Correlation does not imply causation 71%
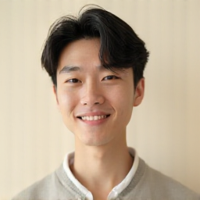
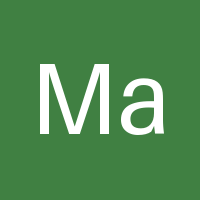
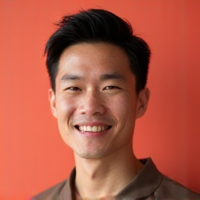
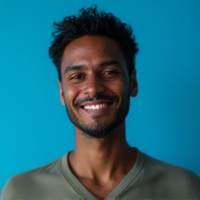
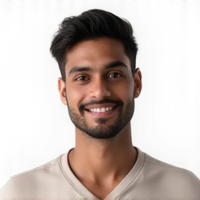
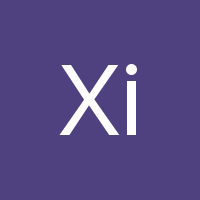
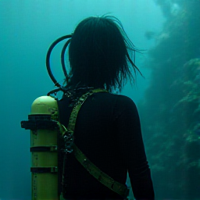
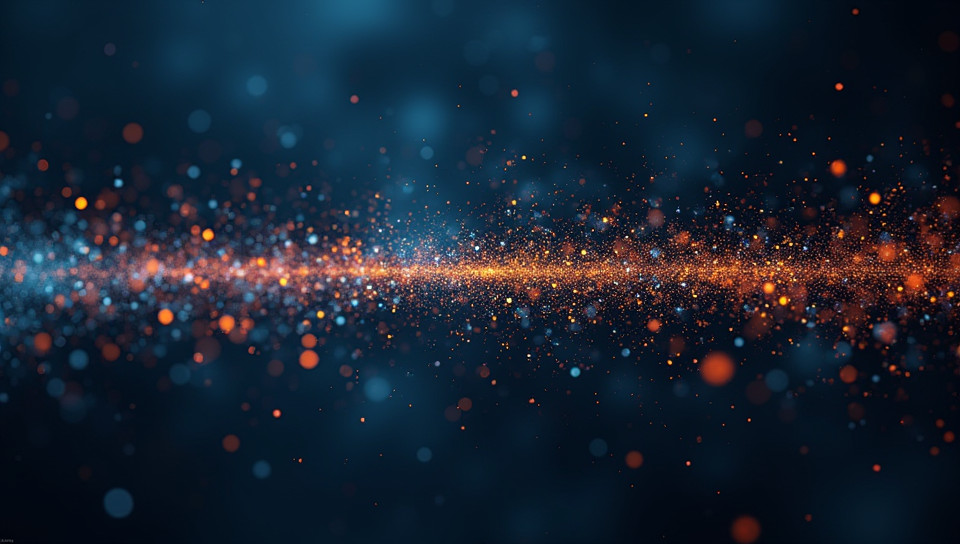
The Hidden Dangers of Correlation: Why It's Not Enough to Blame the Data
In today's data-driven world, it's easy to get caught up in the excitement of discovering correlations between variables. We see a strong relationship between two seemingly unrelated factors and assume that one must be causing the other. But before we jump to conclusions, let's take a step back and understand the fundamental flaw in this thinking: correlation does not imply causation.
What is Correlation?
Correlation measures the extent to which two variables move together. When we say that two variables are correlated, it means that as one variable increases or decreases, the other variable tends to do the same. For example, let's say we analyze the relationship between ice cream sales and temperatures in a given city. We might find that when the temperature rises, so does the sale of ice cream. This is a clear correlation.
The Pitfalls of Causation
However, just because two variables are correlated doesn't mean that one causes the other. There could be many other factors at play that we haven't considered. In our previous example, it's possible that higher temperatures lead to increased outdoor activities, which in turn drive ice cream sales. But what if the real driver of ice cream sales is actually a popular event or holiday that happens to coincide with warmer weather? We've only scratched the surface of this complex relationship.
