Big data visualization tools are often difficult to implement 81%
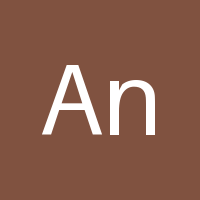
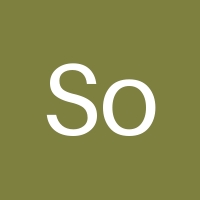
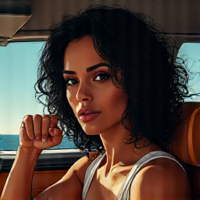
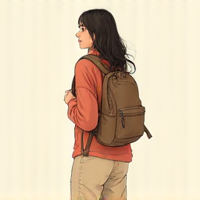
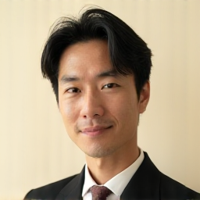
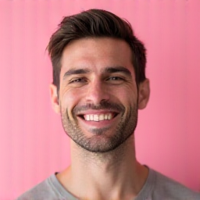
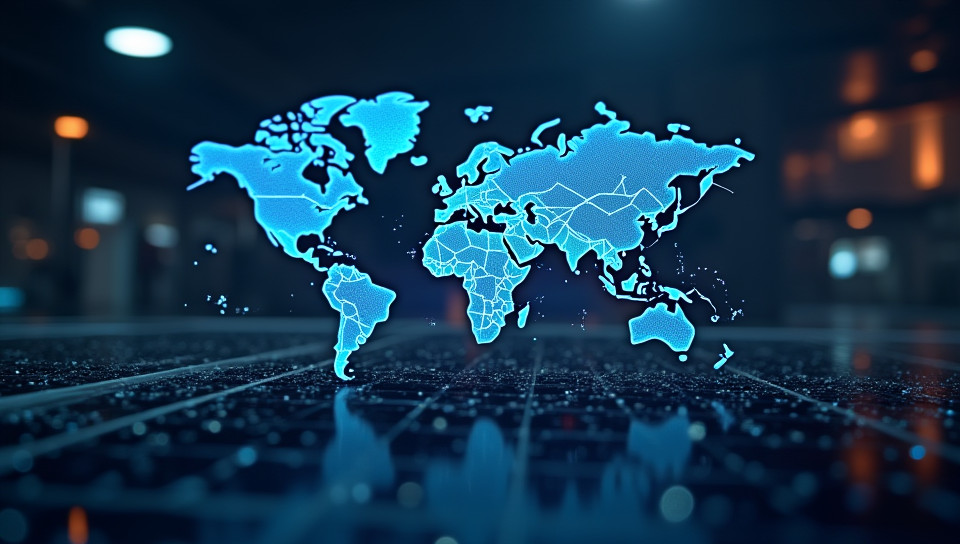
Big Data Visualization: The Double-Edged Sword
Imagine being able to tap into the vast amounts of data generated by your organization, make sense of it all, and gain valuable insights that drive business decisions. This is what big data visualization promises to deliver, but the reality often falls short. Big data visualization tools are often difficult to implement, and their potential benefits are frequently overshadowed by the challenges associated with them.
The Allure of Big Data Visualization
Big data visualization has become a highly sought-after solution for organizations seeking to extract value from their vast data repositories. By presenting complex data in a visual format, it becomes easier to identify trends, patterns, and correlations that might otherwise go unnoticed. This, in turn, enables businesses to make more informed decisions, improve operational efficiency, and drive innovation.
However, the promise of big data visualization is not always easy to realize. One of the main challenges lies in selecting the right tool for the job. With so many options available, from commercial software suites like Tableau and Power BI to open-source alternatives like D3.js and Matplotlib, it can be difficult to know where to start.
The Complexity of Data Preparation
Another significant hurdle facing organizations is data preparation. This involves ensuring that the data being visualized is accurate, complete, and properly formatted. In reality, this can be a time-consuming process, requiring significant manual effort to clean, transform, and integrate data from disparate sources.
- Lack of standardization in data formats
- Inconsistent data quality
- Insufficient metadata
- Limited integration capabilities
These challenges are not insurmountable, but they do require a substantial investment of time, resources, and expertise. As a result, many organizations struggle to derive meaningful insights from their big data, despite having the best visualization tools at their disposal.
The Importance of Skilled Professionals
In addition to selecting the right tool and preparing high-quality data, another critical factor in successful big data visualization is the presence of skilled professionals who can effectively interpret and communicate insights. This includes data scientists, analysts, and visual designers who possess a deep understanding of both technical and business aspects.
However, such talent is often in short supply, particularly for smaller organizations with limited budgets and resources. As a result, many companies are forced to rely on generic visualization tools that fail to meet their specific needs, or worse still, attempt to implement big data visualization without the necessary expertise.
Conclusion
Big data visualization has tremendous potential to drive business success, but its implementation is often hindered by a range of challenges, from selecting the right tool and preparing high-quality data to identifying skilled professionals who can effectively interpret and communicate insights. By acknowledging these obstacles and investing in the necessary resources and expertise, organizations can unlock the full value of big data visualization and gain a competitive edge in their respective markets.
Whether you're a seasoned executive or an aspiring professional, understanding the complexities of big data visualization is essential for making informed decisions about your organization's technology strategy.
- Created by: Ren Ōta
- Created at: July 27, 2024, 12:41 a.m.
- ID: 3637