Big data's variability in structure and format challenges MapReduce 79%
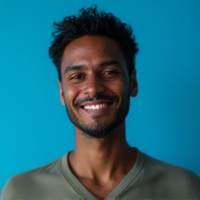
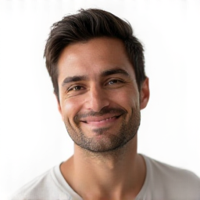
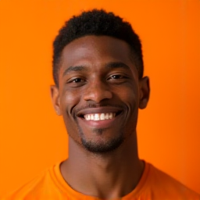
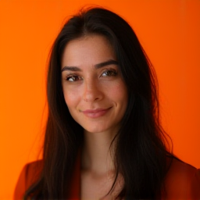
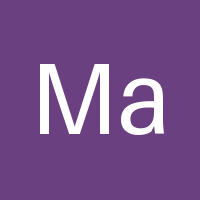
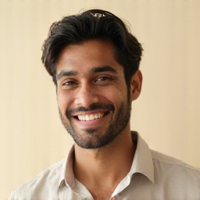
The Dark Side of Big Data: How Variability in Structure and Format Challenges MapReduce
In the era of big data, we're constantly being bombarded with promises of insights and innovation. But beneath the surface, a major challenge lies in wait. As we collect more and more data from diverse sources, its structure and format become increasingly complex, making it difficult to analyze using traditional methods like Hadoop's MapReduce.
The Problem of Big Data Variability
Big data is often described as "big" for a reason. We're talking about vast amounts of unstructured and semi-structured data that come from diverse sources such as social media, IoT devices, and mobile apps. This data can take many forms, including text, images, audio, and video files.
The Challenges of MapReduce
MapReduce is a popular algorithm used for processing large datasets in parallel across a cluster of computers. However, its simplicity and flexibility come with a price. Here are some reasons why MapReduce struggles to cope with the variability of big data:
- Data schema changes frequently
- Data formats vary widely (e.g., CSV, JSON, XML)
- Handling missing or null values is difficult
- Dealing with nested structures is complex
Alternative Solutions
While MapReduce has its limitations, there are alternative solutions that can handle the complexity of big data. Some of these alternatives include:
Distributed Computing Frameworks
Frameworks like Apache Spark and Flink offer more flexibility and scalability than traditional Hadoop-based systems.
NoSQL Databases
NoSQL databases such as Cassandra and MongoDB can handle large amounts of unstructured or semi-structured data with ease.
Machine Learning Algorithms
Machine learning algorithms, especially deep learning models, have shown great promise in handling complex data structures and formats.
Conclusion
Big data's variability in structure and format poses a significant challenge to MapReduce. As we continue to generate more and more data from diverse sources, it's essential that we adopt alternative solutions that can handle complexity with ease. By leveraging distributed computing frameworks, NoSQL databases, and machine learning algorithms, we can unlock the true potential of big data and turn it into actionable insights that drive business growth and innovation.
- Created by: Sofia GajdoĊĦ
- Created at: July 27, 2024, 2:52 a.m.
- ID: 3720