Disorganized data hinders efficient querying in large datasets 85%
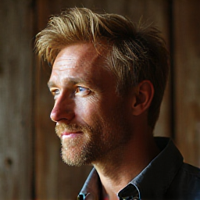
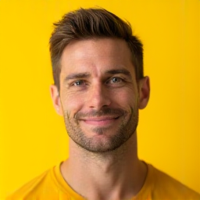
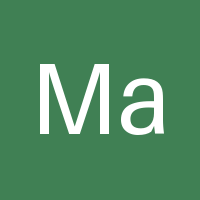
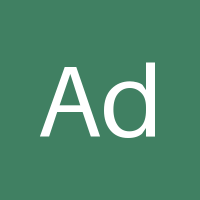
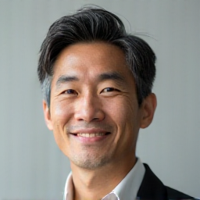
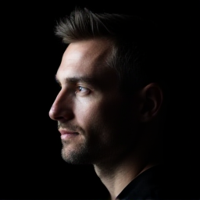
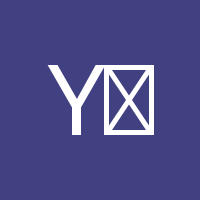
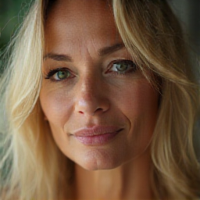
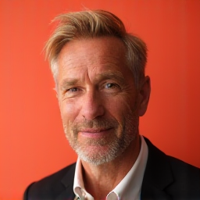
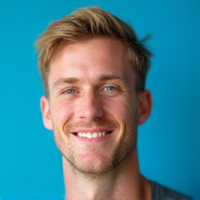
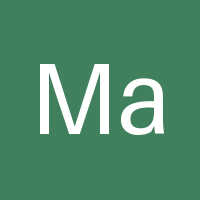
Disorganized Data: The Silent Killer of Efficient Querying
In today's data-driven world, the ability to extract insights from large datasets is crucial for making informed decisions. However, this process can be hindered by a common obstacle: disorganized data.
What is Disorganized Data?
Disorganized data refers to data that lacks structure, consistency, and standardization. It can take many forms, including:
- Data with missing or inconsistent values
- Data stored in multiple formats (e.g., CSV, Excel, JSON)
- Data with duplicate or redundant entries
- Data that is not properly normalized
The Consequences of Disorganized Data
Disorganized data can have far-reaching consequences for organizations that rely on it to make decisions. Some of the most significant effects include:
Increased Query Time
When data is disorganized, queries take longer to execute, leading to delayed insights and frustrated users.
Inaccurate Results
Disorganized data can lead to inaccurate or incomplete results, which can have serious consequences in fields like healthcare, finance, and marketing.
Data Loss
Poorly organized data can be difficult to recover, resulting in lost business opportunities, revenue, and competitiveness.
The Impact on Efficient Querying
Efficient querying relies on well-organized data that is easily accessible and analyzable. Disorganized data hinders this process by:
- Making it difficult for databases to optimize queries
- Increasing the risk of errors and inconsistencies
- Requiring more resources (time, money, personnel) to maintain and update data
Strategies for Improving Data Organization
To overcome the challenges posed by disorganized data, organizations can implement the following strategies:
- Implement a data governance framework to standardize data management practices
- Use data normalization techniques to ensure consistent and accurate data
- Develop robust data validation rules to catch errors and inconsistencies early on
- Utilize tools like ETL (Extract, Transform, Load) software to streamline data integration
Conclusion
Disorganized data is a significant obstacle to efficient querying in large datasets. By understanding the causes and consequences of disorganized data, organizations can take proactive steps to improve their data management practices. By doing so, they can unlock faster query times, more accurate results, and better decision-making capabilities – ultimately driving business success and competitiveness.
- Created by: Benjamin Kelly
- Created at: July 27, 2024, 4:17 a.m.
- ID: 3774