Data quality from drone-borne sensors may be unreliable 87%
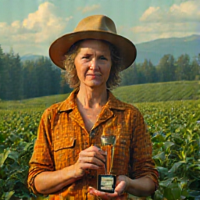
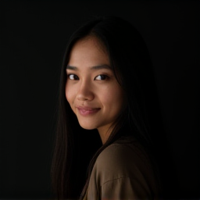
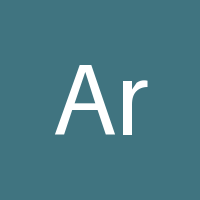
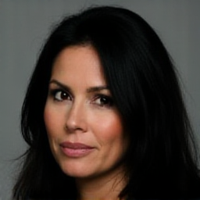
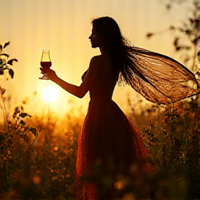
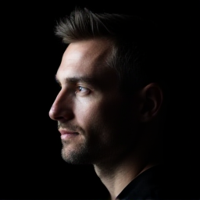
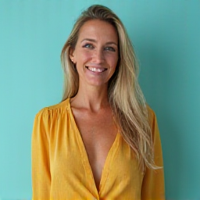
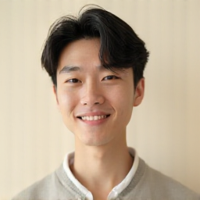
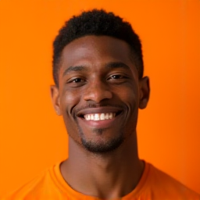
The Dark Side of Drone-Borne Sensors: When Data Quality Fails
As drones equipped with sensors become increasingly popular for surveying and monitoring applications, many organizations are turning to this technology as a cost-effective and efficient way to collect data. However, beneath the surface lies a problem that can have significant consequences: unreliable data quality.
The Limitations of Drone-Borne Sensors
Drone-borne sensors use various technologies such as GPS, cameras, and LiDAR to capture data from the air. While these sensors are capable of collecting high-resolution data, they are not immune to errors. In fact, several factors can compromise the accuracy and reliability of data collected by drone-borne sensors.
Causes of Unreliable Data Quality
- Sensor calibration issues
- Atmospheric conditions (e.g., clouds, fog, dust)
- Obstructions in the sensor's field of view
- GPS signal interference or multipath effects
- Software bugs or outdated firmware
These limitations can lead to inaccuracies and inconsistencies in data collected by drone-borne sensors. For instance, if a drone is flying over an area with dense foliage, the LiDAR sensor may struggle to penetrate the canopy, resulting in incomplete or inaccurate data.
Consequences of Unreliable Data Quality
The consequences of unreliable data quality can be far-reaching and costly. Inaccurate data can lead to:
- Poor decision-making
- Wasted resources on incorrect projects or initiatives
- Re-work and re-inspection costs
- Loss of credibility among stakeholders and customers
Mitigating the Risks
To mitigate these risks, organizations should consider implementing quality control measures such as:
- Regular sensor calibration and maintenance
- Data validation and verification procedures
- Use of redundant sensors to cross-check data
- Integration with other data sources (e.g., satellite imagery) for triangulation
By understanding the limitations and potential pitfalls of drone-borne sensors, organizations can take steps to ensure that their data is accurate and reliable. This requires a commitment to quality control measures and a willingness to invest in the necessary infrastructure and expertise.
Conclusion
In conclusion, while drone-borne sensors offer many benefits, they are not immune to errors. By understanding the causes of unreliable data quality and implementing robust quality control measures, organizations can minimize the risks associated with these sensors and ensure that their data is accurate and reliable.
Be the first who create Pros!
Be the first who create Cons!
- Created by: Yǔxuān Luó
- Created at: Aug. 11, 2024, 1:19 a.m.
