Correlation does not imply a causal link 91%
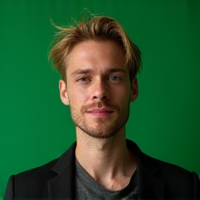
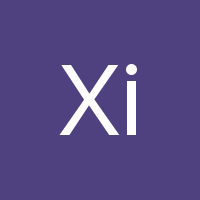
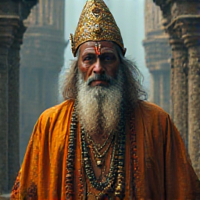
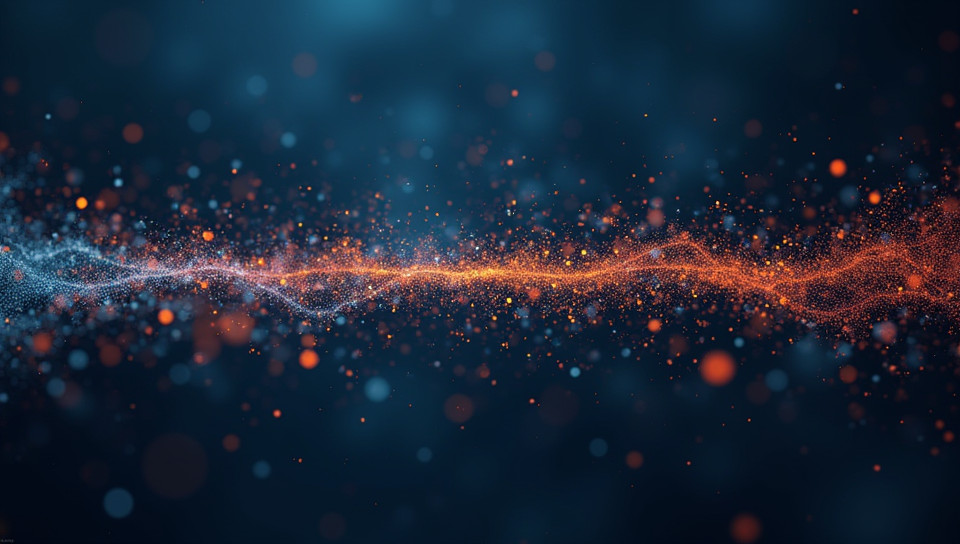
Correlation Does Not Imply Causal Link: A Critical Understanding for Data Analysis
As data analysts, we often rely on statistical methods to identify patterns and relationships between variables. However, there's a crucial distinction that can easily be overlooked in our enthusiasm to uncover insights: correlation does not imply causation.
What is Correlation?
Correlation measures the degree of relationship between two or more variables. It quantifies how much one variable changes when another changes. While correlation is useful for identifying potential relationships, it doesn't provide information about the underlying mechanisms driving these associations. In other words, correlation shows us that something might be related to something else, but it doesn't tell us why.
The Problem with Causal Inference
Many people mistakenly assume that if two variables are highly correlated, one must cause the other. This is a flawed assumption because correlation does not necessarily imply causation. For instance:
- A study found that there's a strong positive correlation between the number of ice cream cones sold and the number of drowning deaths.
- Another study discovered a significant negative correlation between the amount of coffee consumed and life expectancy.
At first glance, it might seem reasonable to conclude that eating ice cream causes drowning or drinking coffee increases lifespan. However, this is not necessarily true. In reality, both variables are likely related to other underlying factors.
Confounding Variables
Confounding variables are external factors that influence the relationship between two or more variables. They can distort our understanding of causality and lead us to incorrect conclusions. For example:
- In the ice cream drowning study, a confounding variable might be temperature: when it's hot outside, people tend to buy more ice cream, which increases drowning deaths because they're swimming in lakes and rivers.
- In the coffee study, a confounding variable could be socioeconomic status: people who drink more coffee are likely wealthier and have access to better healthcare, leading to higher life expectancy.
How to Avoid This Mistake
So how can we avoid mistakenly assuming causality from correlation? Here are some best practices:
- Look for underlying mechanisms that might explain the relationship.
- Consider alternative explanations, such as confounding variables or reverse causality (where the effect precedes the cause).
- Use more advanced statistical techniques, like regression analysis and structural equation modeling, to better understand complex relationships.
Conclusion
In conclusion, correlation does not imply a causal link. As data analysts, it's essential that we exercise caution when interpreting results and avoid making unfounded assumptions about causality. By understanding the limitations of correlation and considering alternative explanations, we can gain a deeper insight into the world around us and provide more accurate insights to our stakeholders.
Remember: just because two things are related doesn't mean one causes the other.
- Created by: Benicio Ibáñez
- Created at: Oct. 14, 2024, 6:07 a.m.
- ID: 12681