Generative adversarial networks leverage two neural network components 70%
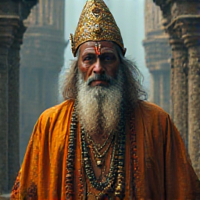
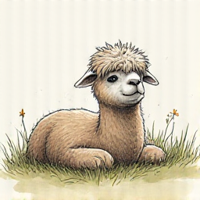
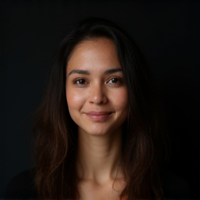
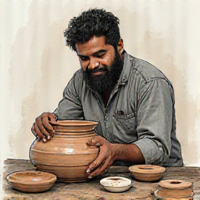
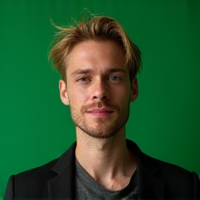
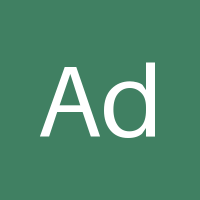
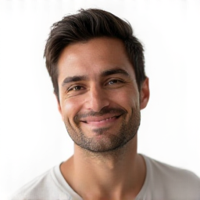
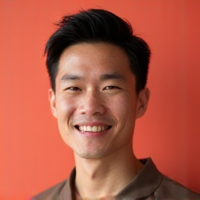
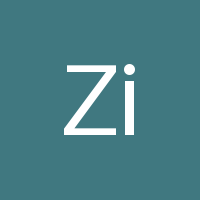
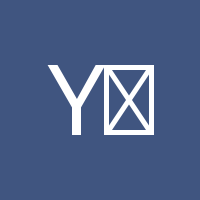
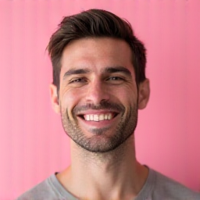
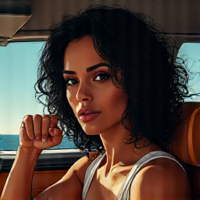
Generative Adversarial Networks: A Game-Changing Approach to Deep Learning
In recent years, Generative Adversarial Networks (GANs) have revolutionized the field of deep learning by enabling machines to learn complex patterns and generate new data samples that are indistinguishable from real ones. At the heart of this technology lie two neural network components that work together in a delicate dance of competition and cooperation.
The Building Blocks of GANs
At its core, a GAN consists of two neural networks: a Generator and a Discriminator. These two networks play opposite roles in the training process, making them adversarial in nature.
- A generator is responsible for producing new data samples that are similar to those in the training dataset.
- A discriminator, on the other hand, is tasked with distinguishing between real data samples from the training dataset and fake data samples generated by the generator.
How GANs Work
During training, the generator produces a batch of new data samples, which are then fed into the discriminator. The discriminator outputs a probability that the input sample is real or fake. This probability serves as feedback to the generator, guiding it to produce better-quality data samples. Conversely, the discriminator learns to improve its ability to distinguish between real and fake samples.
Advantages of GANs
GANs have several advantages over traditional deep learning approaches:
- Improved Data Quality: By generating new data samples that are similar to those in the training dataset, GANs can improve the diversity and quality of the available data.
- Enhanced Feature Learning: The adversarial process between the generator and discriminator encourages both networks to learn more complex features from the data.
Applications of GANs
The applications of GANs are vast and varied:
- Image Generation: GANs can generate realistic images, making them useful for applications such as image editing and manipulation.
- Data Augmentation: By generating new data samples, GANs can augment existing datasets, reducing the need for manual data collection.
Conclusion
Generative Adversarial Networks have transformed the field of deep learning by harnessing the power of two neural network components to generate realistic data samples. With their ability to improve data quality and enhance feature learning, GANs are poised to revolutionize a wide range of applications in computer vision, natural language processing, and more. As researchers continue to push the boundaries of what is possible with GANs, we can expect to see even more innovative solutions emerge in the years to come.
- Created by: Alessandro Pellegrino
- Created at: July 27, 2024, 10:55 p.m.
- ID: 4069