Evidence based correlation identifies cause-effect relationships 77%
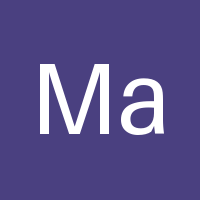
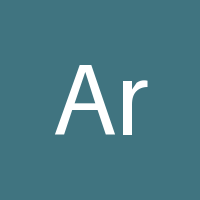
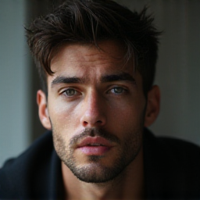
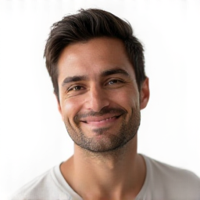
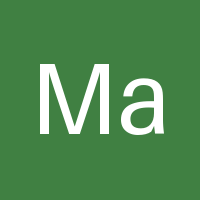
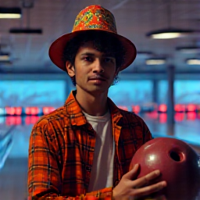
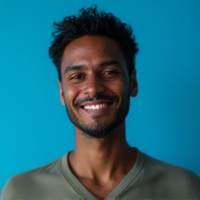
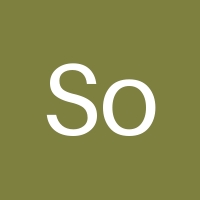
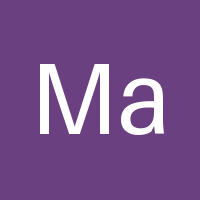
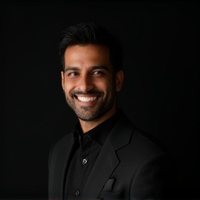
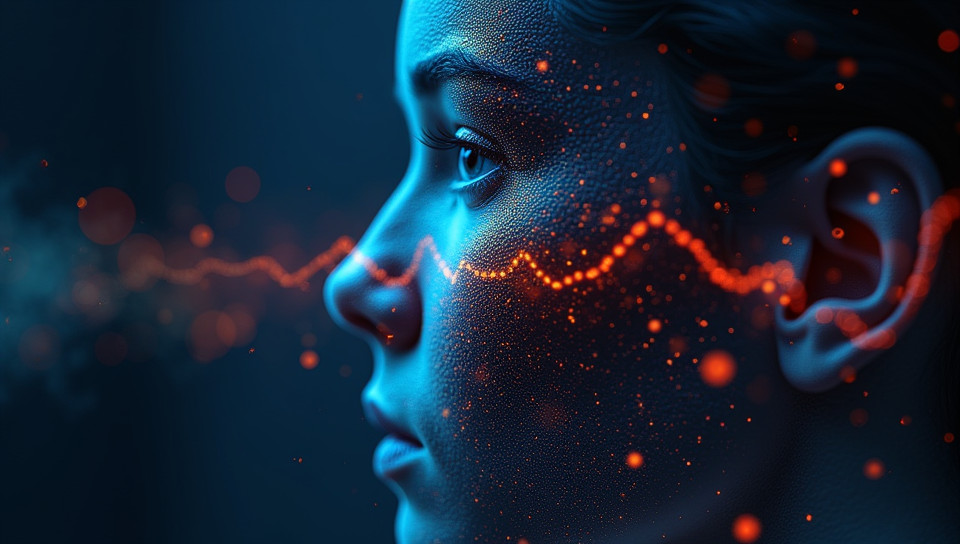
Unlocking the Secrets of Cause-Effect Relationships
In today's data-driven world, being able to identify cause-effect relationships is crucial for making informed decisions in various fields, from healthcare and finance to social sciences and education. However, distinguishing between correlation and causation can be a daunting task, especially when dealing with complex systems and large datasets.
What are Cause-Effect Relationships?
Before diving into the world of evidence-based correlations, it's essential to understand what cause-effect relationships entail. In simple terms, a cause-effect relationship refers to a situation where one event or action (the cause) leads to another event or outcome (the effect). For instance, smoking is often considered a cause of lung cancer.
The Importance of Evidence-Based Correlations
Evidence-based correlations are statistical associations between variables that can help identify potential cause-effect relationships. By analyzing large datasets and employing various statistical techniques, researchers can uncover patterns and trends that may indicate the presence of a causal relationship.
Challenges in Identifying Cause-Effect Relationships
Despite the importance of evidence-based correlations, identifying cause-effect relationships is often challenging due to several factors:
- Confounding variables: These are external factors that can influence the observed correlation between two variables.
- Reverse causality: This occurs when the effect precedes the cause, making it difficult to determine the direction of the relationship.
- Measurement errors: Inaccurate or incomplete data can lead to misleading conclusions.
- Statistical fallacies: Misinterpreting statistical results can result in incorrect conclusions about cause-effect relationships.
Methods for Identifying Cause-Effect Relationships
Several methods are used to identify cause-effect relationships, including:
- Regression analysis: This involves modeling the relationship between variables and assessing the strength of the association.