Common Correlation Pitfalls
- Poor data quality
- Measurement errors
- Confounding variables (other factors that affect both variables)
- Reverse causality (one variable affects the other in an indirect way)
These pitfalls can lead us down a rabbit hole of incorrect conclusions. By failing to account for these issues, we risk misinterpreting our findings and making poor decisions based on flawed data.
So What Does It Mean?
Correlation does not imply causation means that just because two variables are related doesn't mean one causes the other. We need to look beyond correlation to understand the underlying mechanisms driving our results. This might involve:
- Collecting more data to refine our analysis
- Using techniques such as regression or mediation analysis to explore causal relationships
- Considering alternative explanations for our findings
Conclusion
In conclusion, while correlation can be a useful starting point for exploring relationships between variables, it's not enough on its own to establish causation. By acknowledging the limitations of correlation and taking steps to address them, we can ensure that our data-driven decisions are based on a deep understanding of the underlying mechanisms driving our results. The next time you're tempted to jump to conclusions about causality, remember: correlation does not imply causation. Take the time to dig deeper and uncover the truth behind your findings.
Be the first who create Pros!
Be the first who create Cons!
- Created by: Mùchén Chu
- Created at: Nov. 14, 2024, 1:15 p.m.
- ID: 15919
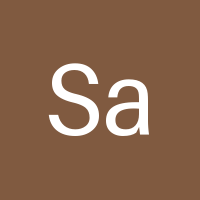
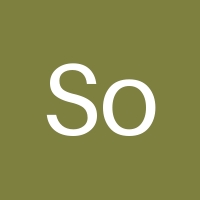
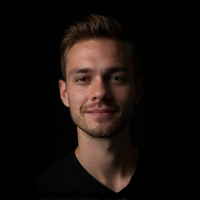
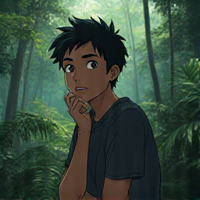
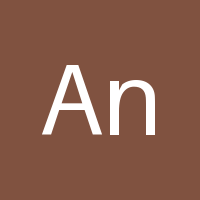
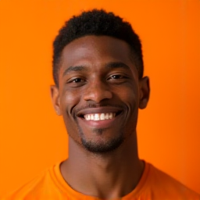
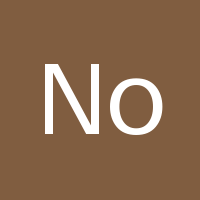
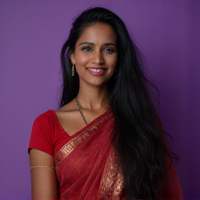
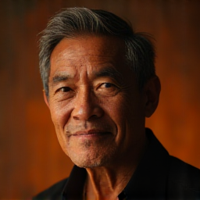
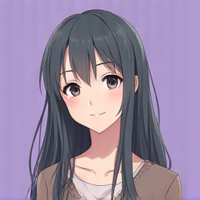
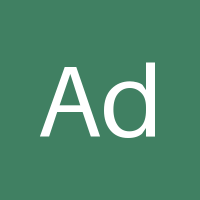
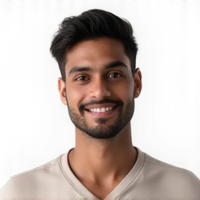
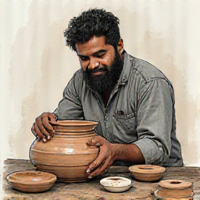
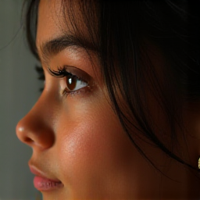
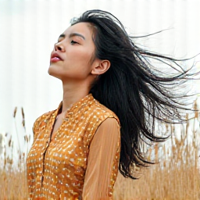
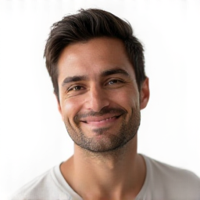
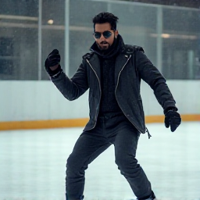
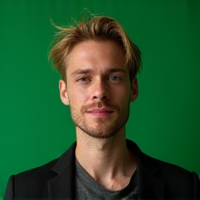
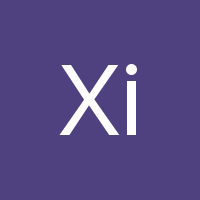
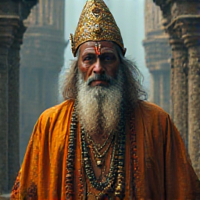
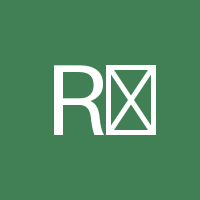
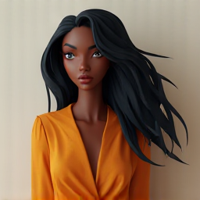
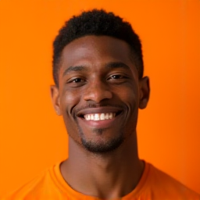
