Data silos and disparate systems hinder effective predictive maintenance 70%
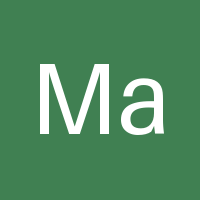
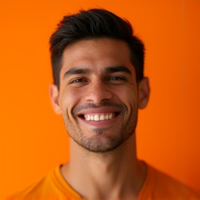
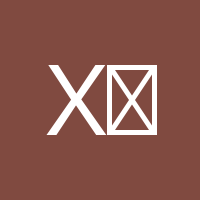
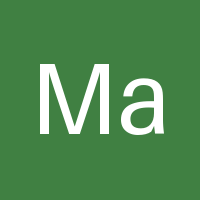
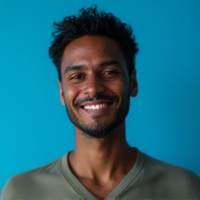
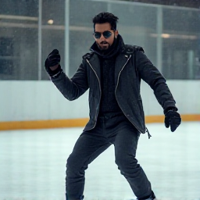
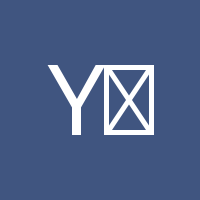
Predictive Maintenance: A Threatened Promise
The promise of predictive maintenance has long been touted as a game-changer for industries reliant on complex machinery and equipment. By leveraging data analytics, companies can identify potential issues before they become major problems, reducing downtime and increasing overall efficiency. However, the reality is that many organizations are still struggling to realize this promise due to one key obstacle: data silos and disparate systems.
The Problem with Data Silos
Data silos refer to isolated repositories of data that are not easily accessible or shareable across different departments or systems within an organization. These silos can be found in various forms, including:
- Machine-generated data from equipment sensors
- Maintenance records and logs
- Quality control data from production lines
- Financial information and budgeting data
Each of these silos contains valuable insights that could inform predictive maintenance strategies. However, when they are not properly integrated or shared, the potential benefits of predictive maintenance are severely limited.
The Consequences of Disparate Systems
Disparate systems refer to different software applications or platforms used by various departments within an organization. These systems often have unique data structures and interfaces, making it difficult to integrate them seamlessly. This leads to:
- Inefficient workflows
- Duplicate data entry
- Inconsistent reporting
- Difficulty in accessing critical information
The result is a disjointed view of the entire operation, where predictive maintenance strategies are hindered by incomplete or inaccurate data.
Breaking Down Silos and Unifying Systems
So how can organizations overcome these challenges? The key lies in implementing a centralized platform that integrates data from various silos and disparate systems. This platform should:
- Support real-time data exchange
- Provide user-friendly interfaces for maintenance personnel
- Offer advanced analytics capabilities
- Integrate with existing systems and software applications
By breaking down data silos and unifying disparate systems, organizations can unlock the full potential of predictive maintenance.
Conclusion
The promise of predictive maintenance is within reach, but only if we address the obstacles that stand in our way. By recognizing the challenges posed by data silos and disparate systems, organizations can take proactive steps to integrate their data and systems. This will enable them to make informed decisions, optimize their operations, and ultimately achieve greater efficiency and productivity. The time for change is now – are you ready to unlock the full potential of predictive maintenance?
- Created by: Marcia Costa
- Created at: July 27, 2024, 9:52 a.m.
- ID: 3969