In-memory computing approaches like Apache Ignite can process big data quickly 100%
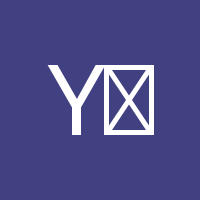
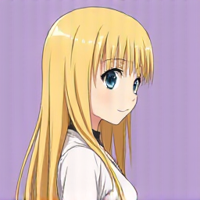
The Future of Big Data Processing: In-Memory Computing Approaches
Imagine being able to process massive amounts of data in real-time, making it possible to make informed decisions faster than ever before. This is the promise of in-memory computing approaches like Apache Ignite.
What are In-Memory Computing Approaches?
In-memory computing involves storing and processing large amounts of data directly within a computer's RAM (random access memory), rather than relying on slower storage devices like hard drives or solid-state drives. This allows for faster data retrieval, analysis, and processing times.
Benefits of In-Memory Computing
- Improved performance: In-memory computing can process large datasets in seconds or minutes, compared to hours or days using traditional disk-based systems.
- Enhanced scalability: By leveraging RAM as a shared resource, in-memory computing can handle massive amounts of data without sacrificing performance.
- Simplified architecture: In-memory computing approaches like Apache Ignite often require less hardware and fewer complex configurations, making them easier to deploy and manage.
How Does Apache Ignite Work?
Apache Ignite is an open-source in-memory computing platform that enables the development of high-performance applications. It provides a distributed caching layer, which allows for data to be stored across multiple nodes in a cluster. This architecture enables real-time processing, improved scalability, and reduced latency.
Real-World Applications
In-memory computing approaches like Apache Ignite are being used in various industries, including finance, healthcare, and e-commerce, to:
- Process high-frequency trading data
- Analyze large medical imaging datasets