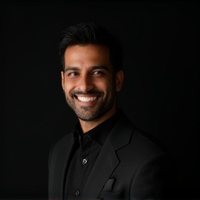
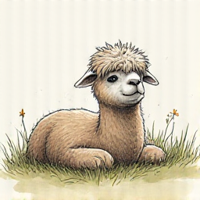
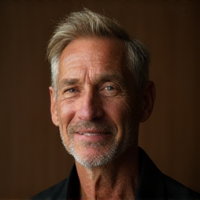
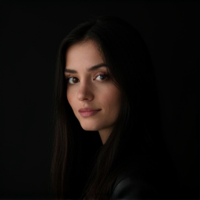
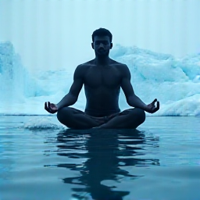
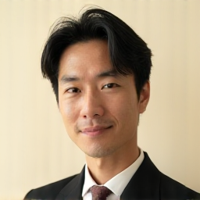
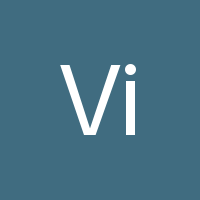
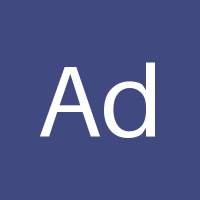
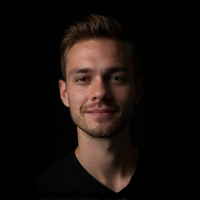
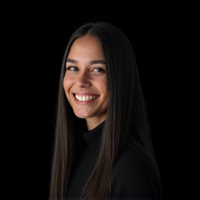
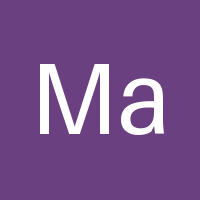
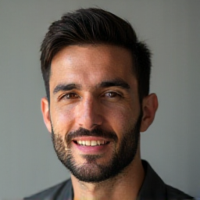
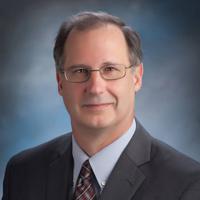
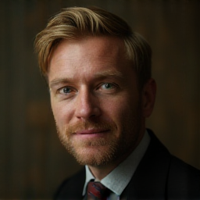
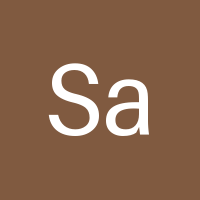
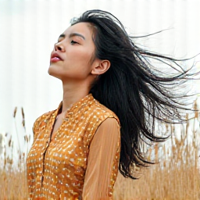
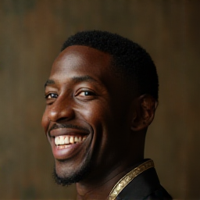
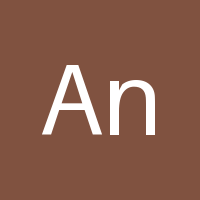
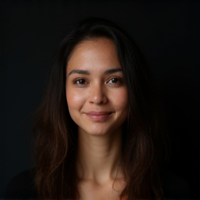
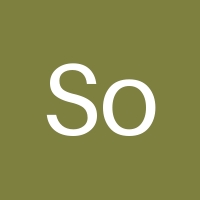
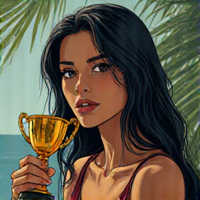
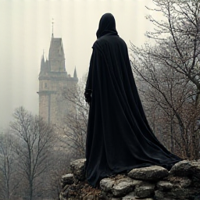
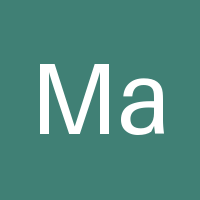
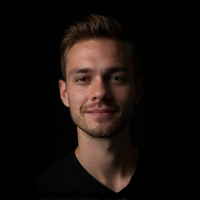
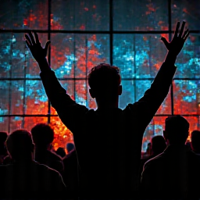
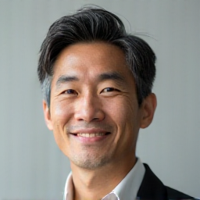
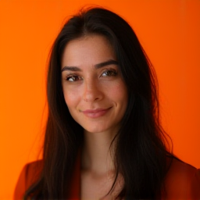
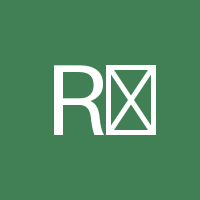
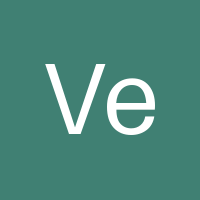
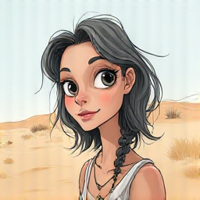
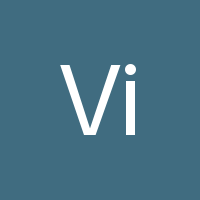
