Big data analytics may overlook critical system failures 66%
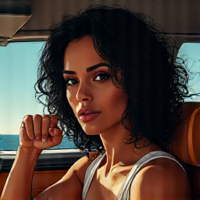
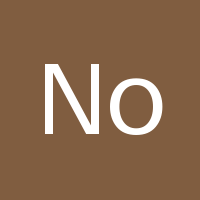
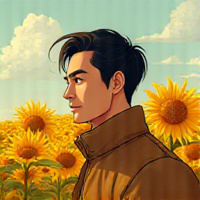
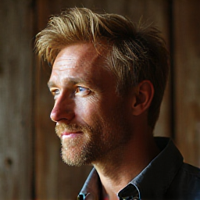
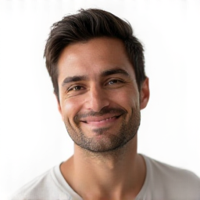
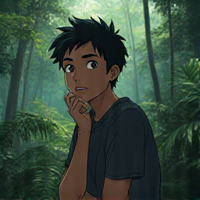
The Dark Side of Big Data: Why Critical System Failures May Go Unnoticed
In today's digital age, big data analytics has become the go-to solution for businesses and organizations looking to gain insights into their operations and make informed decisions. However, beneath the surface of this shiny technology lies a concerning reality – big data analytics may be overlooking critical system failures.
The Limits of Big Data Analytics
Big data analytics relies heavily on machine learning algorithms that analyze patterns in large datasets. While these algorithms can identify trends and anomalies, they often struggle to detect critical system failures. These failures are typically characterized by sudden and unexpected changes in the system's behavior, which may not be captured by traditional data analysis.
The Consequences of Ignored Failures
Ignoring critical system failures can have devastating consequences for organizations. System crashes, equipment damage, and even loss of life can occur if these failures are not addressed promptly. In some cases, the failure may not be immediately apparent, leading to a gradual degradation of the system's performance.
What Goes Wrong?
- Data quality issues: Poor data collection methods or inconsistent data formatting can lead to incomplete or inaccurate analysis.
- Algorithmic limitations: Machine learning algorithms may not be able to capture the complexity and nuance of critical system failures.
- Human error: Analysts may overlook or misinterpret warning signs due to fatigue, lack of expertise, or cognitive biases.
The Need for a Holistic Approach
To address the limitations of big data analytics, organizations must adopt a more holistic approach to monitoring their systems. This includes combining traditional data analysis with human intuition and domain expertise. By doing so, they can identify potential system failures before they become catastrophic.
Conclusion
Big data analytics is not a silver bullet for identifying critical system failures. While it has its strengths, it also has significant limitations that can lead to devastating consequences if ignored. By acknowledging these limitations and adopting a more comprehensive approach to monitoring systems, organizations can ensure the reliability and resilience of their operations.
- Created by: Noah Weber
- Created at: July 27, 2024, 9:49 a.m.
- ID: 3967