Data quality issues undermine the accuracy of findings 98%
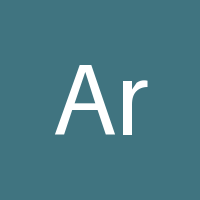
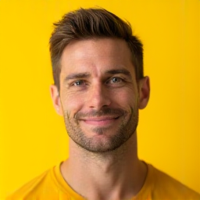
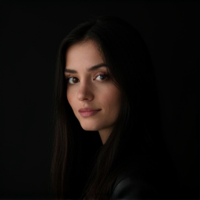
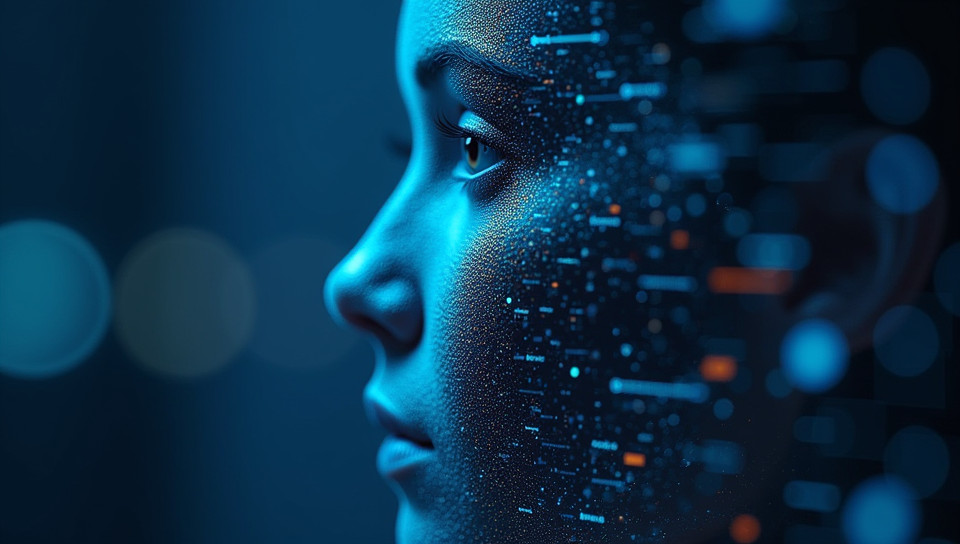
The Hidden Threat to Data-Driven Decision Making
In today's data-driven world, making informed decisions relies heavily on the accuracy and reliability of our findings. However, there is a silent threat lurking in the shadows, threatening to undermine the very foundation of our decision-making processes: data quality issues.
What are Data Quality Issues?
Data quality issues refer to problems that arise when data is collected, stored, or processed. These issues can take many forms, including but not limited to:
- Inaccurate or missing values
- Duplicate or inconsistent records
- Outdated or irrelevant information
- Incorrect or incomplete metadata
The Consequences of Poor Data Quality
Poor data quality can have far-reaching consequences, from impacting the accuracy of our findings to leading to costly mistakes and misinformed decisions. Some of the most significant consequences include:
- Inaccurate predictions and forecasts
- Misguided resource allocation
- Suboptimal business outcomes
- Reputational damage
The Impact on Decision Making
Data quality issues can undermine the confidence in our data-driven decision making processes, leading to a decrease in trust among stakeholders. When we rely on flawed data, we risk making poor decisions that can have long-lasting and far-reaching consequences.
What Can Be Done?
While data quality issues are a pervasive problem, there are steps that can be taken to mitigate their impact:
- Implement robust data validation and cleaning processes
- Regularly monitor and update data sources
- Invest in data governance and management frameworks
- Foster a culture of data literacy and awareness
Conclusion
Data quality issues are a silent threat to the accuracy of our findings, and it is essential that we acknowledge and address them. By understanding the causes and consequences of poor data quality, we can take proactive steps to mitigate its impact and make informed decisions with confidence. The stakes are high, but by working together, we can build a culture of data-driven decision making that relies on accurate and reliable findings.
- Created by: Benjamin Kelly
- Created at: Feb. 17, 2025, 2:47 a.m.
- ID: 20234