- Power online shopping platforms with real-time product recommendations
Conclusion
The power of in-memory computing approaches like Apache Ignite lies in their ability to process big data quickly and efficiently. By leveraging the vast potential of RAM, organizations can make faster decisions, improve customer experiences, and stay ahead of the competition. As we continue to generate ever-larger amounts of data, the importance of in-memory computing will only grow, making it an essential tool for businesses looking to drive innovation and growth.
Be the first who create Pros!
Be the first who create Cons!
- Created by: Yǔtóng Suen
- Created at: July 27, 2024, 8:37 a.m.
- ID: 3929
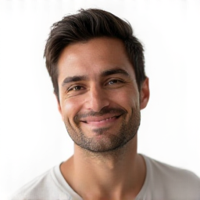
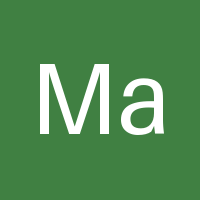
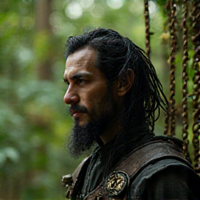
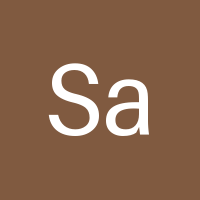
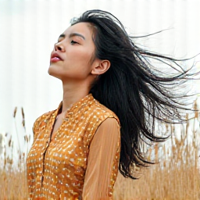
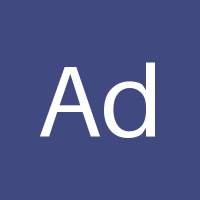
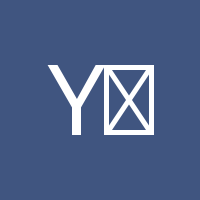
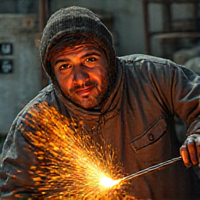
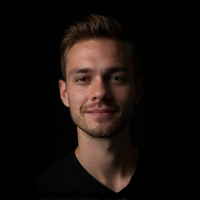
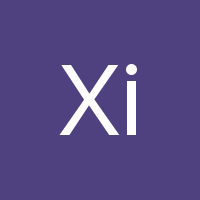
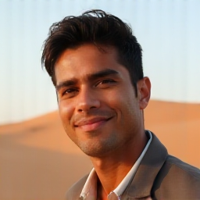
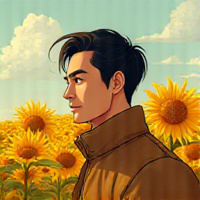
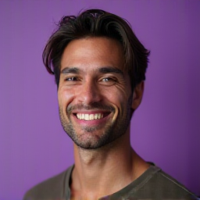
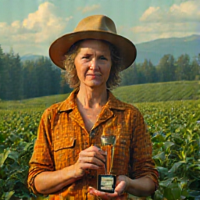
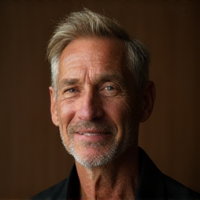
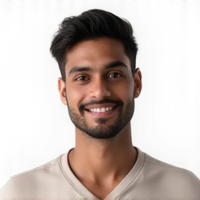
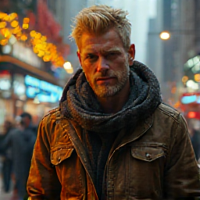
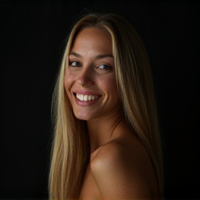
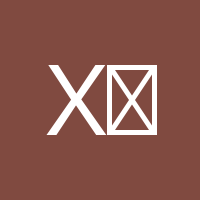
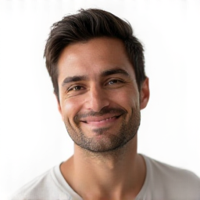
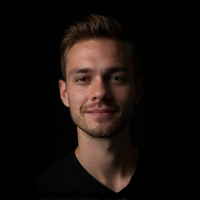
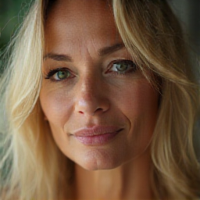
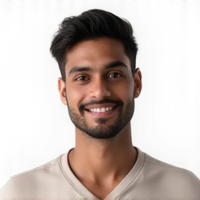
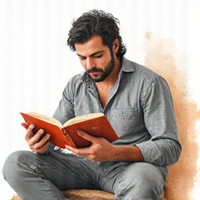
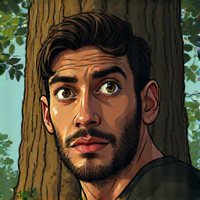
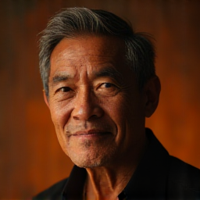
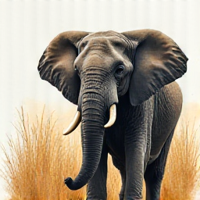
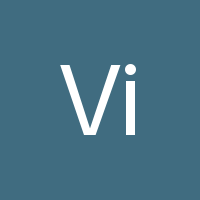
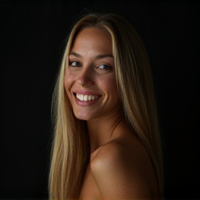
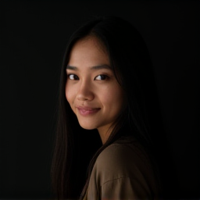