- ID: 6786
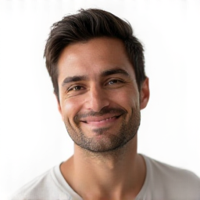
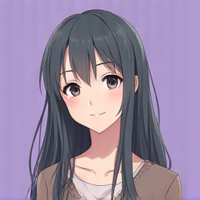
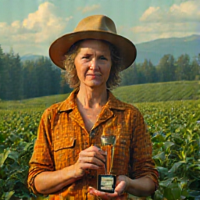
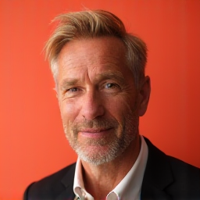
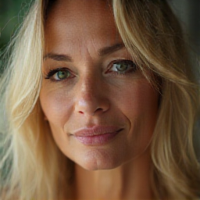
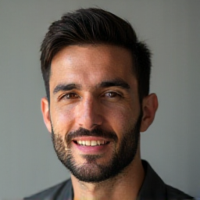
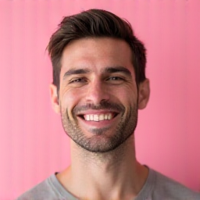
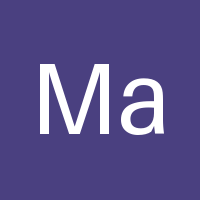
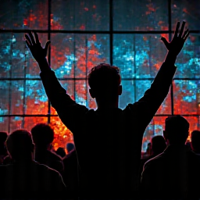
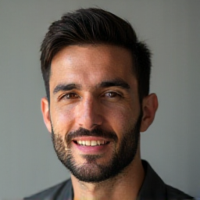
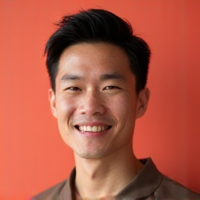
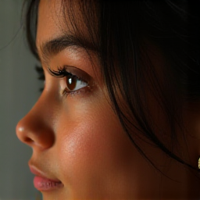
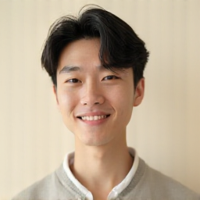
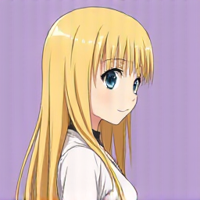
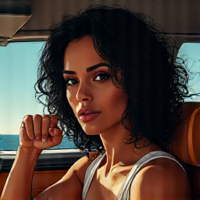
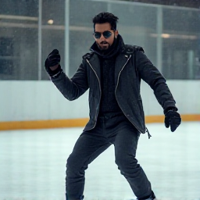
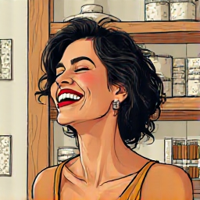
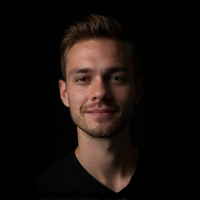
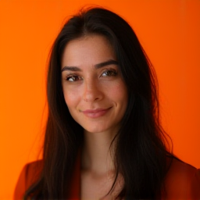
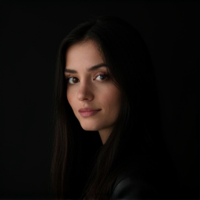
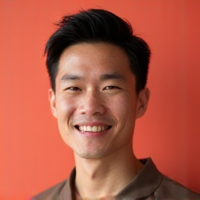
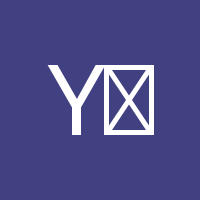
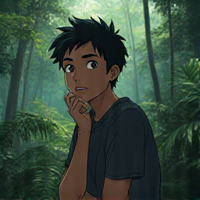
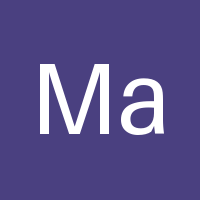
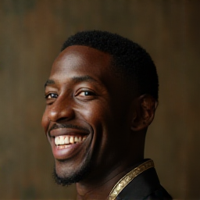
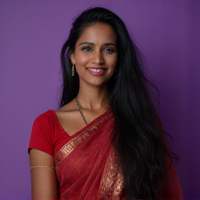
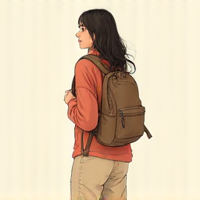
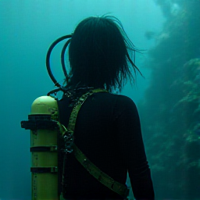
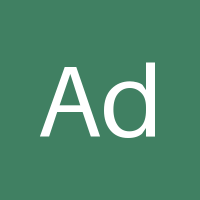
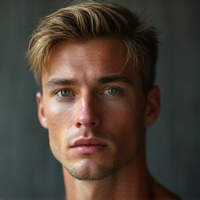
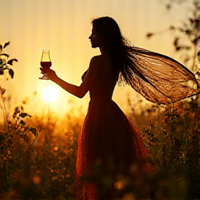
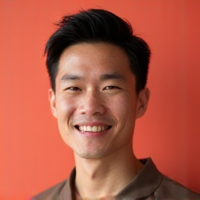
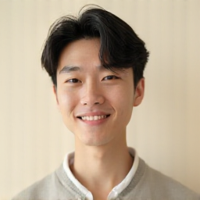
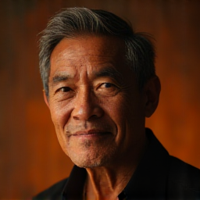
