Data quality issues compromise big data analysis 76%
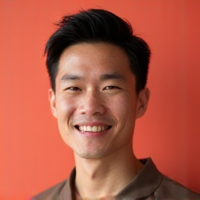
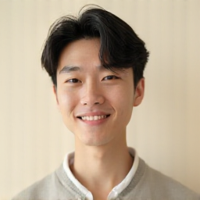
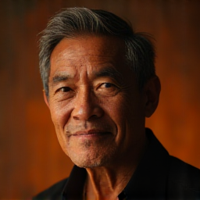
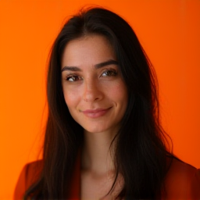
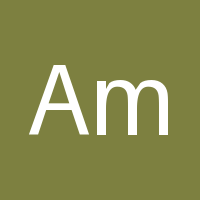
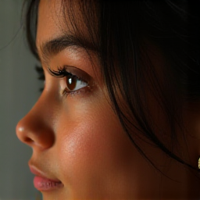
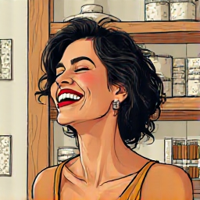
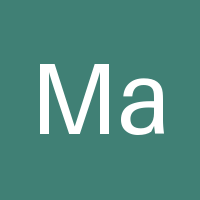
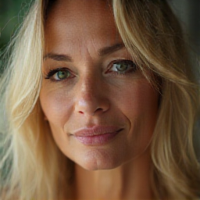
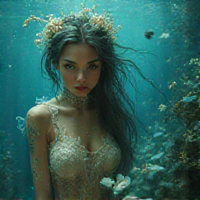
Data Quality Issues Compromise Big Data Analysis
The thrill of diving into big data is often met with excitement and anticipation, but the reality of dealing with poor-quality data can be a letdown. As we generate an increasing amount of data from various sources, it's crucial to understand that data quality issues can significantly compromise our analysis.
What Are Data Quality Issues?
Data quality refers to the accuracy, completeness, and consistency of data. When data is inaccurate, incomplete, or inconsistent, it can lead to flawed conclusions and incorrect insights. This can have serious consequences in various fields such as business, finance, healthcare, and more.
Examples of Data Quality Issues
- Inaccurate customer information
- Missing or duplicate records
- Inconsistent formatting
- Outdated data
- Incorrect encoding schemes
Why Do Data Quality Issues Arise?
Data quality issues can arise from various sources including:
- Human error during data collection or entry
- Technical issues with data storage or transmission
- Lack of standardization in data formats and structures
- Insufficient data validation and verification processes
The Impact of Poor-Quality Data on Big Data Analysis
Poor-quality data can lead to a range of negative consequences, including:
- Inaccurate predictions and forecasts
- Missed opportunities for growth and innovation
- Increased costs due to rework and rectification efforts
- Loss of credibility and trust in data-driven decisions
Best Practices for Ensuring Data Quality
To mitigate the impact of poor-quality data, it's essential to adopt best practices that emphasize data quality. These include:
- Implementing robust data validation and verification processes
- Establishing clear data standards and guidelines
- Providing ongoing training and support for data collectors and analysts
- Regularly auditing and reviewing data quality metrics
Conclusion
Data quality issues are a significant challenge in big data analysis, with far-reaching consequences for businesses and organizations. By understanding the causes of poor-quality data and implementing best practices to ensure data quality, we can improve the accuracy and reliability of our insights and make more informed decisions. It's time to take data quality seriously and prioritize it alongside other key aspects of data management.
- Created by: MarĂa Fernanda Fuentes
- Created at: July 26, 2024, 11:30 p.m.
- ID: 3593