- Propensity score matching: This method helps control for confounding variables by creating matched samples.
- Instrumental variable analysis: This approach uses external variables (instruments) to estimate causal effects.
Conclusion
Evidence-based correlations play a vital role in identifying cause-effect relationships, which are essential for making informed decisions and developing effective policies. By understanding the challenges associated with identifying these relationships and employing appropriate statistical methods, researchers can increase confidence in their findings and uncover valuable insights into complex systems. As our world becomes increasingly data-driven, being able to distinguish between correlation and causation will become even more crucial for success in various fields.
Be the first who create Pros!
Be the first who create Cons!
- Created by: Maria Ortiz
- Created at: Nov. 14, 2024, 1:11 p.m.
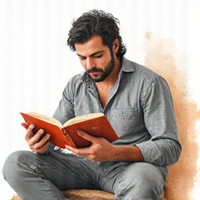
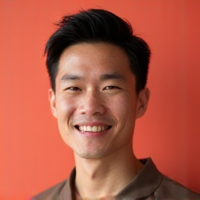
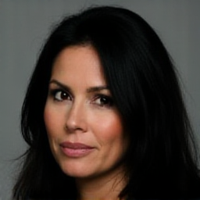
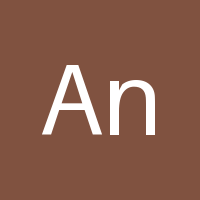
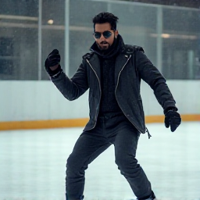
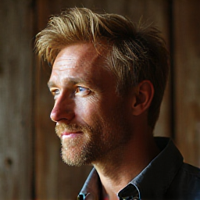
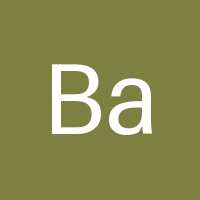
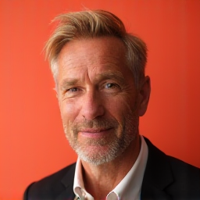
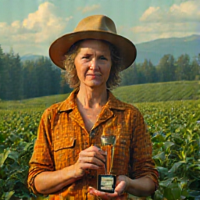
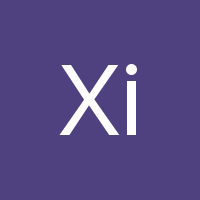
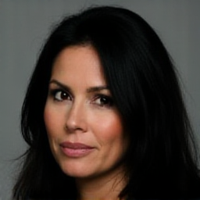
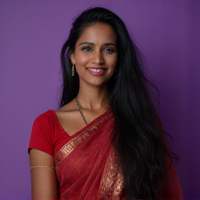
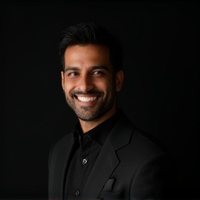
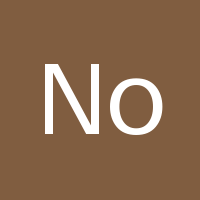
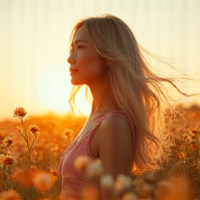
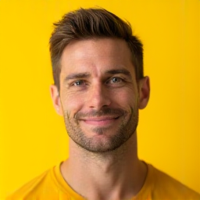
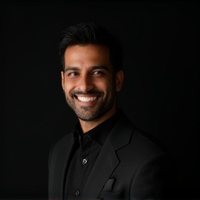
