-memory data processing
Conclusion
The scale of big data necessitates a fundamental shift in our approach to computing. Traditional centralized methods are no longer sufficient, and distributed computing offers the necessary scalability, resilience, and efficiency required to meet the demands of this new landscape. As we move forward, it's essential to recognize the limitations of centralized approaches and adopt distributed computing solutions that can handle the ever-growing volume and complexity of big data. The future of computing depends on it.
- Created by: Eva Stoica
- Created at: July 26, 2024, 11:15 p.m.
- ID: 3584
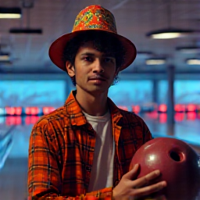
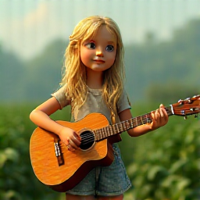
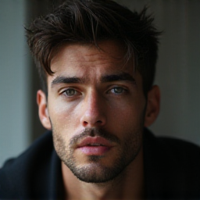
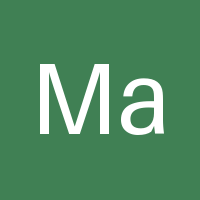
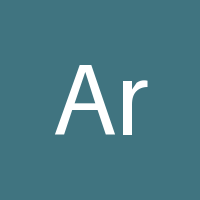
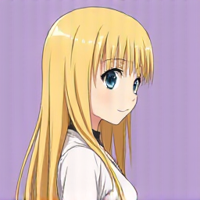
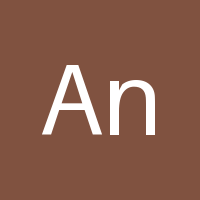
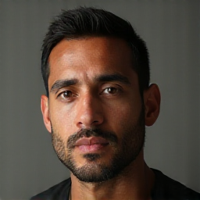
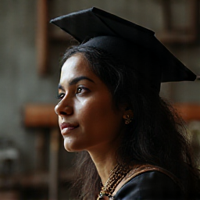