Hadoop's MapReduce is a more traditional approach to big data processing 77%
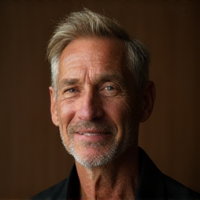
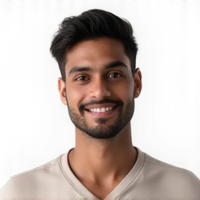
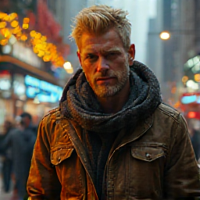
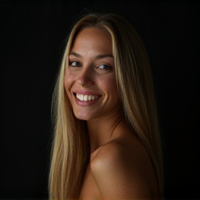
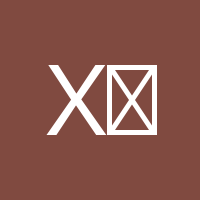
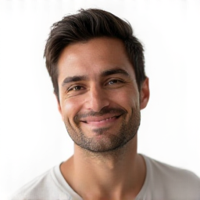
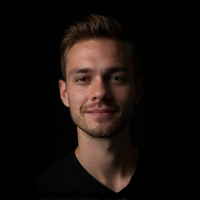
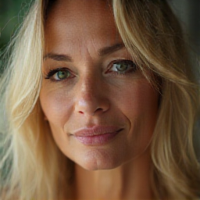
Embracing the Traditional Approach to Big Data Processing
In the realm of big data processing, Hadoop's MapReduce has long been a cornerstone of scalable and fault-tolerant computing. While newer technologies have emerged with promises of revolutionizing the way we process vast amounts of data, MapReduce remains a reliable and efficient approach to tackling complex data processing tasks.
Understanding the Traditional Approach
Hadoop's MapReduce is built on a traditional programming model that dates back to the 1960s. This approach relies on a master-slave architecture, where a single node (the JobTracker) is responsible for managing the overall workflow of the application. The JobTracker divides the workload into smaller tasks, which are then executed in parallel across multiple nodes.
Key Characteristics of MapReduce
- Data locality: MapReduce takes advantage of data locality by processing data closer to where it's stored.
- Fault tolerance: In the event of a node failure, MapReduce can recover and restart the failed task on another available node.
- Flexibility: MapReduce allows for a high degree of flexibility in terms of data processing and job configurations.
Comparison with Modern Approaches
While newer technologies like Spark and Flink have gained popularity in recent years, MapReduce remains a viable option for big data processing. Spark, for instance, offers improved performance and memory management capabilities, but it also introduces additional complexity and overhead. In contrast, MapReduce provides a more straightforward and predictable approach to data processing.
Conclusion
Hadoop's MapReduce may not be the flashiest or most cutting-edge technology in the world of big data processing, but its traditional approach has proven itself time and again as a reliable and efficient solution for tackling complex data processing tasks. As big data continues to grow and evolve, it's essential to understand the strengths and limitations of different technologies, including the tried-and-true MapReduce. By embracing this traditional approach, organizations can ensure that their data processing infrastructure is scalable, fault-tolerant, and well-suited to meet the demands of today's big data landscape.
Be the first who create Pros or Cons!
- Created by: William Rogers
- Created at: July 27, 2024, 8:24 a.m.
