Big data's variability demands robust data quality control measures 95%
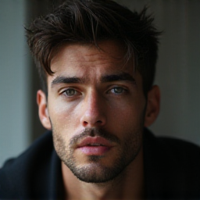
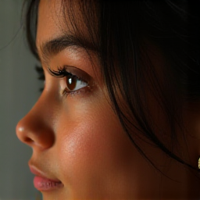
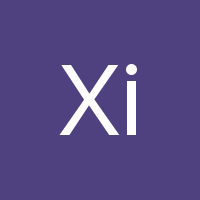
Big Data's Variability Demands Robust Data Quality Control Measures
In today's digital age, big data has become an integral part of decision-making processes in various industries. However, the sheer volume and variability of big data can make it challenging to maintain its quality. A single incorrect or missing piece of data can lead to inaccurate insights, poor business decisions, and ultimately, financial losses.
The Consequences of Poor Data Quality
Poor data quality can have severe consequences on businesses, including:
- Inaccurate market analysis
- Wrong product development
- Ineffective marketing strategies
- Loss of customer trust
- Financial losses due to incorrect decisions
Understanding Big Data's Variability
Big data is characterized by its high volume, velocity, and variety. The variability in big data refers to the different types of data sources, formats, and structures that are used to collect and store data. This variability can lead to inconsistent data quality, making it challenging to maintain accuracy and reliability.
Robust Data Quality Control Measures
To address the variability in big data, organizations need to implement robust data quality control measures. These measures should include:
- Regular data cleaning and validation
- Implementing data governance policies
- Using data profiling techniques
- Continuously monitoring data for errors and inconsistencies
Implementing a Data Quality Framework
A well-defined data quality framework is essential for ensuring the accuracy, reliability, and consistency of big data. This framework should include the following components:
Key Components of a Data Quality Framework
- A clear data governance strategy
- Regular data audits and assessments
- Continuous monitoring and reporting of data quality metrics
- Implementing corrective actions to address data quality issues
Conclusion
Big data's variability demands robust data quality control measures. Organizations must understand the consequences of poor data quality and implement a well-defined data quality framework to ensure accuracy, reliability, and consistency in their big data. By doing so, they can make informed decisions, improve business outcomes, and maintain customer trust.
- Created by: Ximena Moreno
- Created at: July 26, 2024, 11:20 p.m.
- ID: 3587