Handling massive datasets demands efficient processing algorithms 73%
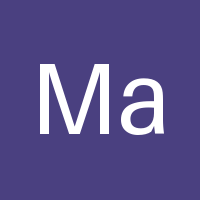
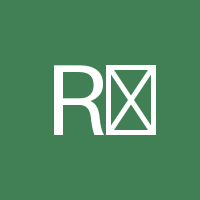
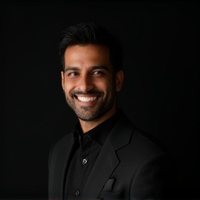
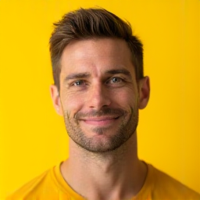
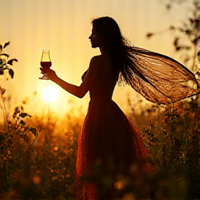
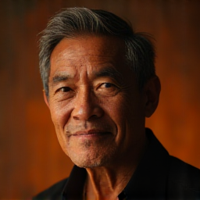
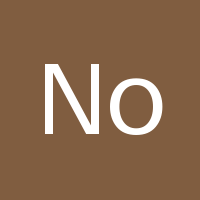
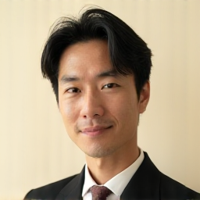
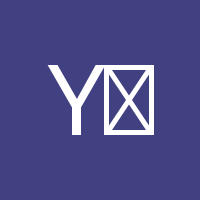
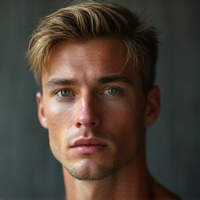
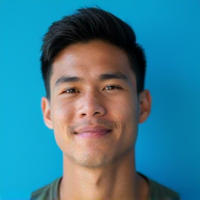
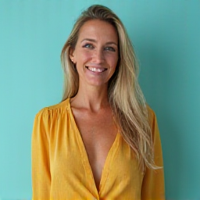
Handling Massive Datasets Demands Efficient Processing Algorithms
In today's data-driven world, organizations are generating vast amounts of data every minute. This explosion in data has created new opportunities for businesses to gain insights and make informed decisions. However, handling massive datasets is a daunting task that requires efficient processing algorithms to extract valuable information from the sea of data.
The Challenges of Handling Massive Datasets
- Data volume: The sheer size of the dataset can be overwhelming, making it difficult to store, manage, and process.
- Data velocity: The rapid generation of new data requires real-time processing capabilities to keep up with the pace.
- Data variety: The diversity of data types, formats, and structures demands flexibility in processing algorithms.
Types of Efficient Processing Algorithms
Efficient processing algorithms can be broadly categorized into two types:
1. Parallel Processing Algorithms
These algorithms divide the dataset into smaller chunks and process them simultaneously across multiple processors or nodes. This approach speeds up processing times and improves overall efficiency.
2. Distributed Processing Algorithms
These algorithms distribute the data across multiple machines, allowing for parallel processing and improving scalability. This approach is particularly useful when dealing with large datasets that don't fit into a single machine's memory.
Choosing the Right Algorithm
Choosing the right algorithm depends on several factors, including:
- Data characteristics: Understanding the type of data, its volume, and velocity helps in selecting the most suitable algorithm.
- Processing power: The number of processors or nodes available determines the level of parallel processing that can be achieved.
- Memory constraints: Limited memory requires algorithms that are designed to handle sparse data or use disk-based storage.
Conclusion
Handling massive datasets demands efficient processing algorithms that can handle high volumes, velocities, and varieties of data. By choosing the right algorithm and understanding its limitations, organizations can unlock valuable insights from their data and make informed decisions. As data continues to grow exponentially, it's essential to invest in efficient processing technologies to stay ahead of the curve.
- Created by: Ren Ōta
- Created at: July 27, 2024, 8:16 a.m.
- ID: 3917