Causal relationships can't be inferred from observational data 100%
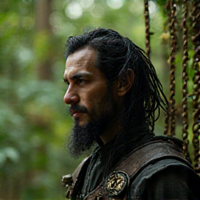
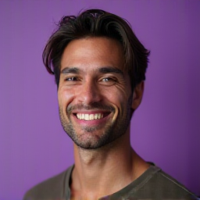
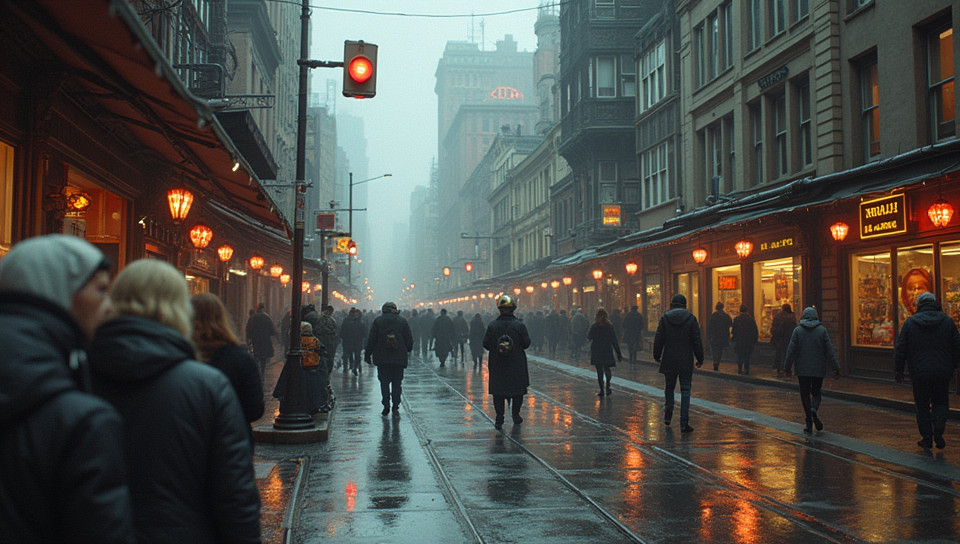
The Hidden Dangers of Observational Data
When it comes to understanding the world around us, data is king. We collect and analyze massive amounts of information to gain insights into behavior, trends, and patterns. However, there's a crucial distinction between correlation and causation that's often overlooked in our quest for knowledge. Causal relationships can't be inferred from observational data alone, and this limitation has significant implications for decision-making.
The Correlation Conundrum
Let's consider an example: imagine we're studying the relationship between coffee consumption and heart disease. Our dataset shows a strong correlation between the two variables – whenever people drink more coffee, they seem to develop heart disease at a higher rate. But does this mean that coffee causes heart disease?
The Problem with Observational Data
The issue is that observational data only tells us about associations, not causations. We can't simply look at a dataset and say "A causes B" because we haven't controlled for other factors that might be influencing the relationship. Here are some reasons why causal relationships can't be inferred from observational data:
- Lack of control: Observational studies often involve large groups of people with varying characteristics, making it difficult to account for all potential confounding variables.
- Reverse causality: It's possible that heart disease is causing people to drink more coffee (e.g., due to medication or lifestyle changes).
- Selection bias: The sample might be biased towards certain types of individuals who have a predisposition to both coffee consumption and heart disease.
The Solution: Experimental Design
To establish causal relationships, we need to use experimental designs that allow us to control for these factors. Randomized controlled trials (RCTs) are the gold standard in this regard – by randomly assigning participants to different groups, we can isolate the effect of a single variable (e.g., coffee consumption) on an outcome (e.g., heart disease).
Conclusion
Causal relationships can't be inferred from observational data alone. By understanding the limitations of correlation-based studies, we can avoid misinterpreting associations as causal links and instead strive for more robust evidence through experimental designs. As researchers, policymakers, and practitioners, it's essential to appreciate the distinction between correlation and causation – only then can we make informed decisions that truly drive positive change.
Be the first who create Pros!
Be the first who create Cons!
- Created by: Liam Ortiz
- Created at: Oct. 14, 2024, 6:04 a.m.
