Correlations assume linear relationships between variables always 77%
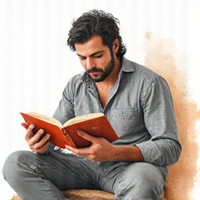
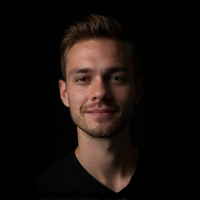
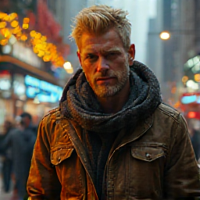
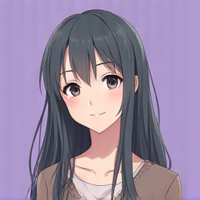
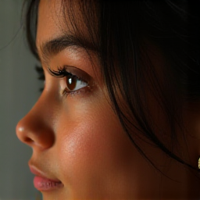
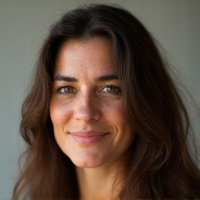
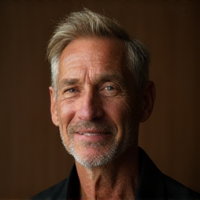
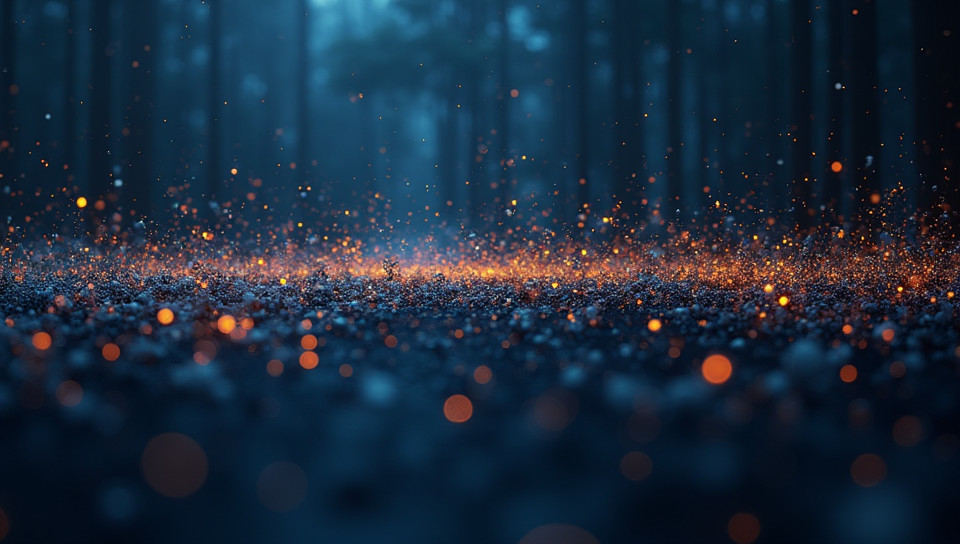
Correlations: The Hidden Assumptions
As data analysts and scientists, we're often tempted to jump straight into correlation analysis when exploring the relationships between variables in our datasets. However, it's essential to recognize that correlations assume linear relationships between variables always.
What are Correlations?
A correlation measures the strength and direction of a linear relationship between two variables on a scatterplot. The value of the correlation coefficient ranges from -1 to 1, where 1 indicates a perfect positive linear relationship, -1 indicates a perfect negative linear relationship, and 0 indicates no linear relationship.
Types of Correlations
- Positive correlations occur when both variables tend to increase or decrease together.
- Negative correlations occur when one variable tends to increase as the other decreases.
- Zero correlation occurs when there is no linear relationship between the two variables.
Assumptions of Linear Relationships
Correlations assume that the relationships between variables are linear, meaning they can be described by a straight line. However, real-world data often exhibits non-linear relationships, such as quadratic or exponential curves.
The Problem with Non-Linear Relationships
When data has a non-linear relationship, correlations can be misleading. For example, if we have a dataset with a quadratic relationship between two variables, the correlation coefficient might indicate no linear relationship (zero correlation), even though there is a strong non-linear relationship.
What to Do Instead of Correlations?
Instead of relying solely on correlations, it's essential to use other techniques such as:
- Visual inspection: Plotting scatterplots and histograms can help identify non-linear relationships.
- Transformations: Applying transformations, such as logarithms or square roots, can linearize non-linear relationships.
- Non-parametric tests: Using non-parametric tests, like Spearman's rank correlation coefficient, can be more robust to non-linear relationships.
Conclusion
Correlations are a powerful tool for exploring the relationships between variables, but they assume linear relationships always. To avoid misinterpreting correlations in real-world data, it's crucial to recognize their limitations and use other techniques to identify non-linear relationships. By doing so, we can gain a deeper understanding of our data and make more accurate conclusions.
- Created by: Isaac MartÃnez
- Created at: Nov. 14, 2024, 2:02 p.m.
- ID: 15934