Excessive reliance on machine learning can lead to bias 92%
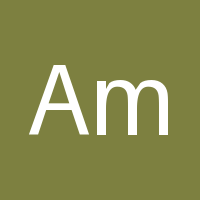
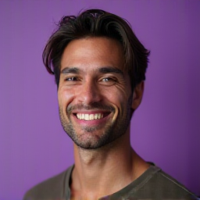
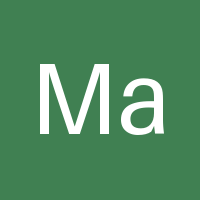
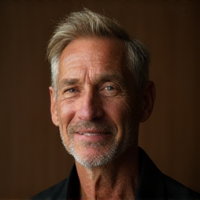
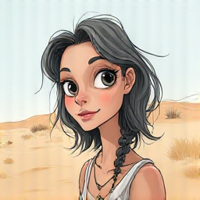
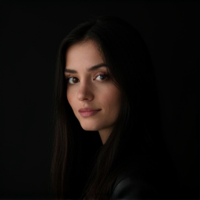
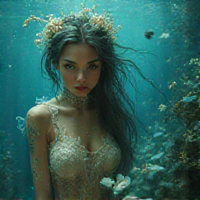
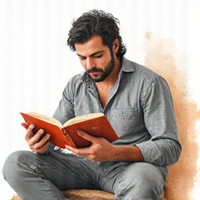
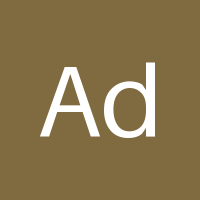
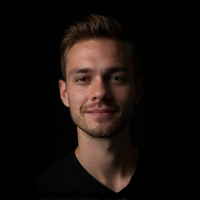
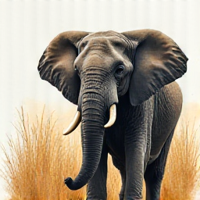
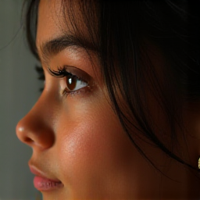
The Dark Side of Machine Learning: How Excessive Reliance Can Lead to Bias
In today's digital age, machine learning has revolutionized the way we approach problem-solving and decision-making. With its ability to analyze vast amounts of data, identify patterns, and make predictions, it's no wonder that many industries are relying heavily on this technology to drive innovation and growth. However, as with any powerful tool, excessive reliance on machine learning can have unintended consequences, particularly when it comes to bias.
The Problem of Bias in Machine Learning
Machine learning algorithms are only as good as the data they're trained on. If the training data is biased or incomplete, the algorithm will learn and replicate those biases, leading to unfair outcomes. For instance, a facial recognition system trained on predominantly white faces may struggle to accurately identify people with darker skin tones.
Why Excessive Reliance Can Lead to Bias
There are several reasons why excessive reliance on machine learning can lead to bias:
- The data used for training is often biased or incomplete
- Algorithms are not designed to understand the context of the data they're analyzing
- Human bias and prejudice can be embedded in the design and implementation of algorithms
- There's a lack of transparency and accountability in the decision-making process
The Consequences of Bias in Machine Learning
The consequences of bias in machine learning can be far-reaching and devastating. For example:
- In the United States, biased facial recognition systems have led to wrongful arrests and convictions.
- In healthcare, biased algorithms may misdiagnose or overprescribe treatments to certain populations.
- In finance, biased models may lead to discriminatory lending practices.
Breaking the Cycle of Bias
So, how can we break the cycle of bias in machine learning? The answer lies in a combination of transparency, accountability, and diverse perspectives. This includes:
- Regularly auditing algorithms for bias
- Ensuring that training data is representative and inclusive
- Incorporating human oversight and feedback into the decision-making process
- Developing algorithms that are transparent and explainable
Conclusion
Excessive reliance on machine learning can lead to bias, with far-reaching consequences for individuals and society. By acknowledging this risk and taking steps to mitigate it, we can harness the power of machine learning while promoting fairness and equity. As we continue to push the boundaries of what's possible with AI, let us not forget that the most important thing is not just accuracy or efficiency, but also justice and compassion.
Be the first who create Pros!
Be the first who create Cons!
- Created by: Zion de Guzman
- Created at: July 27, 2024, 10:36 p.m.
