Insufficient computational resources slow down big data processing 67%
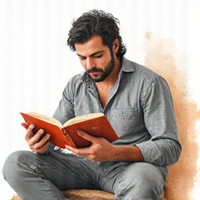
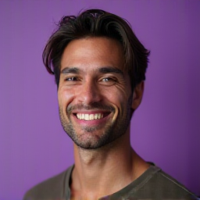
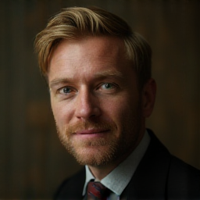
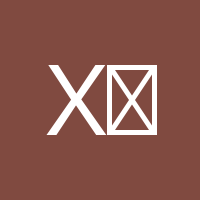
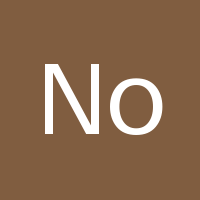
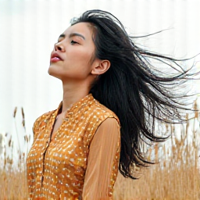
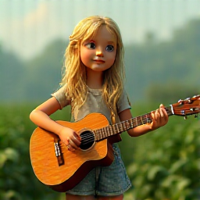
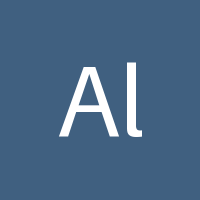
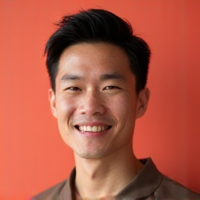
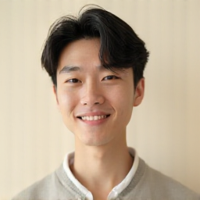
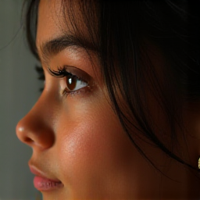
Insufficient Computational Resources Slow Down Big Data Processing
As we continue to generate and collect vast amounts of data, our ability to process and analyze this information is becoming increasingly crucial for businesses, organizations, and individuals alike. However, one major roadblock stands in the way: insufficient computational resources.
The Challenges of Big Data
Big data processing requires a tremendous amount of computing power, memory, and storage capacity. With large datasets growing exponentially, traditional hardware and software infrastructure are often unable to keep up with the demands placed upon them. This can lead to slow processing times, errors, and even system crashes.
Causes of Insufficient Computational Resources
There are several reasons why computational resources may be insufficient for big data processing:
- Outdated hardware and software
- Limited memory and storage capacity
- Inadequate network infrastructure
- Poorly optimized algorithms and workflows
- Lack of expertise in managing large-scale computing systems
The Consequences of Insufficient Computational Resources
The consequences of insufficient computational resources can be severe. They include:
- Delayed insights and decision-making
- Decreased productivity and efficiency
- Increased costs due to manual intervention and troubleshooting
- Reduced accuracy and reliability of results
- Missed opportunities for innovation and growth
Solutions to the Problem
To address the issue of insufficient computational resources, consider the following strategies:
- Invest in modern hardware and software infrastructure that is optimized for big data processing
- Implement cloud-based computing solutions to scale up or down as needed
- Optimize algorithms and workflows for maximum efficiency
- Develop expertise in managing large-scale computing systems
- Leverage distributed computing techniques to harness collective processing power
Conclusion
Insufficient computational resources are a major obstacle to big data processing, but it is not an insurmountable one. By understanding the causes of the problem, recognizing its consequences, and implementing effective solutions, we can unlock the full potential of big data analysis and drive business success. As the demands on our computing infrastructure continue to grow, it's essential that we prioritize investment in modern hardware, software, and expertise to stay ahead of the curve.
- Created by: Mùchén Chu
- Created at: July 27, 2024, 9:54 a.m.
- ID: 3970