Generalization is not a primary objective during training 85%
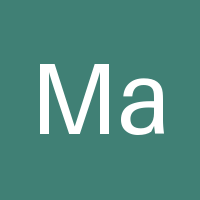
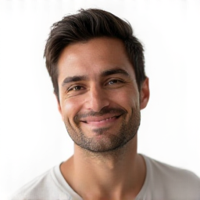
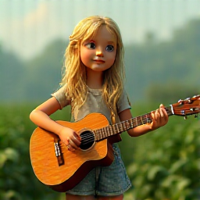
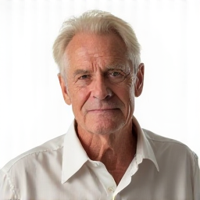
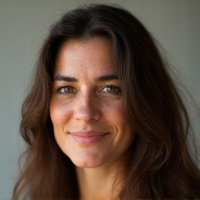
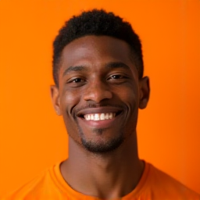
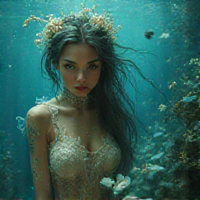
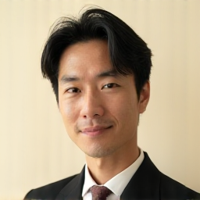
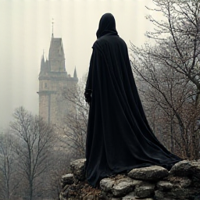
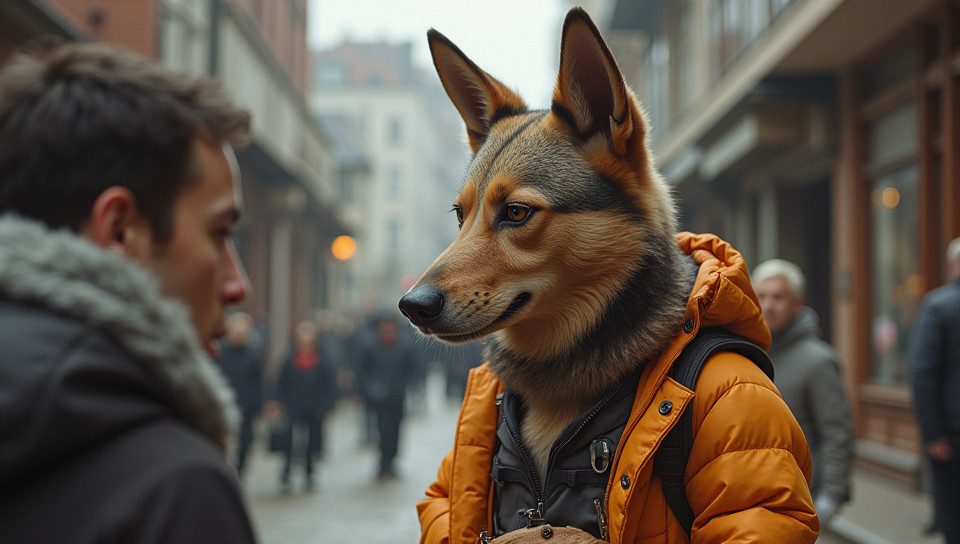
The Misconception of Generalization in Training
When it comes to machine learning, one common misconception is that the primary objective during training is to achieve generalization. However, this assumption can be detrimental to the overall performance and effectiveness of a model.
What is Generalization?
Generalization refers to the ability of a model to perform well on unseen data, beyond what was used for training. While this may seem like an essential goal, it's not always the primary focus during training.
The Primary Objective: Minimizing Loss
The main objective of training a machine learning model is to minimize the loss function, which measures the difference between the predicted output and the actual output. By minimizing the loss, the model learns to make accurate predictions on the training data.
Why Generalization is Not Always the Focus
There are several reasons why generalization may not be the primary focus during training:
- Model complexity: Overfitting can occur when a model is too complex for the training data. In this case, the model may perform well on the training data but poorly on unseen data.
- Limited data: With limited training data, it's challenging to achieve good generalization performance.
- Task-specific requirements: Depending on the task, other objectives such as precision or recall might be more important than generalization.
The Role of Validation
Validation is an essential step in machine learning that helps evaluate a model's performance on unseen data. However, validation should not be seen as a replacement for minimizing the loss during training. Instead, it should be used to monitor the model's progress and make adjustments to the hyperparameters or architecture as needed.
Conclusion
While generalization is an essential aspect of machine learning, it's not always the primary objective during training. By focusing on minimizing the loss function and using validation to evaluate performance, models can learn to make accurate predictions on unseen data. Remember, achieving good generalization performance requires careful tuning of hyperparameters, selection of appropriate architectures, and effective use of validation.
- Created by: Charles Lopez
- Created at: Feb. 17, 2025, 10:07 p.m.
- ID: 20598