Data scientists work with big data to uncover hidden patterns 67%
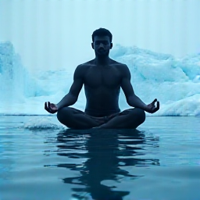
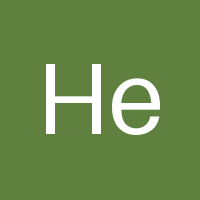
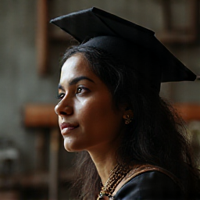
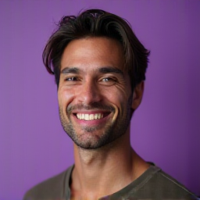
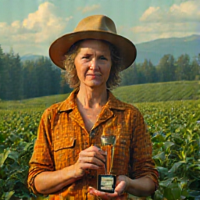
Unlocking Insights from Big Data: The Power of Data Science
In today's digital age, data is being generated at an unprecedented rate and volume. Every click, every swipe, every purchase – it all contributes to the vast ocean of big data that organizations are grappling with. Amidst this chaos lies a treasure trove of hidden patterns, waiting to be unearthed by data scientists who possess the skill and expertise to navigate this complex landscape.
What is Big Data?
Big data refers to the large, complex, and diverse sets of data that traditional data processing tools and techniques cannot handle efficiently. It encompasses structured, semi-structured, and unstructured data from various sources such as social media, IoT devices, sensors, log files, and more. The sheer volume, velocity, and variety of big data pose significant challenges for organizations seeking to derive meaningful insights from it.
Challenges in Working with Big Data
Working with big data is not a trivial task. It requires specialized skills, infrastructure, and tools to handle the massive amounts of data being generated every second. Some of the key challenges faced by data scientists when working with big data include:
- Handling high-volume and high-speed data streams
- Dealing with missing or inconsistent data values
- Integrating data from diverse sources and formats
- Identifying meaningful patterns and correlations
The Role of Data Science in Uncovering Hidden Patterns
Data science is an interdisciplinary field that combines elements of computer science, statistics, and domain expertise to extract insights from complex datasets. By applying advanced techniques such as machine learning, natural language processing, and data visualization, data scientists can uncover hidden patterns and trends within big data that would otherwise remain invisible.
Real-World Applications of Data Science
Data science has numerous real-world applications across industries such as healthcare, finance, marketing, and more. Some examples include:
- Predictive maintenance in manufacturing to prevent equipment failures
- Personalized medicine based on genetic profiles
- Targeted advertising using customer behavior data
- Risk management in financial institutions using credit scoring models
Conclusion
Data scientists play a vital role in unlocking the secrets hidden within big data. By developing and applying advanced techniques, they can help organizations make informed decisions, improve operational efficiency, and stay ahead of the competition. As the world continues to generate more data than ever before, the demand for skilled data scientists will only continue to grow. Whether you're a seasoned professional or just starting out in your career, the field of data science offers numerous opportunities for growth and development.
- Created by: Pari Singh
- Created at: July 27, 2024, 12:46 a.m.
- ID: 3641