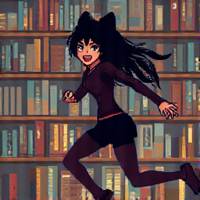
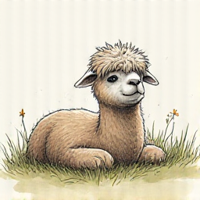
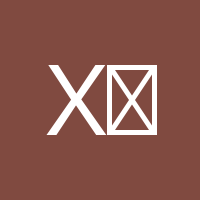
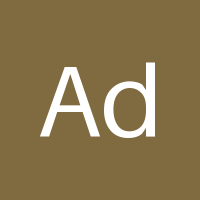
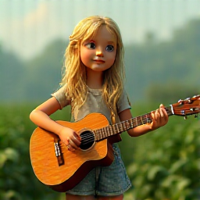
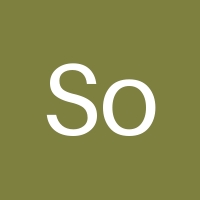
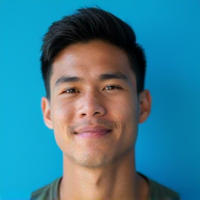
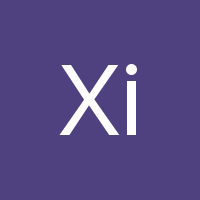
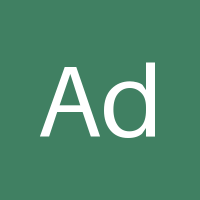
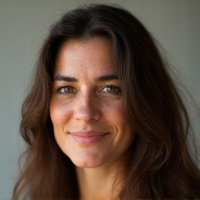
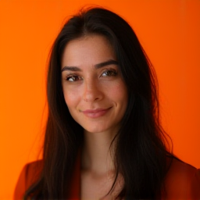
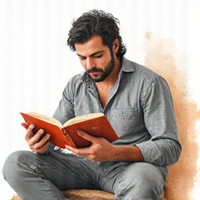
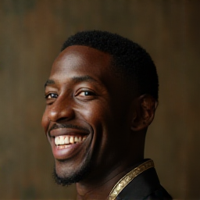
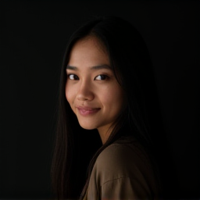
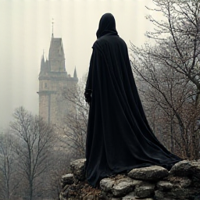
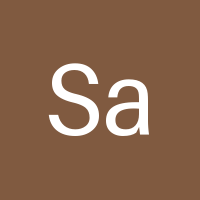
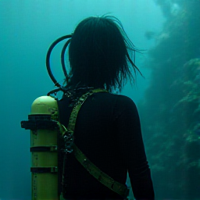
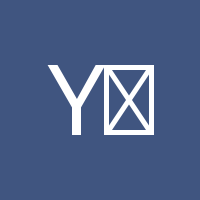
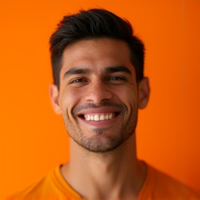
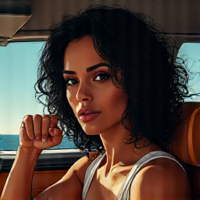
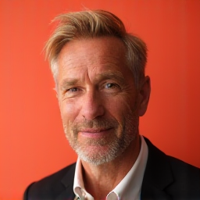
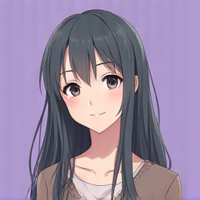
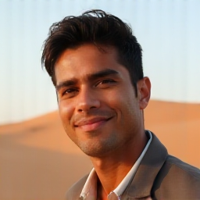
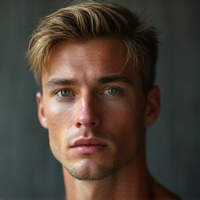
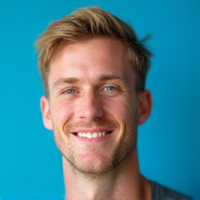
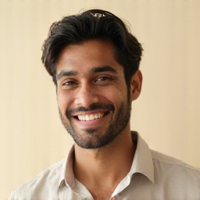
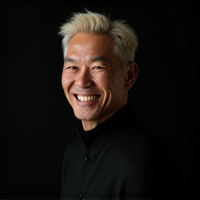
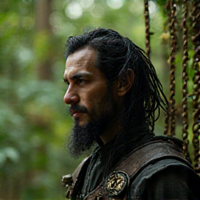
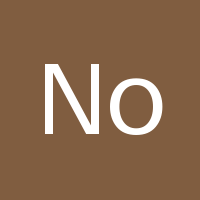
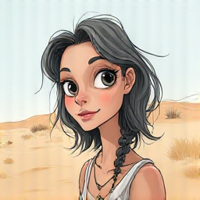
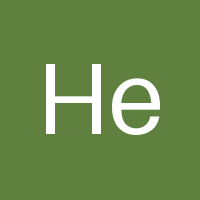
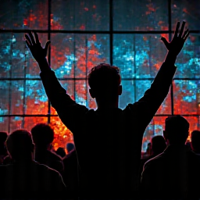
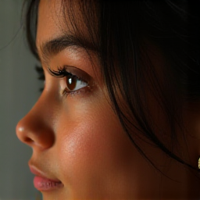
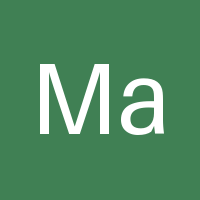
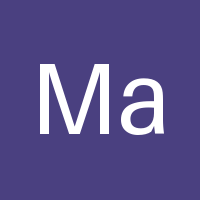
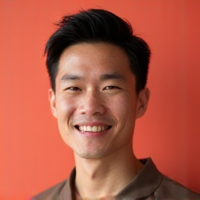
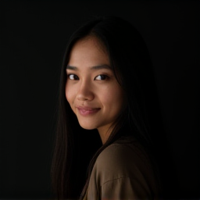
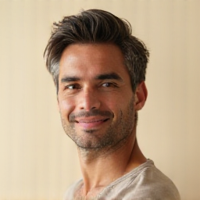
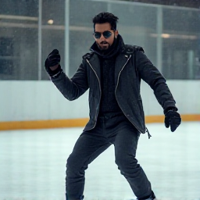