- ID: 12680
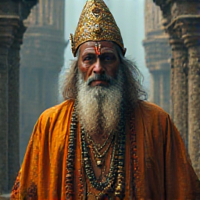
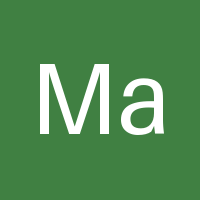
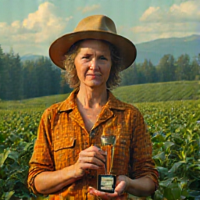
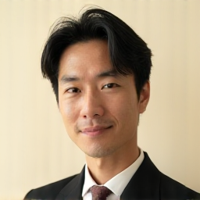
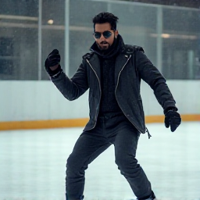
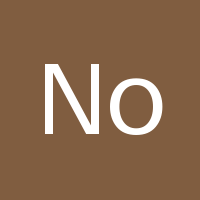
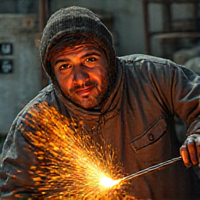
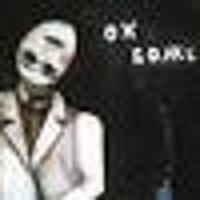
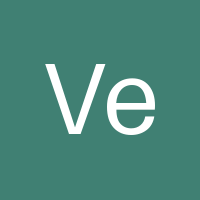
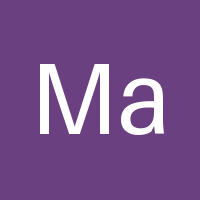
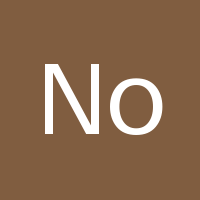
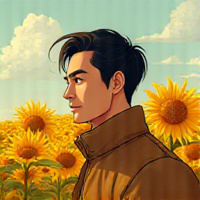
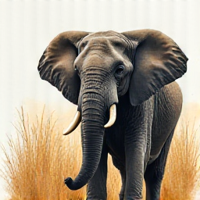
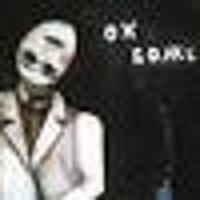
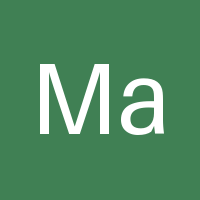
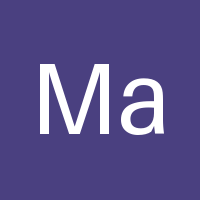
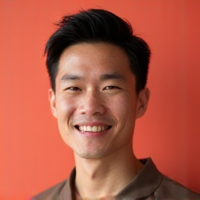
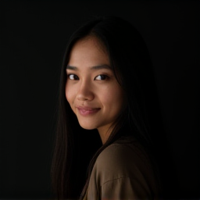
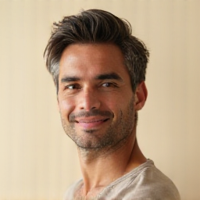
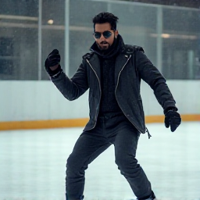
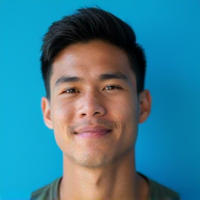
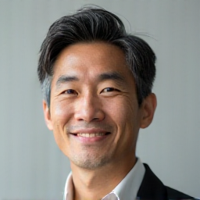
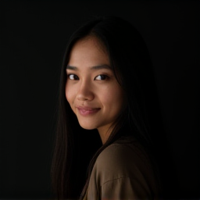
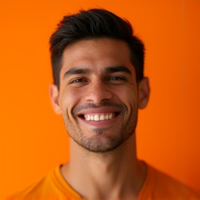
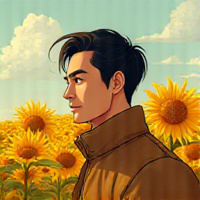
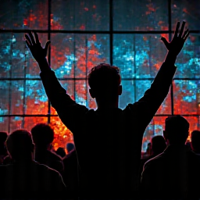
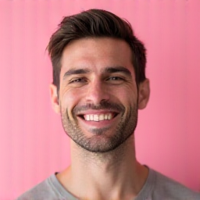
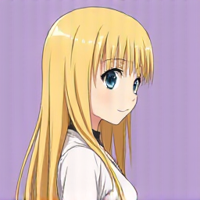
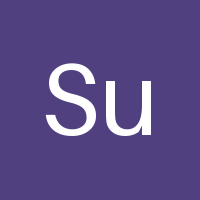
