Human annotation is essential for effective supervised learning 90%
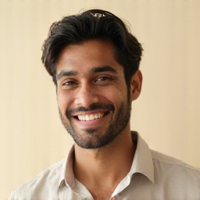
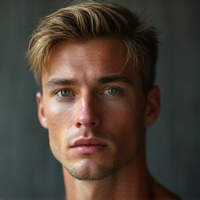
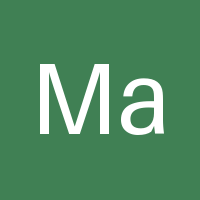
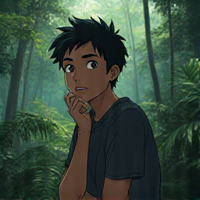
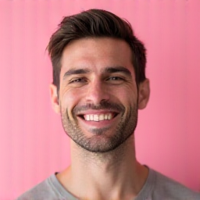
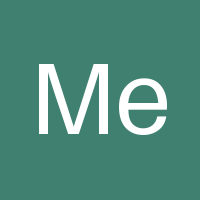
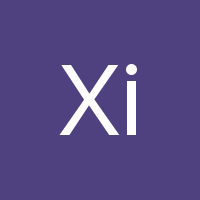
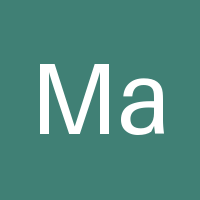
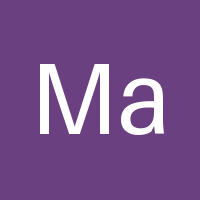
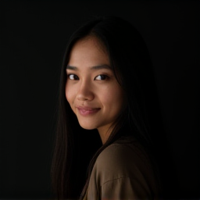
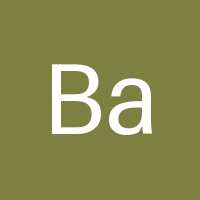
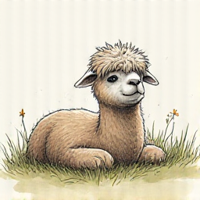
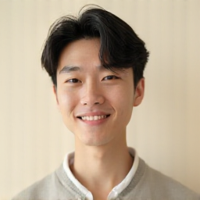
Human Annotation: The Key to Unlocking Effective Supervised Learning
As artificial intelligence continues to revolutionize industries and transform the way we live, one crucial component of its success often goes overlooked: human annotation. Behind every well-trained machine learning model lies a sea of annotated data, carefully curated by humans to provide the insights needed for effective supervised learning.
The Importance of High-Quality Training Data
High-quality training data is the backbone of any successful machine learning project. It's what enables models to learn from patterns and relationships in the data, making predictions and decisions with increasing accuracy. But how do we ensure that our training data is accurate, relevant, and comprehensive? That's where human annotation comes in.
The Role of Human Annotation
Human annotation involves labeling and categorizing data to provide context and meaning. It requires a deep understanding of the problem domain, as well as attention to detail and consistency. By annotating data, humans can:
- Identify patterns and relationships that may be difficult for machines to detect
- Ensure accuracy and relevance in the training data
- Provide diverse perspectives and insights that can improve model performance
- Develop high-quality datasets that are representative of real-world scenarios
The Impact on Model Performance
The quality of human annotation directly impacts the performance of machine learning models. When data is accurately annotated, models learn more effectively and make better predictions. Conversely, poor or inconsistent annotation can lead to model drift, where the model becomes less accurate over time.
Conclusion
Human annotation is a critical component of effective supervised learning. By investing in high-quality human annotation, we can develop machine learning models that are accurate, reliable, and scalable. As AI continues to shape our world, it's essential that we prioritize human annotation as an integral part of the development process. Only then can we unlock the full potential of machine learning and reap its many benefits.
- Created by: Maria Reed
- Created at: July 27, 2024, 11:25 p.m.
- ID: 4084