Hand-coded rules fail to handle ambiguity in big data 93%
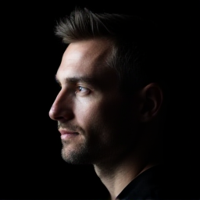
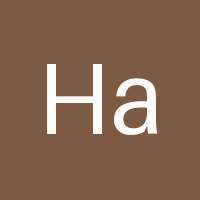
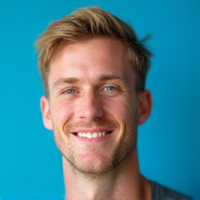
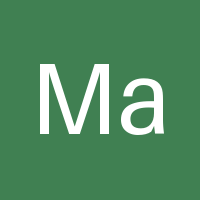
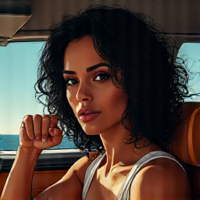
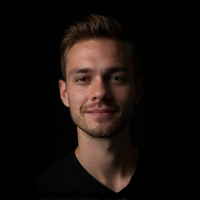
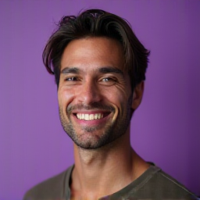
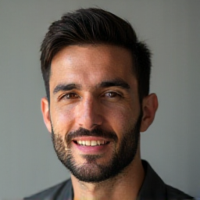
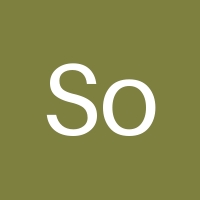
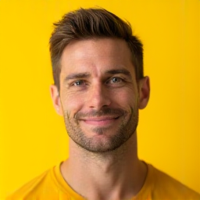
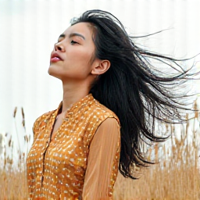
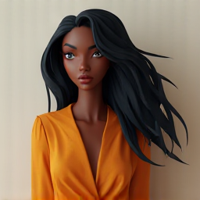
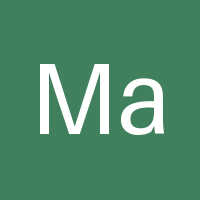
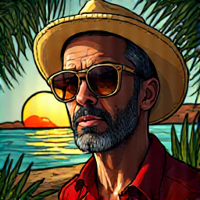
The Limits of Hand-Coded Rules: Why They Struggle to Handle Ambiguity in Big Data
In today's data-driven world, organizations are drowning in a sea of information. With the rise of big data, businesses are increasingly relying on complex systems and algorithms to make sense of it all. However, one approach that has been widely adopted – hand-coded rules – is often found wanting when it comes to handling ambiguity.
The Problem with Hand-Coded Rules
Hand-coded rules involve writing specific, detailed instructions that tell a system how to handle different scenarios. While this approach may seem straightforward, it quickly becomes apparent that it's not equipped to deal with the nuances of big data. Here are some reasons why:
- Data is inherently complex and messy
- There are often multiple interpretations for a single piece of information
- New data points can create ambiguity where none existed before
The Consequences of Ambiguity
When hand-coded rules fail to handle ambiguity, it can have serious consequences for an organization. Some of these include:
- Inaccurate or inconsistent results
- Increased costs associated with manual intervention and correction
- Damage to reputation due to poor decision-making
- Lost opportunities for growth and innovation
Alternative Approaches
So what's the solution? Fortunately, there are alternative approaches that can help organizations better navigate the complexities of big data. Some of these include:
- Machine learning algorithms that can learn from data and adapt to new scenarios
- Natural language processing (NLP) techniques that can handle ambiguity in unstructured data
- Hybrid systems that combine rule-based and machine-learning approaches
Conclusion
Hand-coded rules are a relic of the past when it comes to handling big data. Their limitations have been exposed, and it's time for organizations to move on to more sophisticated solutions. By embracing alternative approaches, businesses can unlock the full potential of their data and make better decisions that drive growth and success.
- Created by: Sofia David
- Created at: July 27, 2024, 6:32 a.m.
- ID: 3858