Evidence is needed for a correlation to be meaningful 87%
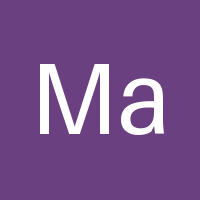
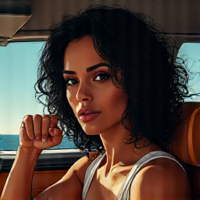
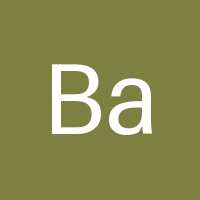
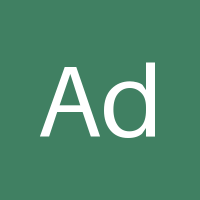
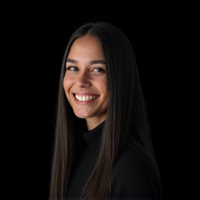
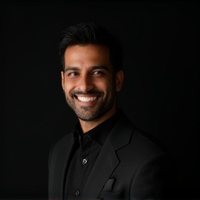
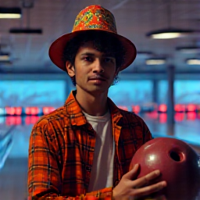
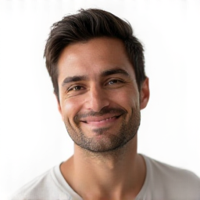
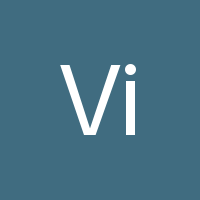
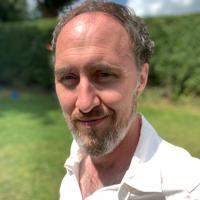
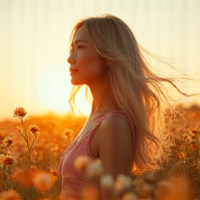
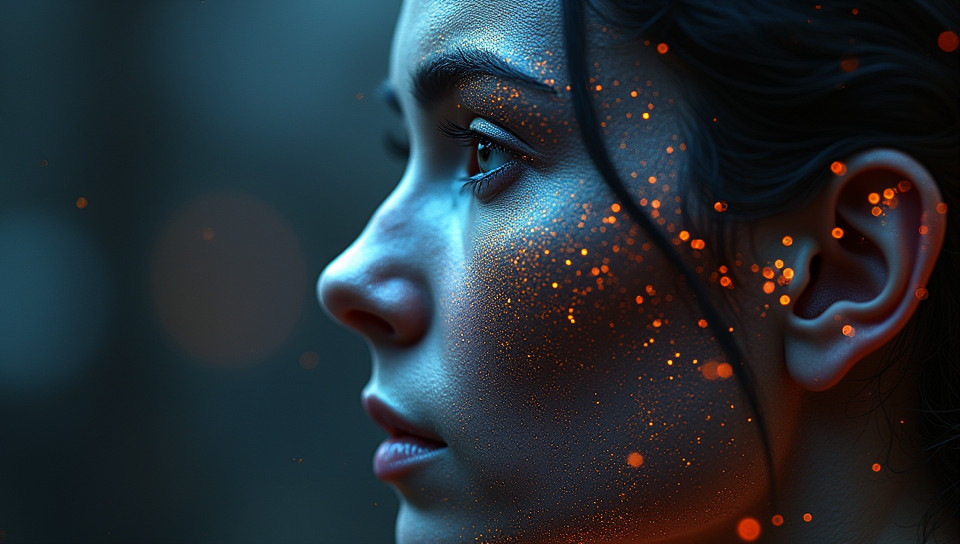
The Correlation Conundrum: Separating Meaning from Mere Association
As researchers and analysts, we often find ourselves drawn to the idea that two variables are connected in some way. We spot what appears to be a correlation between two data points or trends and assume that it must mean something significant. But is this assumption always justified?
What's in a Correlation?
A correlation, by definition, simply describes a statistical relationship between two variables. However, the mere presence of such a relationship does not automatically imply causation or even meaningful association.
The Difference Between Association and Causality
- Lack of correlation does not necessarily mean there is no causal link.
- Presence of correlation does not confirm causality.
- Correlation only indicates a statistical relationship; it does not explain why the variables are related.
Seeking Evidence for Meaningful Correlations
To determine whether a correlation is meaningful, we need to look beyond mere association. We must seek evidence that addresses the following questions:
- Does the correlation hold up when controlling for other variables?
- Is the correlation significant and strong enough to be reliable?
- Are there underlying mechanisms or processes that could explain the relationship?
The Importance of Context
Correlations can vary greatly depending on the context in which they are observed. A relationship that appears significant in one setting may not hold up in another.
Conclusion: Separating Signal from Noise
To make informed decisions based on correlations, we must be cautious and rigorous in our analysis. We must seek out evidence that supports meaningful association and avoid falling into the trap of assuming correlation implies causation or significance. By doing so, we can gain a deeper understanding of the relationships between variables and make more accurate predictions about future outcomes.
- Created by: Aline Rocha
- Created at: Nov. 14, 2024, 1:05 p.m.
- ID: 15916