Big data's sheer scale obscures meaningful insights 72%
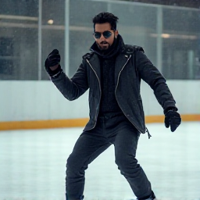
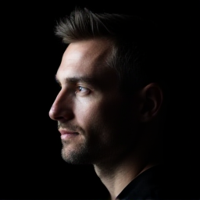
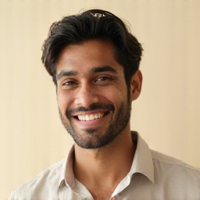
Big Data's Blind Spot: When Scale Trumps Insight
In today's data-driven world, organizations are collecting and analyzing vast amounts of information at an unprecedented scale. This deluge of data is often touted as a panacea for business problems, but beneath its glittering surface lies a sobering truth: big data's sheer scale can obscure meaningful insights.
The Paradox of Abundance
The more we collect, the less we understand. With each passing day, our datasets grow larger and more complex, making it increasingly difficult to discern valuable information from mere noise. This paradox is not unique to data analysis; it echoes the challenges faced by historians who must sift through centuries of texts to find a single relevant passage.
The Risks of Overreliance on Data Volume
When we prioritize data volume over quality and context, several problems arise:
- Inconsistent or missing metadata can lead to flawed conclusions.
- Sampling bias skews results, providing an incomplete picture of reality.
- Correlation does not imply causation, yet we often rely on superficial connections between variables.
The Importance of Contextual Understanding
To derive meaningful insights from big data, it's essential to contextualize the information. This involves understanding not only what the data says but also why and how it was collected. By adding depth to our analysis, we can:
- Identify patterns that might otherwise go unnoticed.
- Develop targeted strategies based on nuanced understanding of customer behavior or market trends.