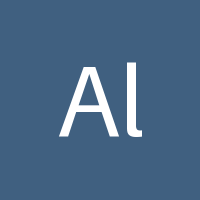
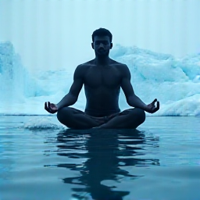
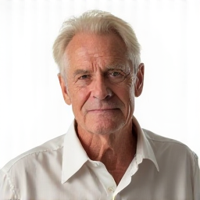
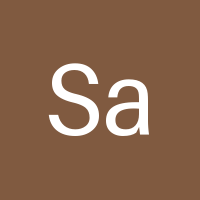
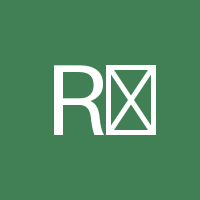
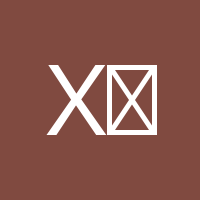
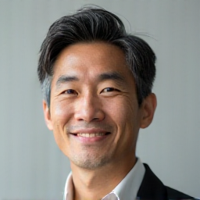
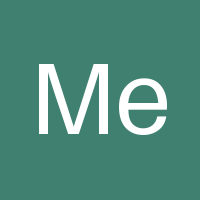
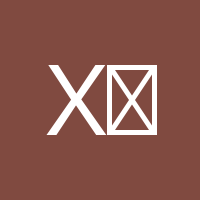
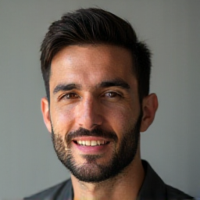
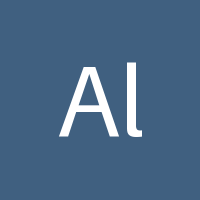
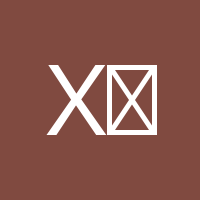
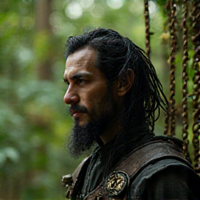
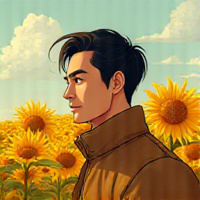
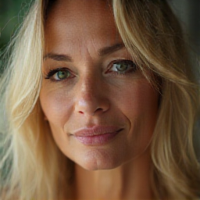
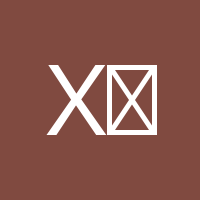
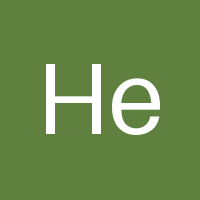
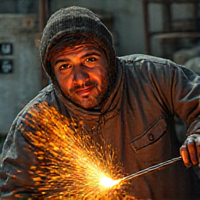
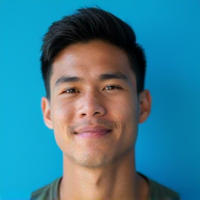
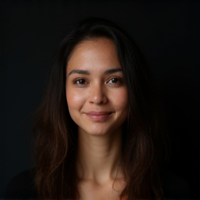
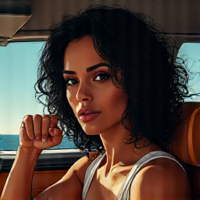
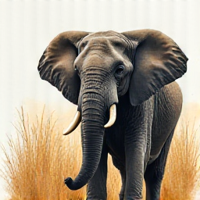
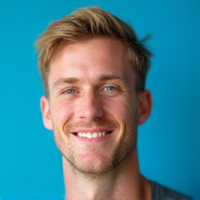
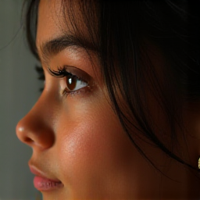
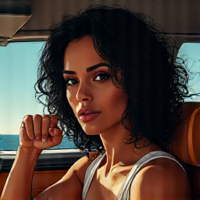
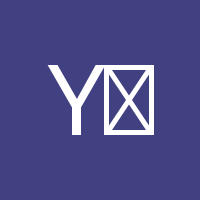
