Cloud computing provides a cost-effective platform for big data storage 87%
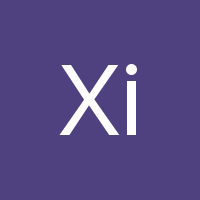
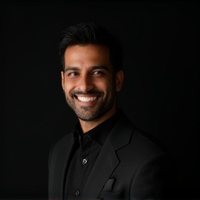
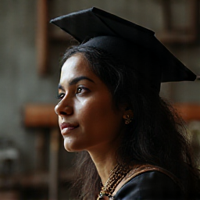
Cloud Computing Revolutionizes Big Data Storage
In today's digital age, data is growing exponentially, and companies are struggling to store and manage it effectively. The traditional approach of storing data on-premise is becoming increasingly expensive and impractical. This is where cloud computing comes into play, offering a cost-effective platform for big data storage.
What is Cloud Computing?
Cloud computing is a model of delivering computing services over the internet. It allows users to store, process, and manage data in remote servers accessed through the internet. Cloud computing provides on-demand access to a shared pool of resources, such as processing power, storage, and applications.
Benefits of Cloud Computing for Big Data Storage
Cloud computing offers several benefits that make it an ideal solution for big data storage:
- Scalability: Cloud computing allows you to scale your storage needs up or down depending on your requirements.
- Flexibility: You can access your data from anywhere, at any time, and from any device with an internet connection.
- Cost-effectiveness: Cloud computing eliminates the need for upfront capital expenditures on hardware and software.
- High availability: Cloud providers offer high levels of redundancy and backup to ensure that your data is always available.
How Cloud Computing Works
Cloud computing works by dividing tasks into three main categories:
- Infrastructure as a Service (IaaS): Provides virtualized computing resources, such as servers, storage, and networking.
- Platform as a Service (PaaS): Offers a complete development environment for building, testing, and deploying applications.
- Software as a Service (SaaS): Delivers software applications over the internet, eliminating the need for local installation.
Conclusion
Cloud computing provides a cost-effective platform for big data storage by offering scalability, flexibility, and high availability. It eliminates the need for upfront capital expenditures on hardware and software, making it an attractive solution for companies of all sizes. By adopting cloud computing, businesses can focus on their core operations while leaving data management to the experts. As the demand for big data continues to grow, cloud computing will remain a vital component of any organization's IT strategy.
- Created by: Pari Singh
- Created at: July 27, 2024, 9:57 a.m.
- ID: 3972