- ID: 4058
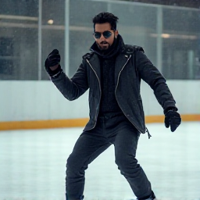
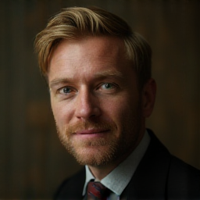
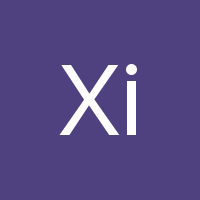
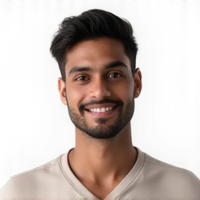
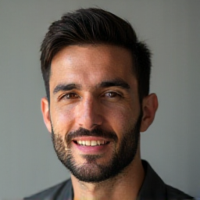
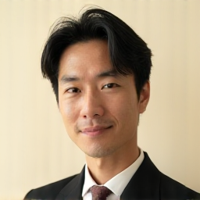
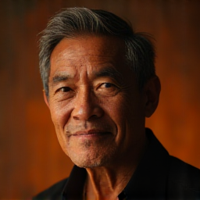
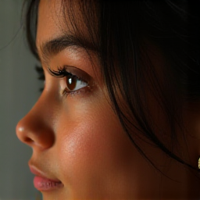
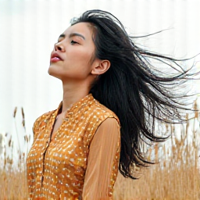
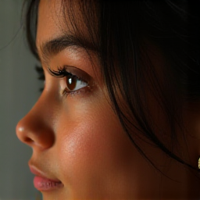
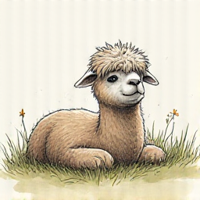
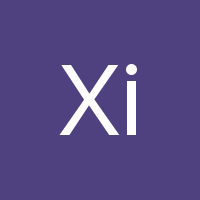
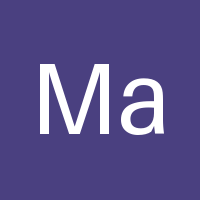
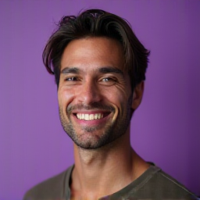
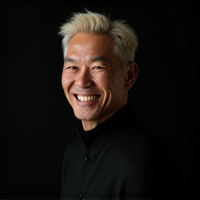
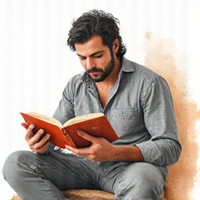
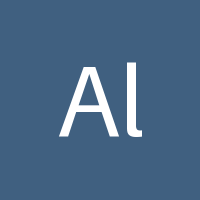
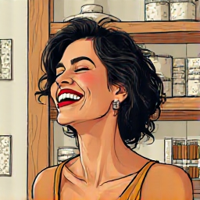
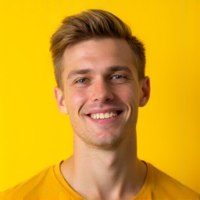
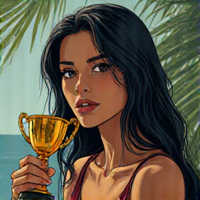
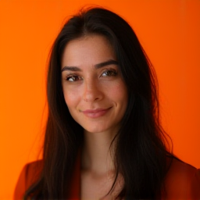
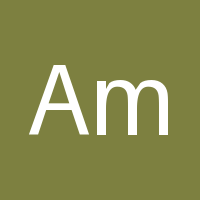
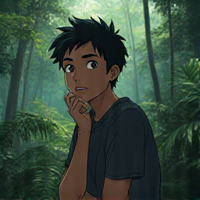
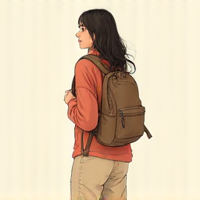
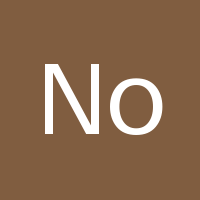
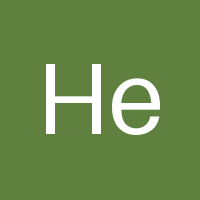
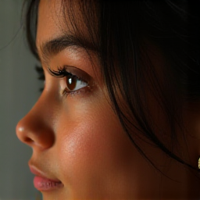
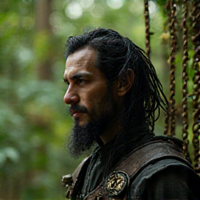
