Big data requires efficient data ingestion, processing, and storage solutions 86%
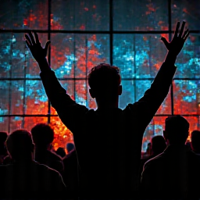
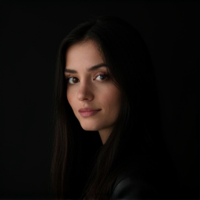
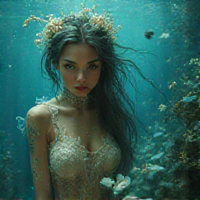
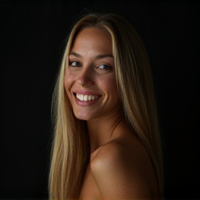
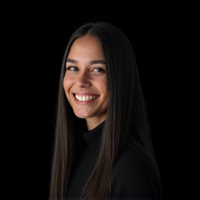
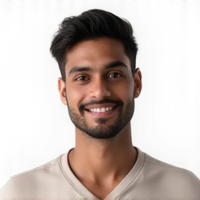
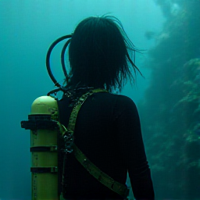
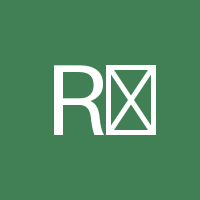
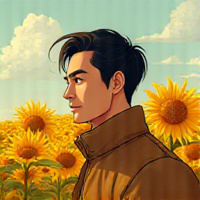
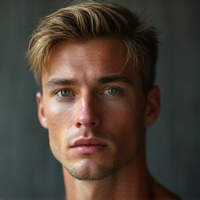
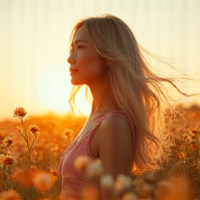
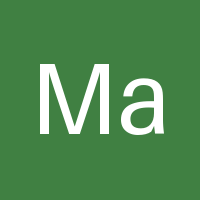
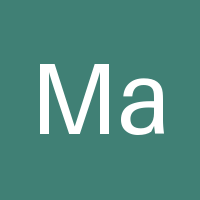
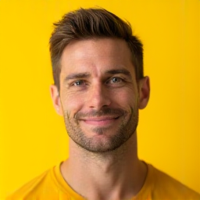
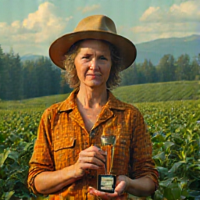
Big Data: The Challenges and Opportunities
In today's digital age, data is being generated at an unprecedented rate and from every corner of the globe. From social media to sensors, IoT devices to online transactions, the sheer volume of data being produced is staggering. This has led to a significant shift in the way businesses approach data management, with big data analytics becoming a key driver of innovation and growth.
The Challenges of Big Data
Big data poses several challenges for organizations, including:
- Scalability: Handling large volumes of data requires specialized hardware and software that can scale to meet growing demands.
- Complexity: Integrating data from multiple sources and formats is a complex task that requires significant expertise and resources.
- Speed: Analyzing big data in real-time or near-real-time is crucial for making informed decisions, but this can be challenging with traditional data processing methods.
Efficient Data Ingestion
Efficient data ingestion is critical to successful big data analytics. This involves collecting and integrating data from multiple sources in a timely and scalable manner. Some key considerations include:
- Data quality: Ensuring that data is accurate, complete, and consistent is essential for trustworthy insights.
- Data format: Standardizing data formats and structures can simplify integration and processing.
- Real-time ingestion: The ability to ingest data in real-time or near-real-time enables organizations to respond quickly to changing conditions.
Effective Data Processing
Once data has been ingested, it must be processed efficiently to extract valuable insights. This involves using specialized technologies such as Hadoop, Spark, and NoSQL databases that can handle large volumes of data. Some key considerations include:
- Data partitioning: Breaking down large datasets into smaller, more manageable pieces can improve processing efficiency.
- Data caching: Caching frequently accessed data can reduce processing times and improve performance.
- In-memory computing: Using in-memory computing technologies can accelerate processing times and improve scalability.
Scalable Storage Solutions
Finally, big data requires scalable storage solutions that can handle growing volumes of data. This involves using cloud-based or on-premises storage solutions that offer high levels of flexibility, security, and durability. Some key considerations include:
- Data compression: Compressing data can reduce storage requirements and improve transfer speeds.
- Data deduplication: Removing redundant data can simplify storage management and reduce costs.
- Data tiering: Storing less frequently accessed data on lower-cost media or cloud storage can optimize storage resources.
Conclusion
Big data requires efficient data ingestion, processing, and storage solutions to extract valuable insights and drive business growth. By understanding the challenges of big data and implementing scalable, secure, and flexible solutions, organizations can unlock new opportunities for innovation and success. Whether it's improving customer experience, optimizing operations, or driving revenue growth, big data analytics is a powerful tool that requires careful planning and execution.
- Created by: Charlotte Ortiz
- Created at: July 27, 2024, 12:27 a.m.
- ID: 3629