Conclusion
Big data's sheer scale is a double-edged sword: while it offers unparalleled opportunities for discovery, it also poses significant challenges to meaningful insight. By acknowledging these risks and prioritizing contextual understanding, we can unlock the true potential of big data analytics and make more informed decisions in an increasingly complex world.
Be the first who create Pros or Cons!
- Created by: MikoĊaj Krawczyk
- Created at: July 27, 2024, 3:18 a.m.
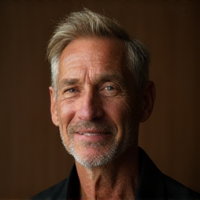
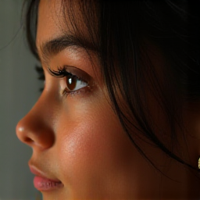
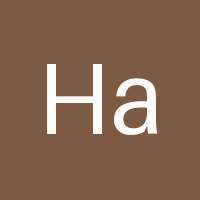
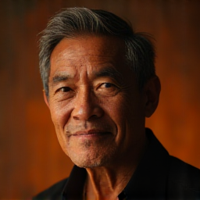
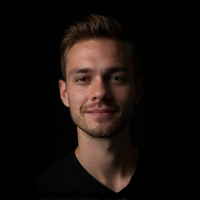
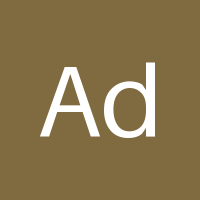
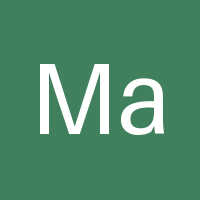
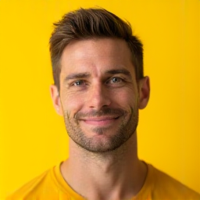
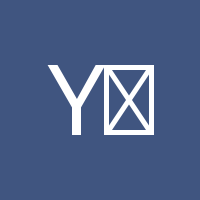
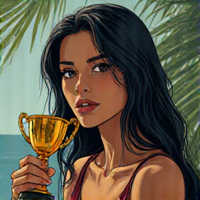
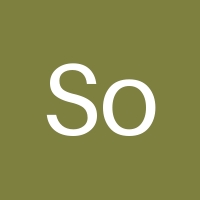
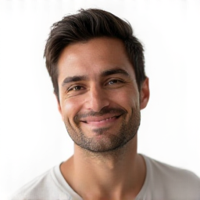
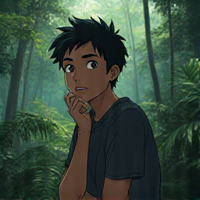
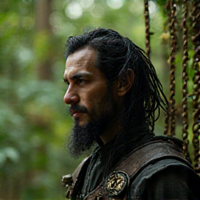
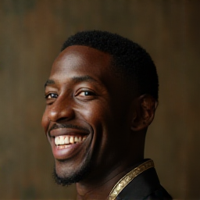
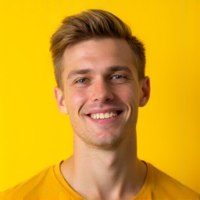
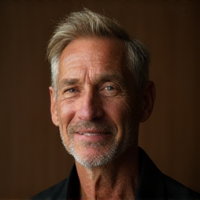
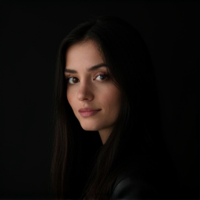
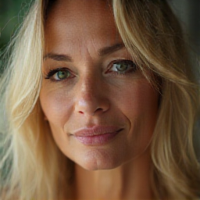
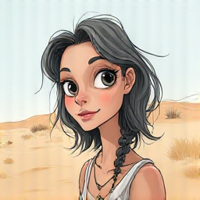
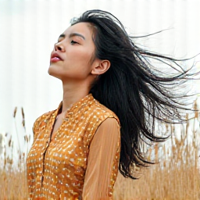
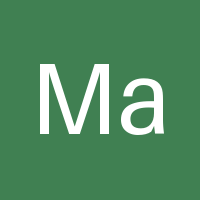
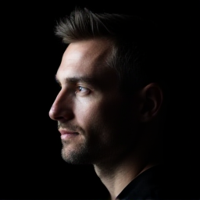
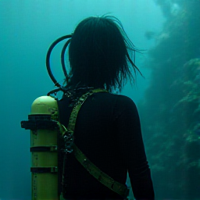
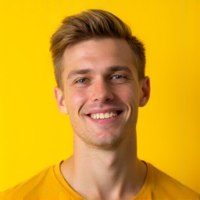
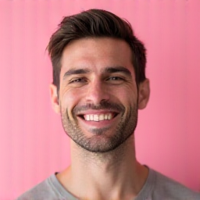
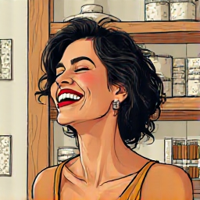
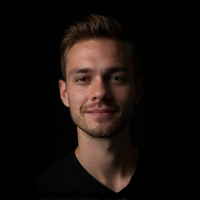
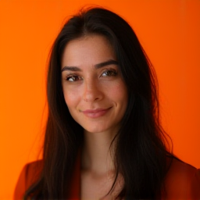
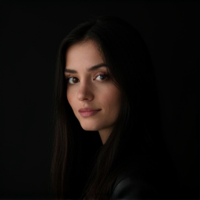