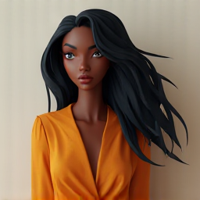
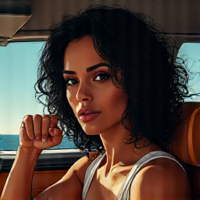
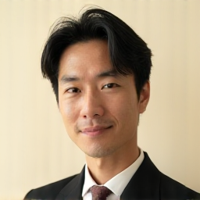
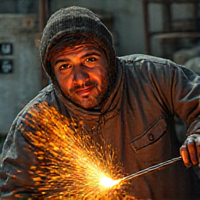
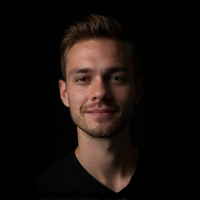
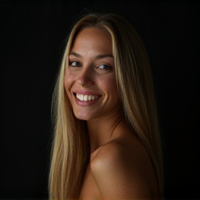
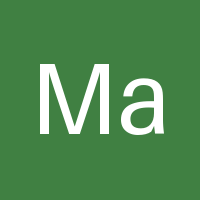
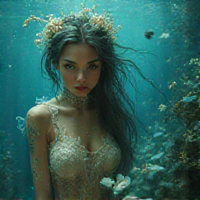
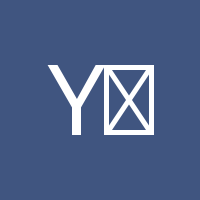
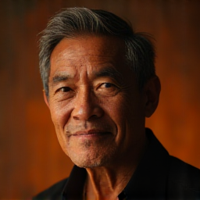
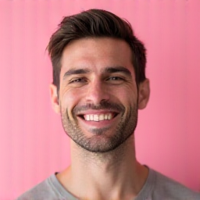
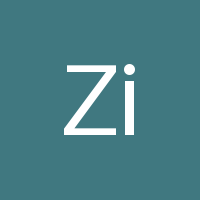
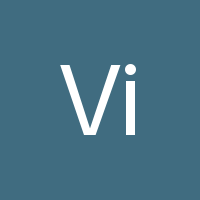
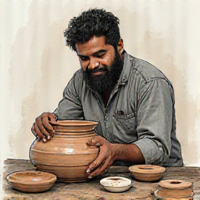
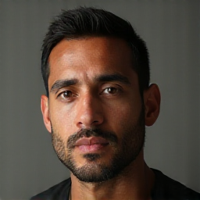
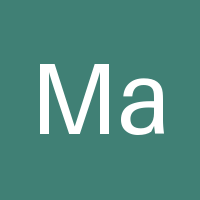
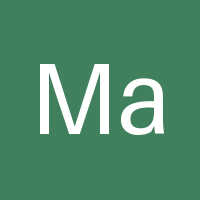
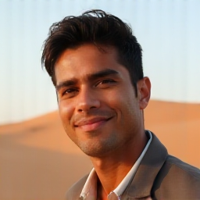
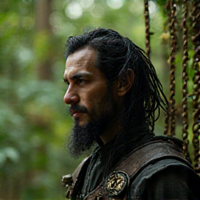
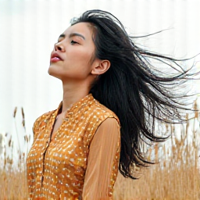
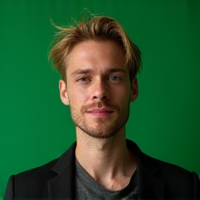
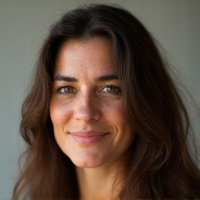
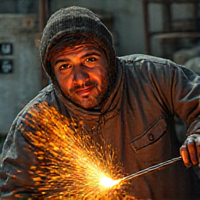
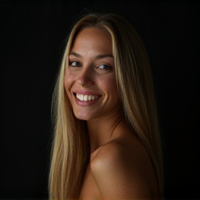
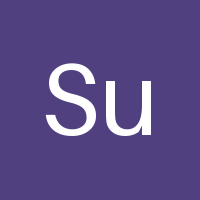
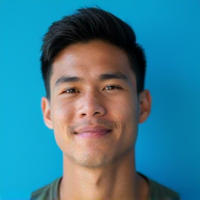
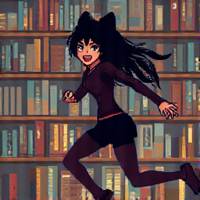
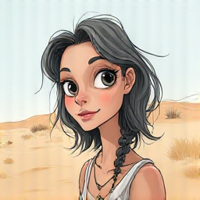
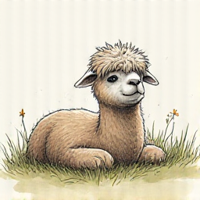