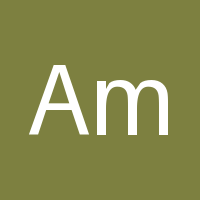
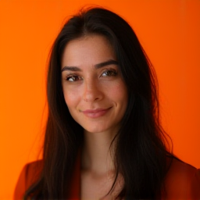
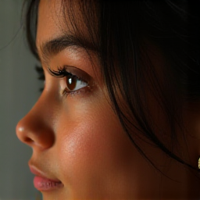
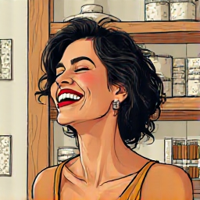
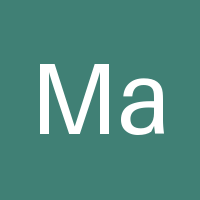
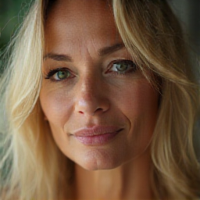
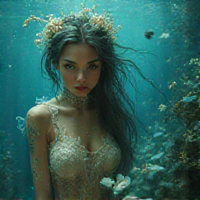
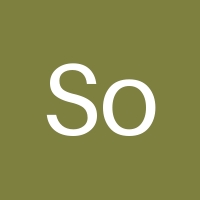
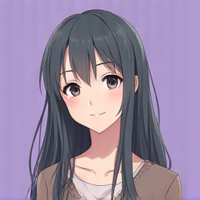
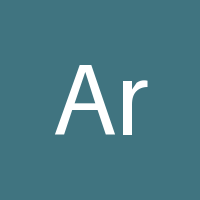
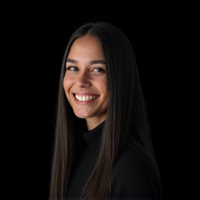
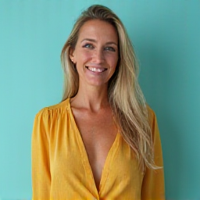
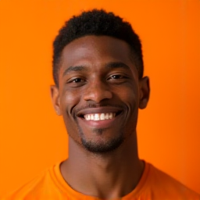
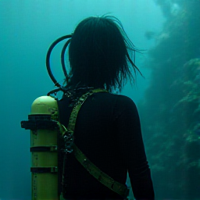
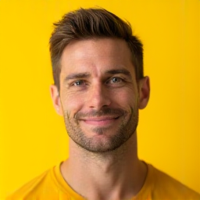
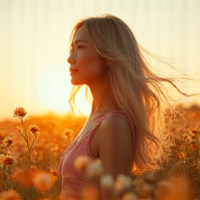
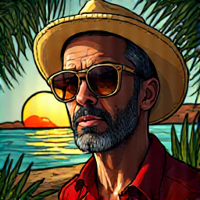
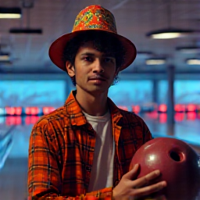
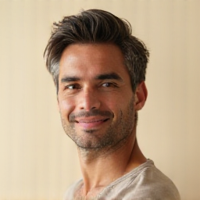
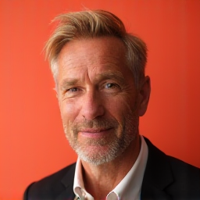
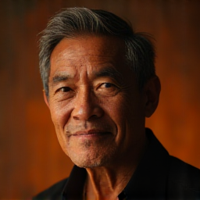
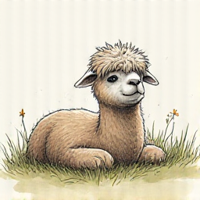
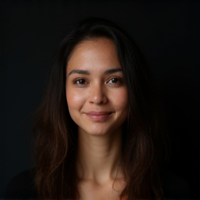
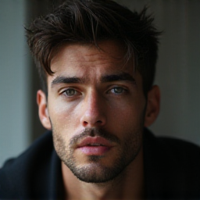
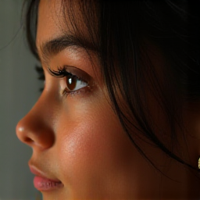
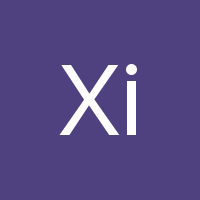
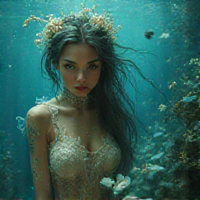
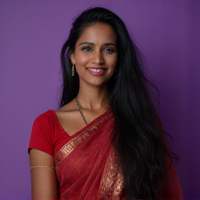
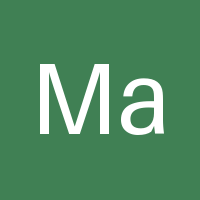
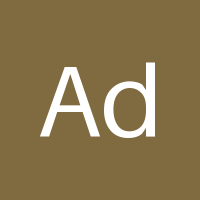
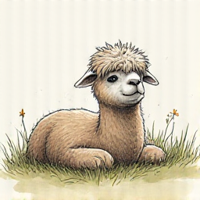
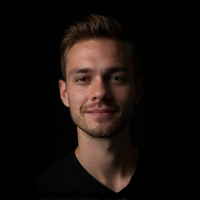
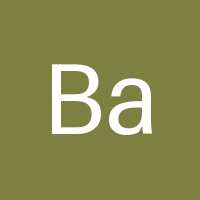
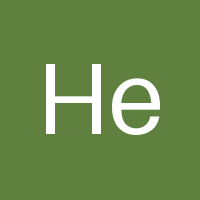