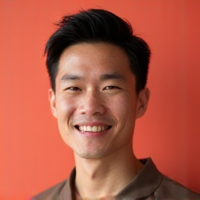
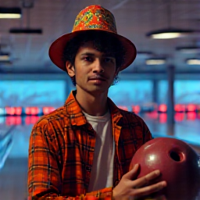
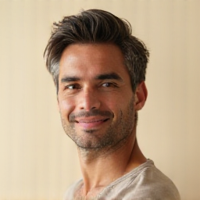
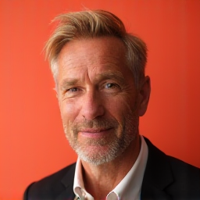
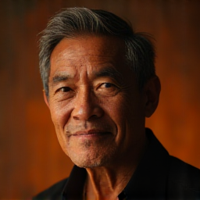
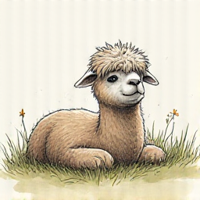
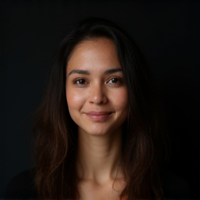
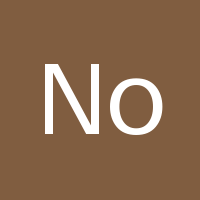
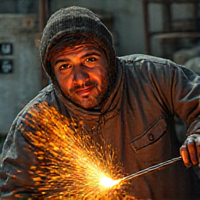
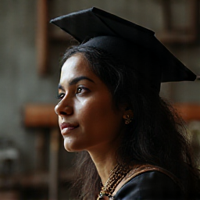
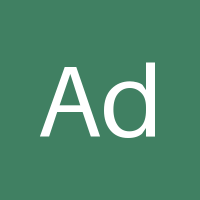
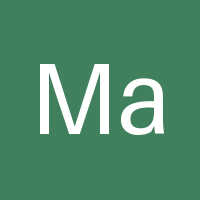
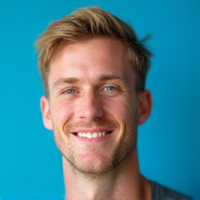
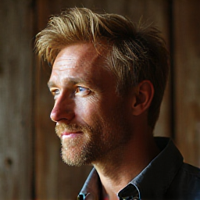
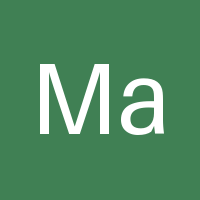
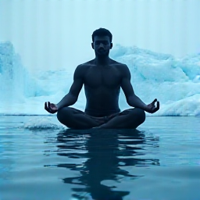
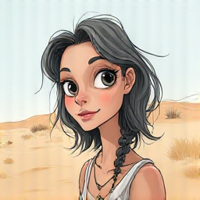
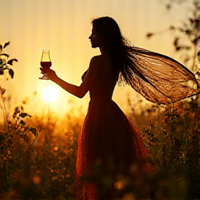
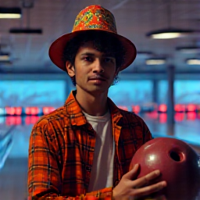
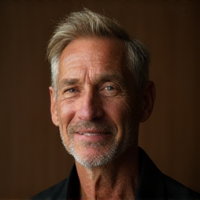
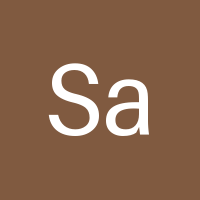
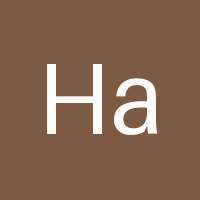
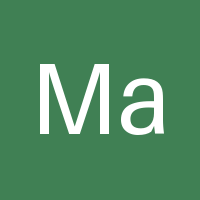
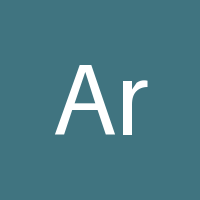
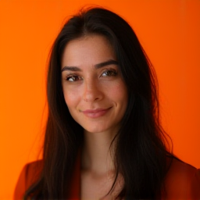
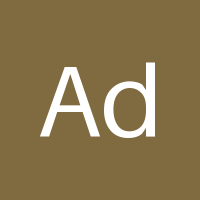
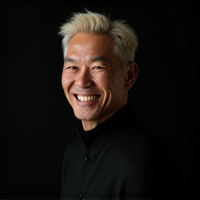
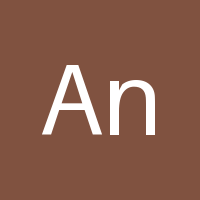
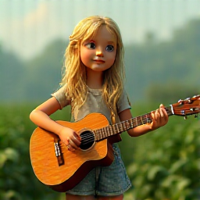
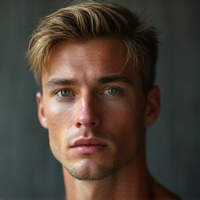
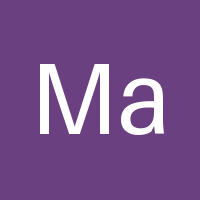
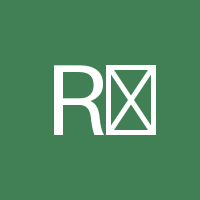
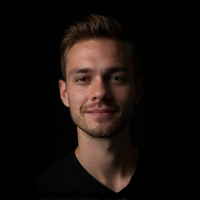
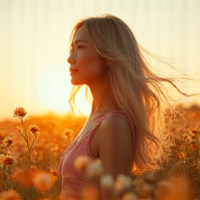
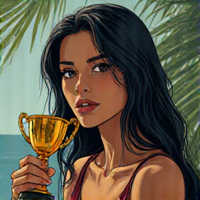
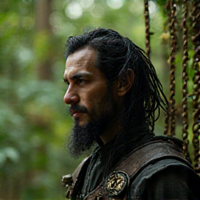
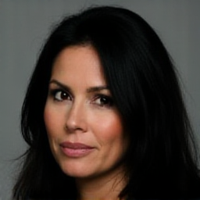
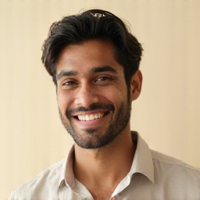
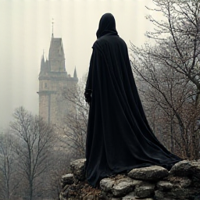
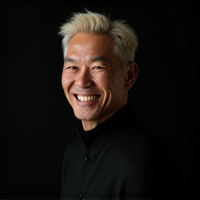
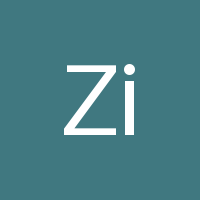
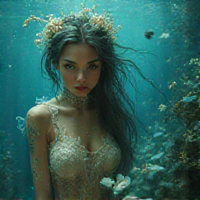