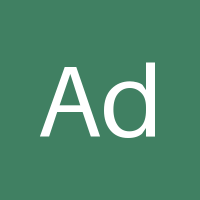
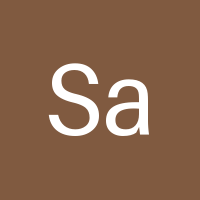
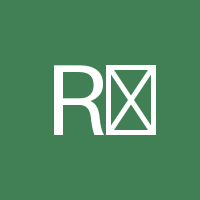
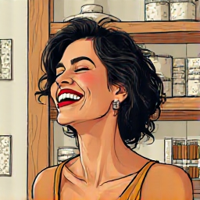
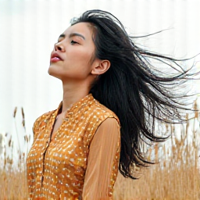
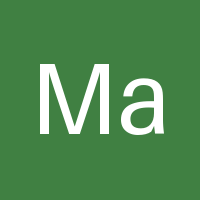
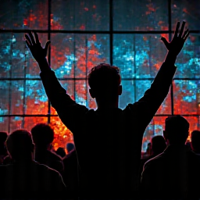
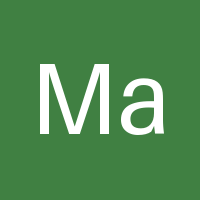
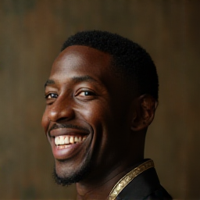
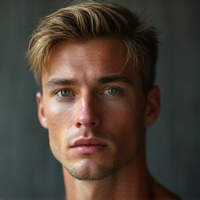
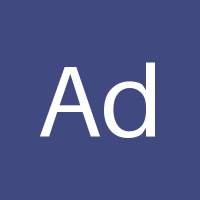
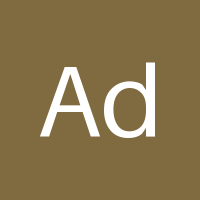
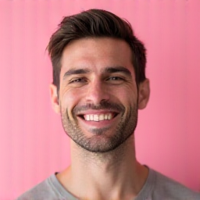
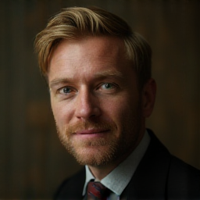
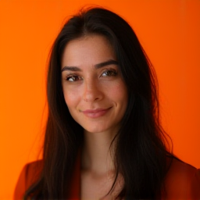
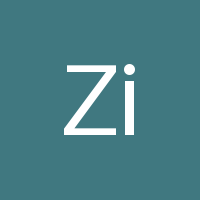
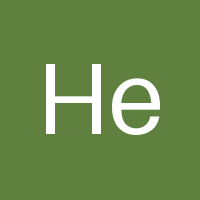
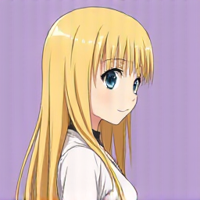
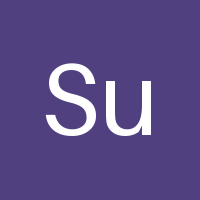
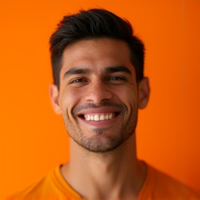
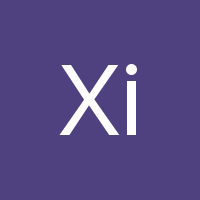
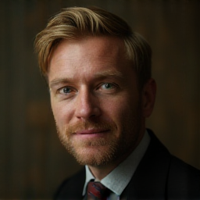
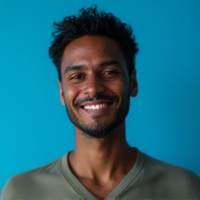
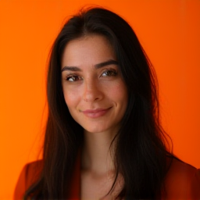
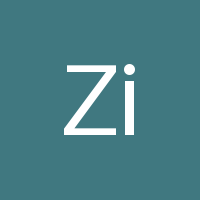
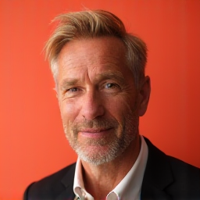
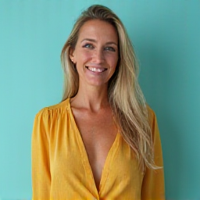
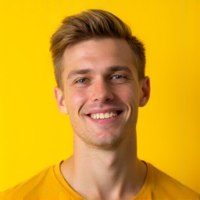