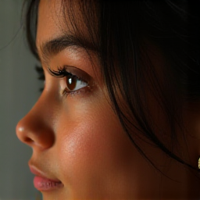
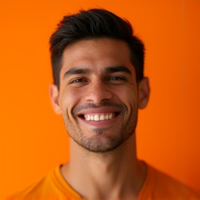
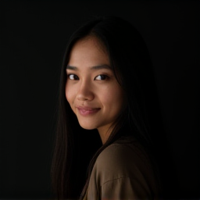
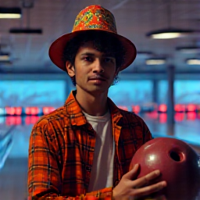
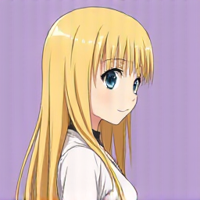
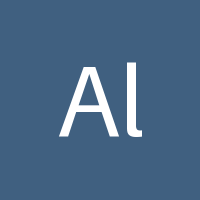
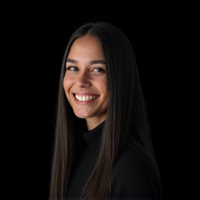
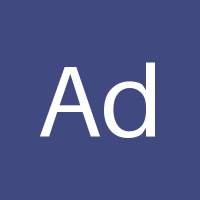
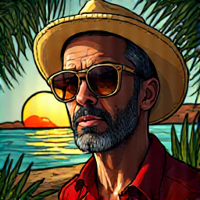
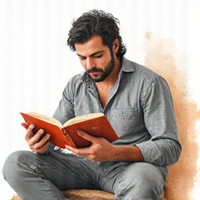
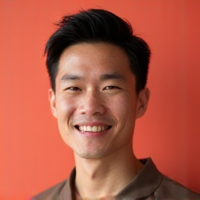
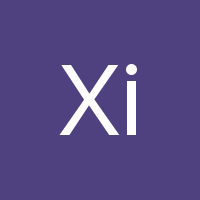
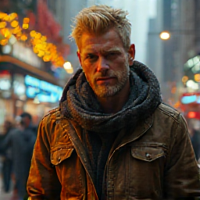
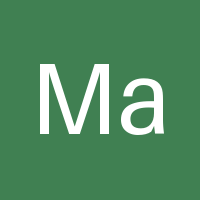
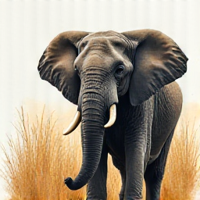
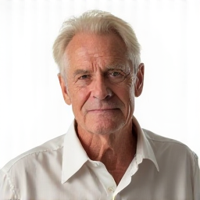
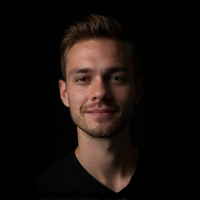
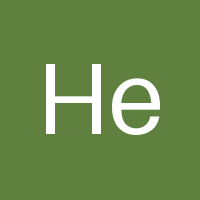
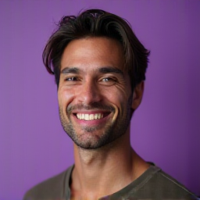
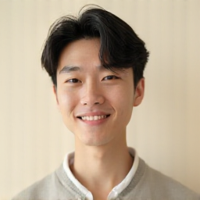
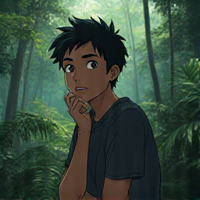
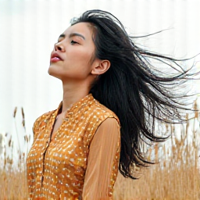
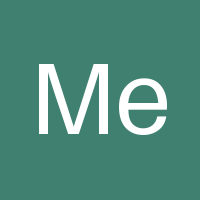
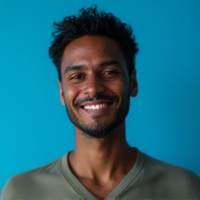
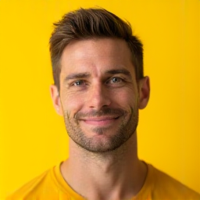
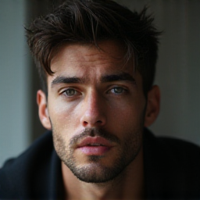
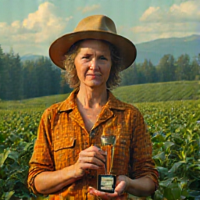
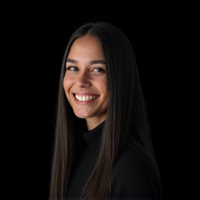
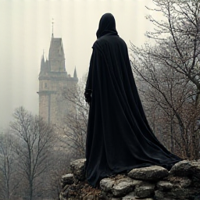