Domain-specific knowledge trumps neural network models 55%
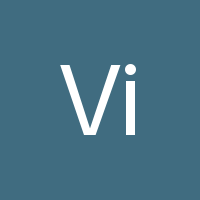
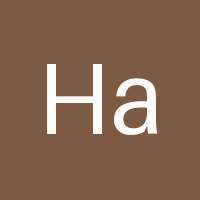
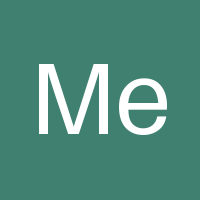
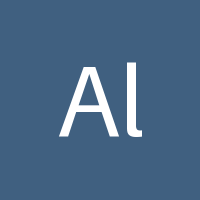
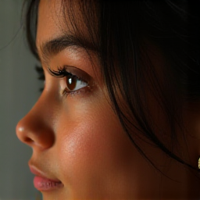
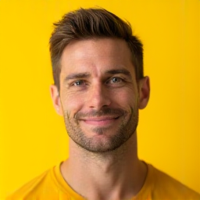
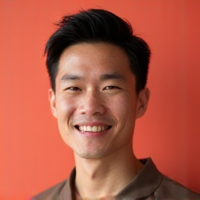
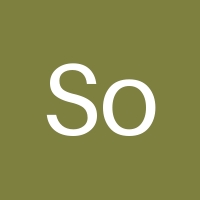
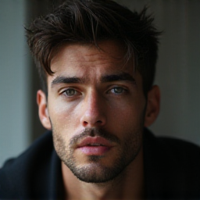
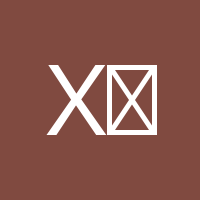
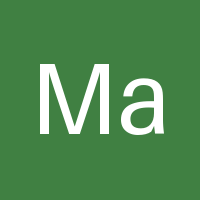
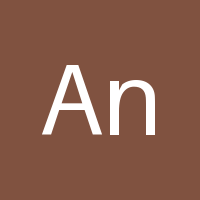
Domain-specific knowledge trumps neural network models
In today's AI-driven landscape, many experts believe that neural networks are the holy grail of machine learning. However, there is a growing body of evidence that suggests domain-specific knowledge can be just as powerful, if not more so, than these complex algorithms.
The Limits of Neural Networks
Neural networks have been incredibly successful in tasks such as image recognition and natural language processing. They are capable of learning from large datasets and improving their performance over time. However, they rely on statistical patterns and correlations within the data to make predictions or decisions. This can lead to issues when dealing with:
- Unusual inputs or edge cases
- Limited training data
- Concept drift (when the underlying concept changes)
The Power of Domain-specific Knowledge
Domain-specific knowledge refers to the unique understanding and expertise that comes from working in a specific field or industry. It encompasses the nuances, subtleties, and complexities that are difficult to capture with neural networks alone. This type of knowledge is often acquired through years of experience, education, and real-world application.
Why Domain-specific Knowledge Trumps Neural Network Models
There are several reasons why domain-specific knowledge can be more effective than neural network models:
- Contextual understanding: Domain experts have a deep understanding of the context in which the data is being used. This allows them to make more informed decisions and avoid common pitfalls.
- Knowledge integration: Experts can integrate multiple sources of information and use their judgment to arrive at a decision, rather than relying solely on statistical patterns.
- Transfer learning: Domain-specific knowledge can be applied across different domains or tasks, allowing experts to adapt quickly to new situations.
Conclusion
While neural networks have their strengths, domain-specific knowledge is often the key to success in many fields. By leveraging this unique expertise, individuals and organizations can make more informed decisions, avoid common pitfalls, and achieve better outcomes. As we move forward in an increasingly complex world, it's essential to recognize the value of domain-specific knowledge and incorporate it into our decision-making processes.
- Created by: Sofia David
- Created at: July 27, 2024, 11:13 p.m.
- ID: 4077