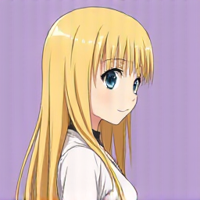
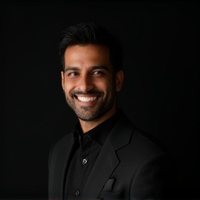
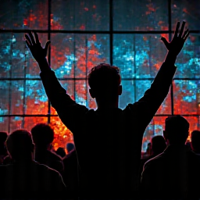
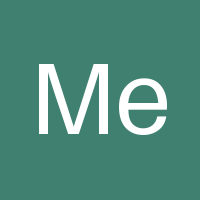
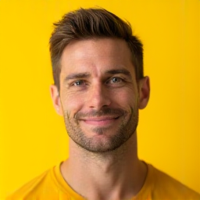
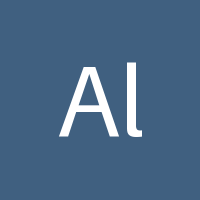
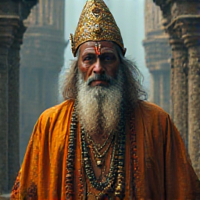
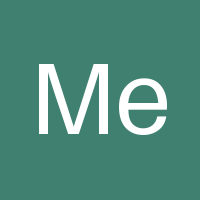
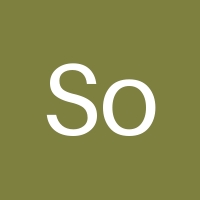
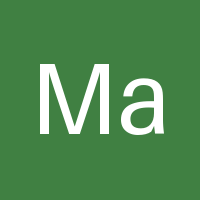
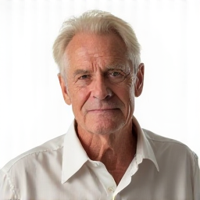
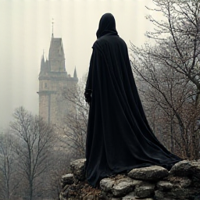
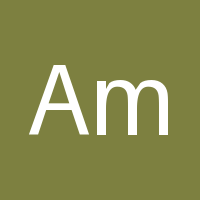
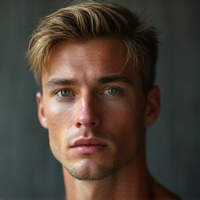
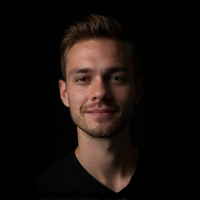
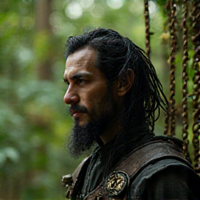
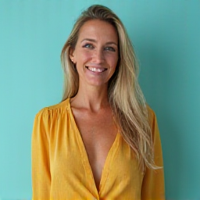
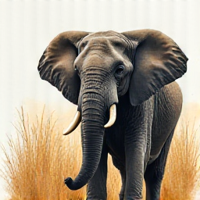
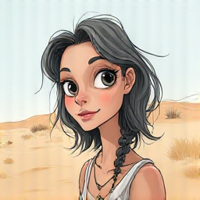
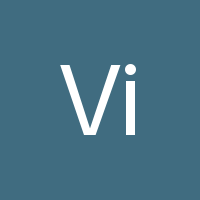
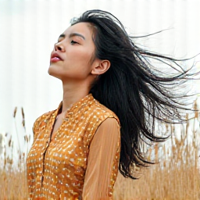
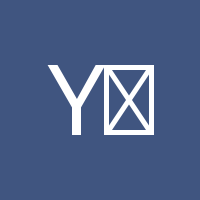
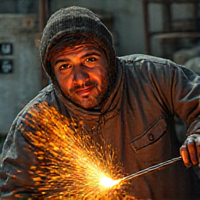
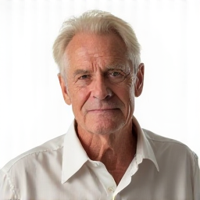
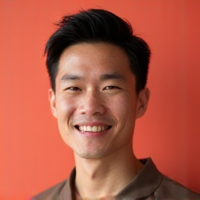
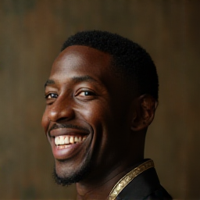