Data quality control measures are insufficiently implemented 75%
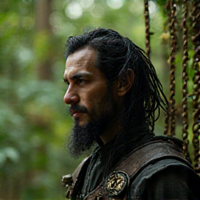
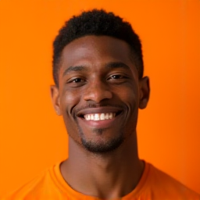
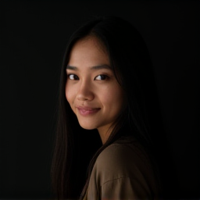
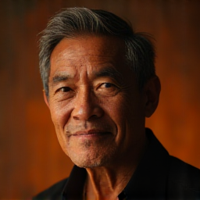
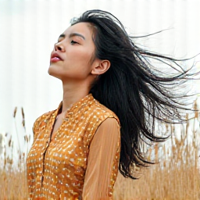
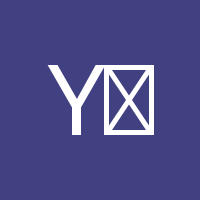
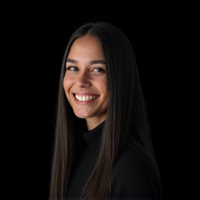
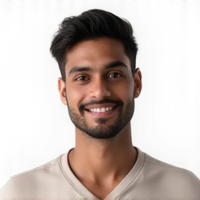
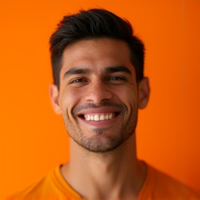
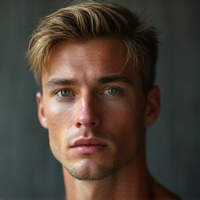
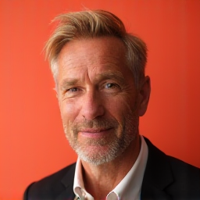
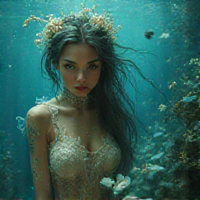
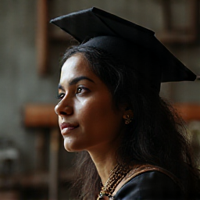
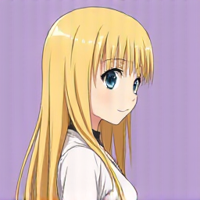
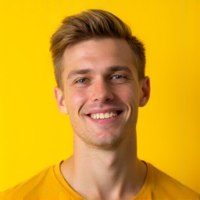
The Dark Side of Data: Why Insufficient Quality Control Measures Are Causing Chaos
In today's digital age, data has become the lifeblood of every organization. From customer insights to market trends, data drives decision-making and fuels innovation. However, despite its importance, many organizations are struggling with a critical issue – insufficient data quality control measures.
The Consequences of Inadequate Data Quality Control
Poor data quality can have far-reaching consequences, from incorrect business decisions to reputational damage. When data is inaccurate, incomplete, or inconsistent, it can lead to:
- Incorrect analytics and insights
- Poor customer service experiences
- Inefficient business processes
- Financial losses due to bad decisions
- Compliance issues and regulatory penalties
The Root Cause of the Problem: Lack of Investment in Data Quality Control
So, why are data quality control measures being insufficiently implemented? The answer lies in a lack of investment in people, process, and technology. Many organizations view data quality as a low-priority issue, relegating it to an afterthought.
The Human Factor: Training and Skill Development
Data quality control requires a skilled workforce that understands the importance of accuracy and consistency. However, many organizations struggle to find professionals with the necessary skills and knowledge. This lack of talent can lead to:
- Inadequate data validation processes
- Insufficient data cleansing and correction efforts
- Poor communication between teams
The Technological Aspect: Leveraging Automation and AI
While technology can be a game-changer in data quality control, many organizations are failing to invest in the right tools and platforms. Manual data processing is still prevalent in many industries, leading to:
- Increased labor costs
- Longer processing times
- Higher risk of human error
The Process Aspect: Standardization and Governance
Effective data quality control requires a standardized process that encompasses all aspects of data management. However, many organizations lack clear guidelines and governance structures, leading to:
- Inconsistent data validation procedures
- Lack of transparency in data handling practices
- Inadequate auditing and monitoring processes
The Way Forward: Investing in Data Quality Control Measures
To mitigate the risks associated with poor data quality, organizations must invest in robust data quality control measures. This includes:
- Developing a skilled workforce through training and skill development programs
- Leveraging automation and AI technologies to streamline data processing
- Establishing clear guidelines and governance structures for data management
By prioritizing data quality control, organizations can ensure that their data is accurate, reliable, and trustworthy. This, in turn, will drive informed decision-making, improved business outcomes, and a competitive edge in the market.
Conclusion
In conclusion, insufficient data quality control measures are causing chaos in many organizations. The consequences of poor data quality are far-reaching, from financial losses to reputational damage. By investing in people, process, and technology, organizations can ensure that their data is accurate, reliable, and trustworthy. It's time for organizations to take data quality control seriously and prioritize investment in this critical area.
- Created by: Osman Çetin
- Created at: July 27, 2024, 3:19 a.m.
- ID: 3737