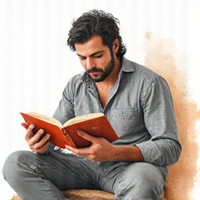
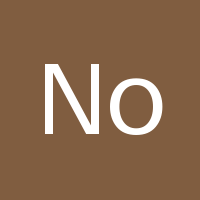
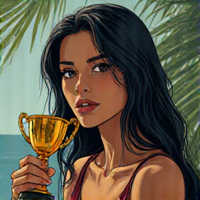
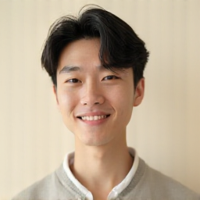
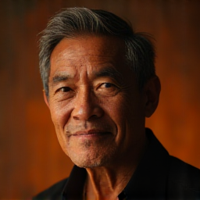
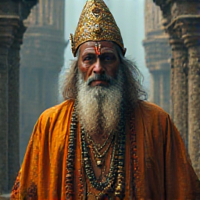
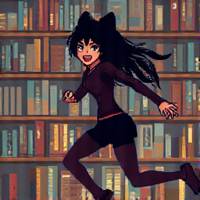
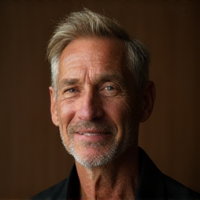
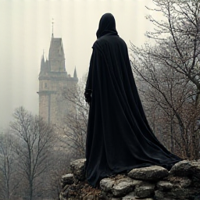
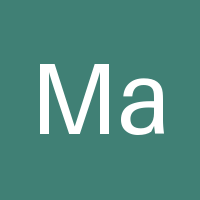
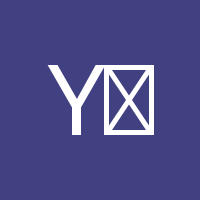
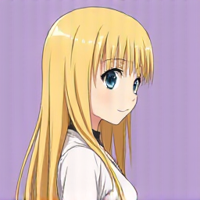
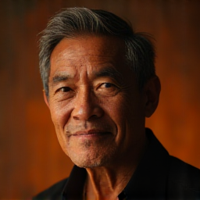
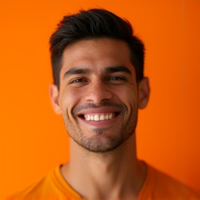
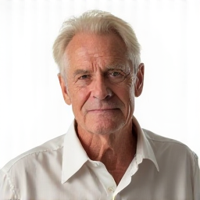
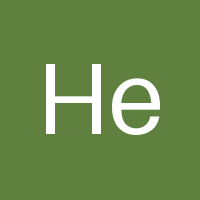
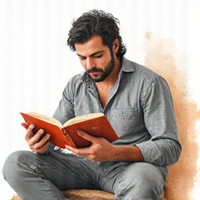
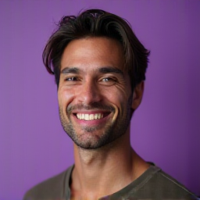
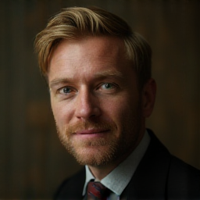
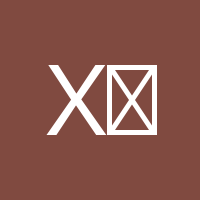
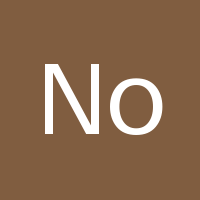
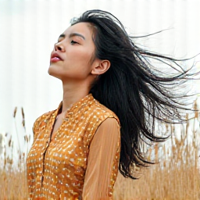
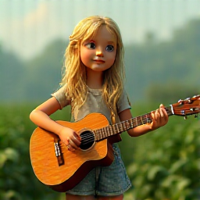
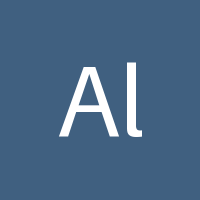
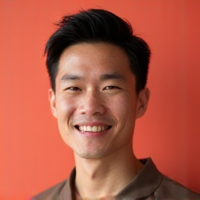
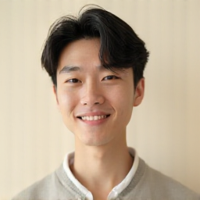
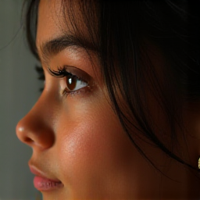
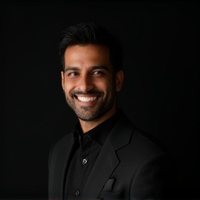
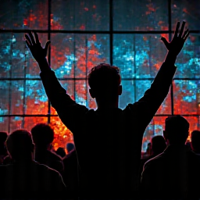
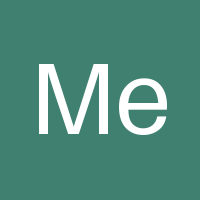
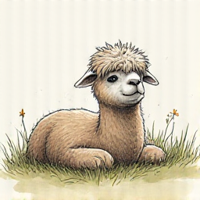
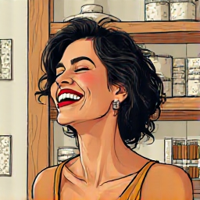
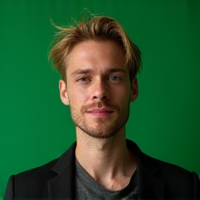
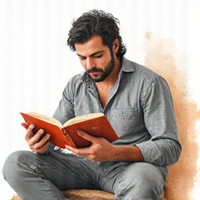
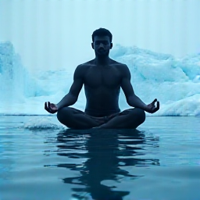
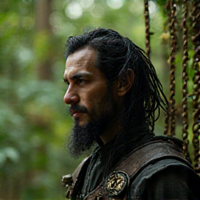
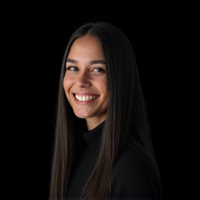
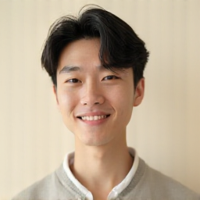
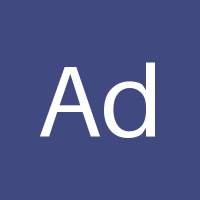
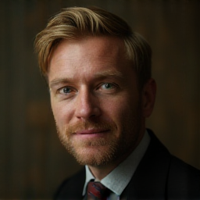
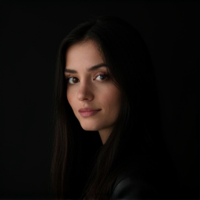
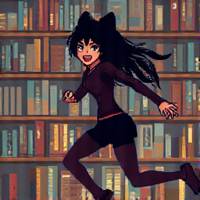
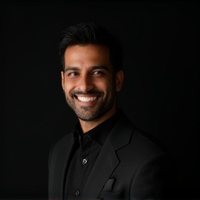
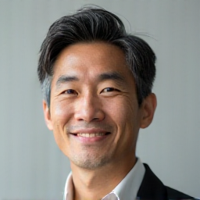
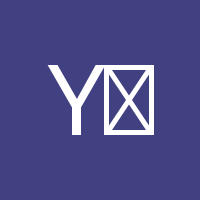
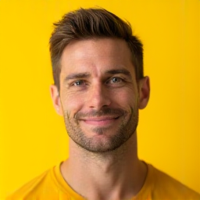