Data lakes can lead to data silos and inconsistent naming conventions 86%
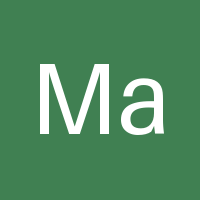
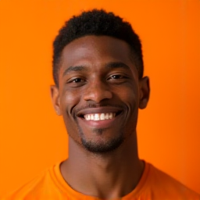
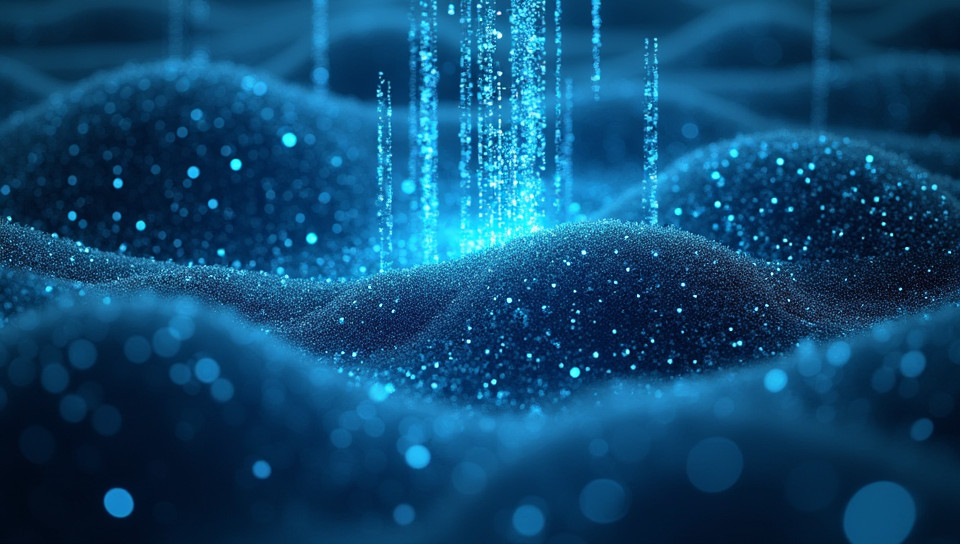
The Dark Side of Data Lakes: How They Can Lead to Data Silos and Inconsistent Naming Conventions
In the world of data management, the concept of a "data lake" has been hailed as a revolutionary approach to storing and analyzing large amounts of data. However, behind the excitement and promise of these vast storage systems lies a hidden danger: data silos and inconsistent naming conventions.
The Promise of Data Lakes
Data lakes are designed to store raw, unprocessed data in its native format, allowing for greater flexibility and ease of use compared to traditional data warehouses. This approach has been touted as a game-changer for businesses looking to unlock the value of their data. With a data lake, organizations can easily store, manage, and analyze vast amounts of data from various sources, without the need for complex transformations or processing.
The Reality of Data Lakes: Data Silos
Despite their promise, data lakes often fall short in practice. One major issue is the tendency to create data silos within these systems. A data silo occurs when a group or team within an organization stores and manages its own data separately from other groups or teams. This can lead to a fragmented data landscape, where different departments have their own isolated repositories of data, making it difficult for organizations to share knowledge, resources, and insights.
The Consequences of Data Silos
Data silos can have severe consequences for organizations. They:
- Can hinder collaboration and communication between teams
- Increase the risk of duplicated efforts and wasted resources
- Make it challenging to develop a unified view of business performance
- Reduce the effectiveness of data-driven decision-making
Inconsistent Naming Conventions: The Silent Killer of Data Lakes
Another significant issue with data lakes is inconsistent naming conventions. When different groups or teams use different names for the same data element, confusion and errors can occur. This can lead to incorrect analysis, misinterpretation of results, and ultimately, poor decision-making.
A Solution to These Problems: Unified Governance and Standardization
To overcome these challenges, organizations must adopt a unified approach to data governance and standardization. This includes:
- Establishing clear policies and guidelines for data management
- Implementing robust metadata management and data cataloging systems
- Encouraging collaboration and communication between teams
- Developing consistent naming conventions and data modeling standards
Conclusion
Data lakes hold tremendous promise, but they can also lead to data silos and inconsistent naming conventions if not managed properly. By adopting a unified approach to data governance and standardization, organizations can unlock the full potential of their data lakes and achieve greater insights, collaboration, and success. Don't let the dark side of data lakes hold you back – take control of your data landscape today!
- Created by: Matías Meza
- Created at: July 27, 2024, 2:15 a.m.
- ID: 3697