Complexities in handling high-dimensional datasets 90%
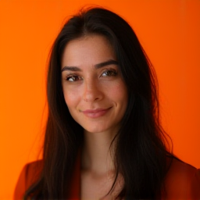
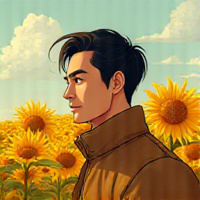
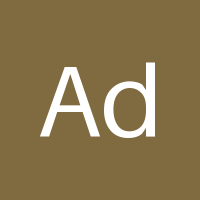
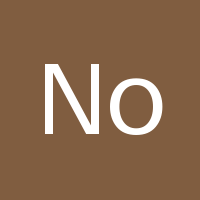
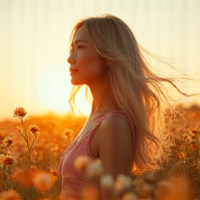
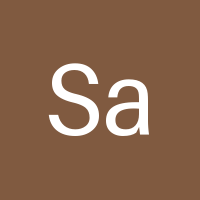
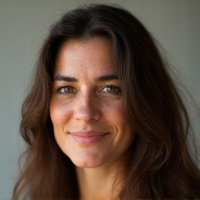
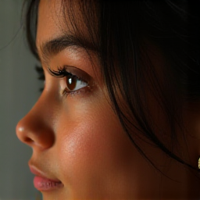
Handling High-Dimensional Datasets: The Hidden Challenges
In today's data-driven world, we are constantly being bombarded with an influx of complex datasets that have multiple variables and dimensions. These high-dimensional datasets can provide valuable insights into various fields such as healthcare, finance, and social sciences. However, they also present a significant challenge to data scientists and analysts who need to make sense of them.
The Challenges of High-Dimensional Data
High-dimensional data is characterized by its many features or variables. While having more data may seem like a good thing, it can actually lead to a range of challenges that make it difficult to analyze and understand. Some of the key issues associated with high-dimensional data include:
- Increasing computational complexity
- Difficulty in identifying patterns and relationships between variables
- Overfitting and underfitting models due to the curse of dimensionality
Understanding the Curse of Dimensionality
The curse of dimensionality is a phenomenon that occurs when we try to analyze high-dimensional data. As the number of dimensions increases, the distance between data points also increases, making it more difficult to identify patterns and relationships. This can lead to models that are overly complex or inaccurate.
Data Reduction Techniques
To overcome some of these challenges, data reduction techniques such as feature selection and dimensionality reduction can be used. Feature selection involves selecting a subset of the most relevant features from the original dataset, while dimensionality reduction involves transforming the high-dimensional data into a lower-dimensional space.
Conclusions
Handling high-dimensional datasets is a complex task that requires careful consideration of various challenges and limitations. By understanding these challenges and using appropriate techniques such as data reduction, we can unlock valuable insights from our data and make more informed decisions. As data scientists and analysts, it is essential to be aware of the complexities associated with high-dimensional data and to use effective strategies to overcome them.
- Created by: Adriana Gonçalves
- Created at: July 26, 2024, 11:59 p.m.
- ID: 3611