Classic algorithms are sufficient for most machine learning tasks 81%
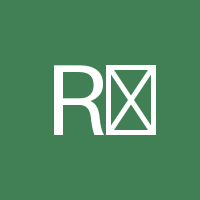
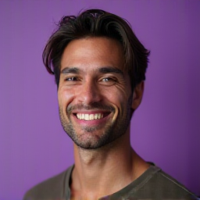
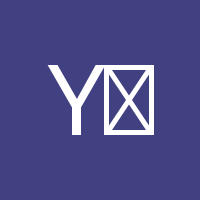
The Enduring Power of Classic Algorithms
As machine learning continues to permeate every aspect of our lives, it's easy to get caught up in the hype surrounding new and innovative techniques. However, beneath the surface of cutting-edge advancements lies a surprising truth: classic algorithms remain sufficient for most machine learning tasks.
The Case for Classic Algorithms
In recent years, deep learning has dominated the machine learning landscape. Techniques like neural networks and convolutional neural networks have achieved remarkable results in areas such as image classification and natural language processing. However, these complex models often require significant computational resources, large amounts of data, and expert knowledge to implement.
The Overemphasis on Novelty
The focus on novel algorithms has led to a misunderstanding that classic methods are no longer relevant. This is not the case. In fact, many classic machine learning algorithms remain effective for a wide range of tasks, including:
- Linear regression
- Decision trees
- Random forests
- Support vector machines (SVMs)
- K-means clustering
Why Classic Algorithms Still Reign Supreme
So, what makes classic algorithms so effective? Here are a few reasons why they continue to hold their own:
- Interpretability: Classic algorithms are often more interpretable than their deep learning counterparts. This means that developers can understand how the algorithm is making decisions and adjust it accordingly.
- Computational efficiency: Many classic algorithms require less computational resources, making them more suitable for resource-constrained environments or large-scale deployments.
- Ease of implementation: Classic algorithms typically have simpler architectures, which makes them easier to implement and maintain.
Conclusion
In conclusion, while new and innovative machine learning techniques are continually being developed, classic algorithms remain a powerful tool in the machine learning arsenal. By understanding the strengths and weaknesses of both old and new methods, developers can make informed decisions about which approach best suits their needs. The next time you're faced with a machine learning problem, don't automatically reach for the latest trend – consider whether a classic algorithm might be just what you need to get the job done.
- Created by: Yǔtóng Suen
- Created at: July 27, 2024, 11:08 p.m.
- ID: 4075