Difficulty in finding meaningful patterns in big data 62%
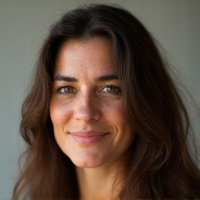
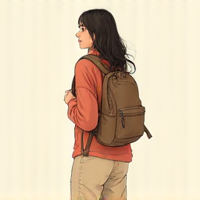
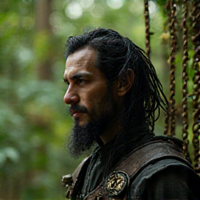
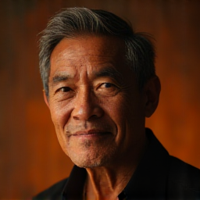
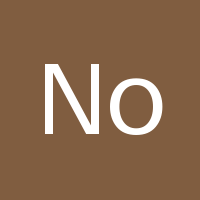
The Elusive Patterns in Big Data: Why Meaningful Insights Remain Elusory
Big data has revolutionized the way we approach business, healthcare, and research. With the ability to collect and store vast amounts of information, organizations can gain valuable insights that inform their decisions. However, this sea of data comes with a significant challenge – finding meaningful patterns amidst the noise.
The Problem of Pattern Extraction
As data volumes grow exponentially, so does the complexity of analyzing them. Traditional methods of pattern recognition, such as statistical analysis and machine learning algorithms, become increasingly ineffective in extracting valuable insights from large datasets. This is because these methods are designed to identify patterns within smaller, more manageable sets of data.
The Curse of Dimensionality
One major obstacle to pattern extraction is the curse of dimensionality. As the number of variables (features) increases, the distance between data points grows exponentially, making it difficult to visualize and analyze the data in its entirety. This results in a phenomenon known as "feature fatigue," where models become overwhelmed by the sheer number of features and fail to capture meaningful relationships.
The Importance of Context
Another challenge lies in understanding the context behind the data. Without domain-specific knowledge or contextual information, it's challenging to identify relevant patterns. For instance, in medical research, understanding the nuances of patient demographics, comorbidities, and treatment outcomes is crucial for identifying predictive patterns.
- Examples of such challenges include:
- Identifying correlations between seemingly unrelated variables
- Understanding the impact of biases on data interpretation
- Recognizing the limitations of models trained on incomplete or noisy data
The Need for Innovative Solutions
To overcome these challenges, innovative solutions are needed. These may involve:
- Developing new machine learning algorithms that can handle high-dimensional data and complex relationships
- Incorporating domain-specific knowledge into model development to improve interpretability
- Utilizing techniques such as feature selection and dimensionality reduction to simplify analysis
Conclusion
Extracting meaningful patterns from big data is an intricate task. It requires a combination of technical expertise, domain-specific knowledge, and innovative solutions. By acknowledging the challenges associated with pattern extraction and addressing them head-on, we can unlock the full potential of big data and make informed decisions that drive business growth, improve healthcare outcomes, and advance scientific discovery.
- Created by: Osman Çetin
- Created at: July 27, 2024, 7:57 a.m.
- ID: 3905