Insufficient data cleansing processes compromise maintenance predictions 94%
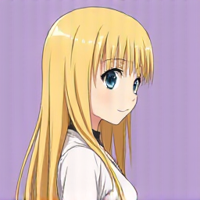
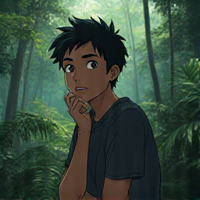
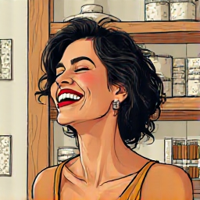
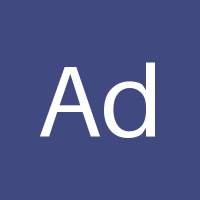
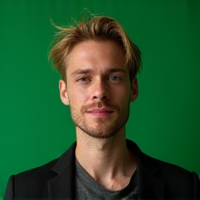
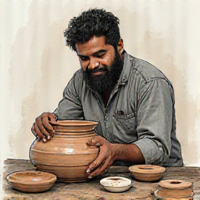
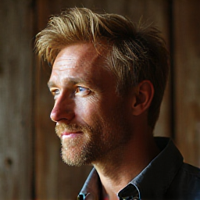
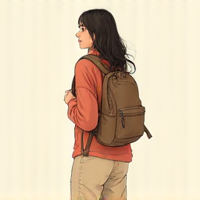
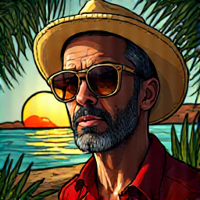
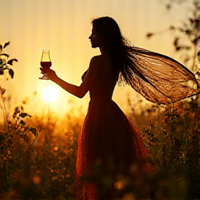
Predictive Maintenance: The Hidden Dangers of Insufficient Data Cleansing
Imagine being responsible for ensuring the smooth operation of critical infrastructure, such as power plants or transportation systems, where even a slight delay can have catastrophic consequences. To prevent breakdowns and optimize performance, maintenance teams rely on predictive analytics to forecast when equipment is likely to fail. However, if data cleansing processes are inadequate, these predictions may be nothing more than wishful thinking.
The Importance of Data Quality
Data quality is crucial for accurate predictive modeling. In the context of maintenance prediction, data includes various types of information such as sensor readings, historical failure records, and environmental factors. If this data is contaminated with errors or inconsistencies, it can lead to flawed predictions that may result in unnecessary downtime or even catastrophic failures.
Common Data Issues
- Missing values
- Duplicates
- Inconsistent formatting
- Outliers and anomalies
- Inaccurate or outdated information
These issues can be caused by a variety of factors, including human error, equipment malfunctions, or data integration problems. If left unaddressed, they can compromise the integrity of the entire dataset.
The Consequences of Insufficient Data Cleansing
Insufficient data cleansing processes can have far-reaching consequences for maintenance teams. Some potential risks include:
- Overlooking critical issues that may lead to equipment failure
- Wasting resources on unnecessary repairs or replacements
- Missing opportunities for proactive maintenance and cost savings
- Eroding trust in predictive analytics and decision-making processes
The Path Forward
To mitigate these risks, it is essential to prioritize data cleansing as a critical step in the predictive modeling process. This involves implementing robust data quality controls, regular data validation, and thorough error handling procedures. By doing so, maintenance teams can ensure that their predictions are based on reliable and accurate data, ultimately leading to safer, more efficient, and cost-effective operations.
Conclusion
In conclusion, insufficient data cleansing processes can have devastating consequences for maintenance predictions. By acknowledging the importance of data quality and implementing robust data cleansing strategies, maintenance teams can unlock the full potential of predictive analytics and ensure that their equipment runs smoothly, efficiently, and safely. The stakes are high, but with a focus on data integrity, we can build a more reliable and resilient future for critical infrastructure and beyond.
- Created by: Elif Ă–zdemir
- Created at: July 27, 2024, 9:43 a.m.
- ID: 3964