Data quality issues compromise predictive modeling accuracy 86%
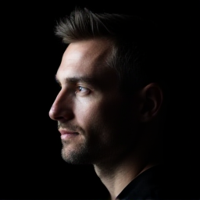
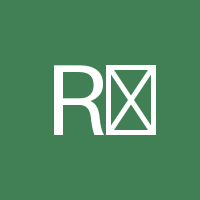
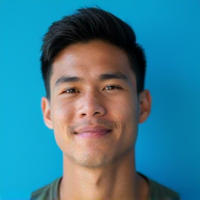
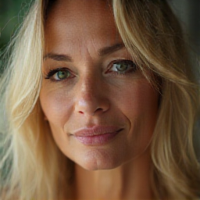
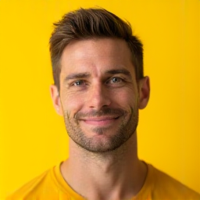
Data Quality Issues Compromise Predictive Modeling Accuracy
As data-driven decision making becomes increasingly prevalent, organizations are turning to predictive modeling as a key tool for gaining insights and driving business outcomes. However, the accuracy of these models relies heavily on the quality of the underlying data. In this article, we'll explore how data quality issues can compromise predictive modeling accuracy and what steps organizations can take to mitigate these risks.
The Importance of Data Quality
High-quality data is essential for building accurate predictive models. This means that data should be complete, consistent, and free from errors or inconsistencies. However, in reality, data quality issues are common and can have significant consequences for predictive modeling accuracy.
Common Data Quality Issues
- Missing values
- Inconsistent formatting
- Duplicate records
- Incorrect or outdated information
- Inaccurate categorization of data points
These issues can arise due to a variety of reasons such as human error, technical glitches, or inadequate data governance processes. Regardless of the cause, the consequences for predictive modeling accuracy can be severe.
The Impact on Predictive Modeling Accuracy
Data quality issues can lead to a range of problems including:
- Biased models that fail to generalize to new data
- Overfitting and underfitting
- Reduced model interpretability
- Inaccurate predictions and poor decision-making
These consequences can have far-reaching implications for organizations, from lost revenue and reputational damage to regulatory non-compliance.
Mitigating Data Quality Risks
To mitigate the risks associated with data quality issues, organizations should implement robust data governance processes that include:
- Regular data cleaning and validation
- Implementation of data quality checks and monitoring tools
- Development of clear data standards and guidelines
- Training for data professionals on data quality best practices
By taking these steps, organizations can ensure that their predictive models are built on high-quality data, leading to more accurate predictions and better decision-making.
Conclusion
Data quality issues are a major threat to the accuracy of predictive modeling. By understanding the common causes and consequences of these issues, organizations can take proactive steps to mitigate these risks. By prioritizing data quality and implementing robust governance processes, organizations can ensure that their predictive models deliver reliable insights and drive business success.
- Created by: Jacob Navarro
- Created at: July 26, 2024, 10:52 p.m.
- ID: 3570