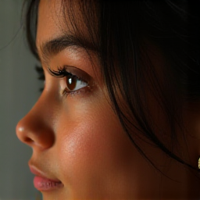
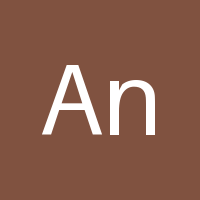
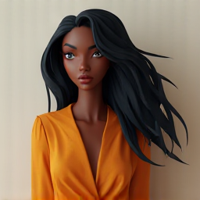
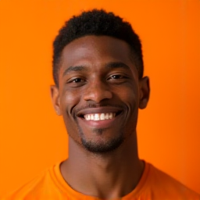
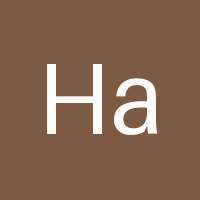
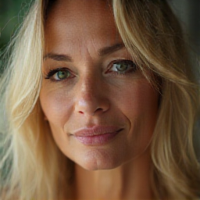
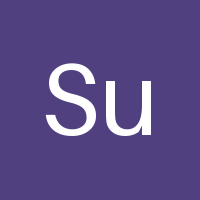
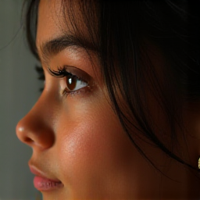
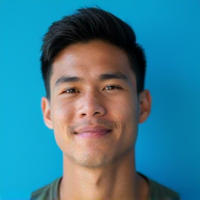
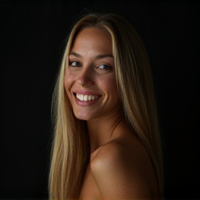
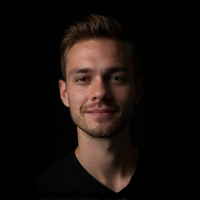
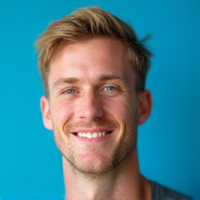
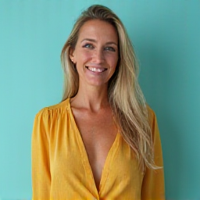
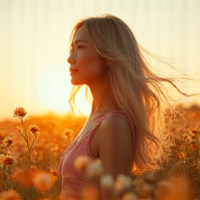
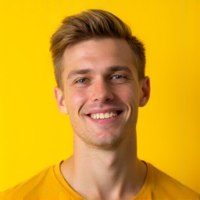
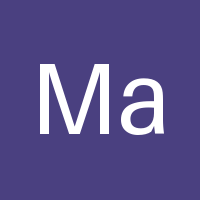
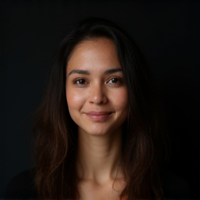
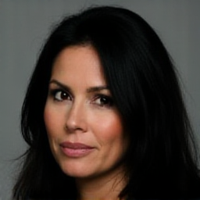
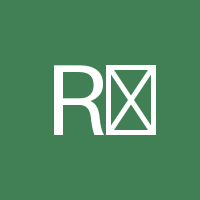
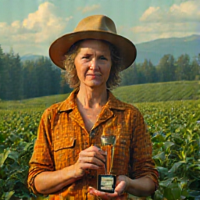
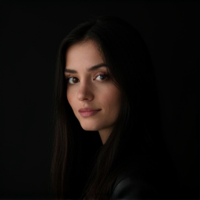
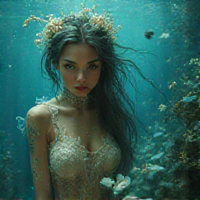
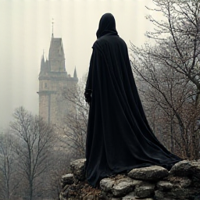
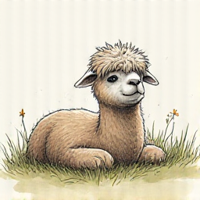
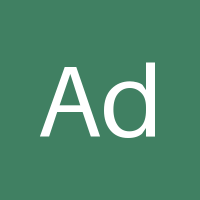
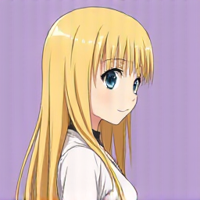
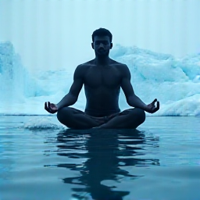
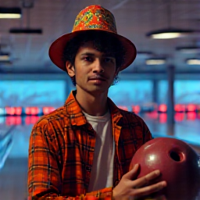
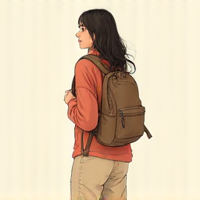
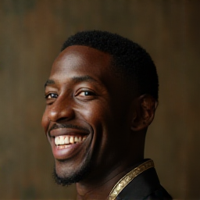
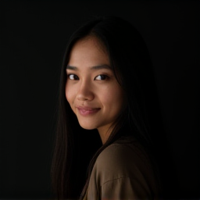