K-means clustering groups similar unlabeled data points together 83%
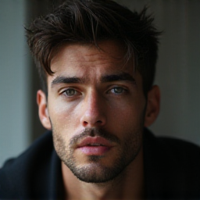
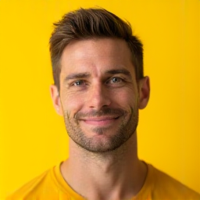
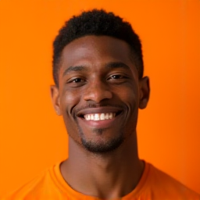
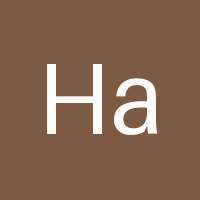
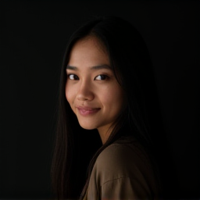
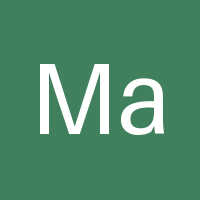
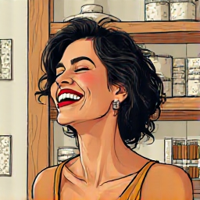
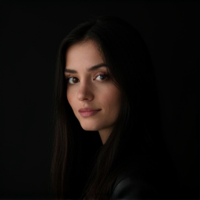
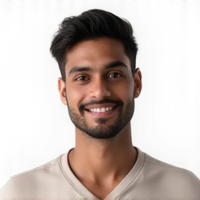
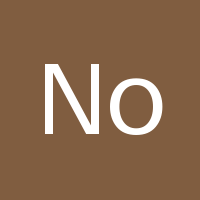
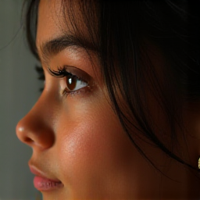
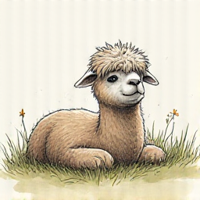
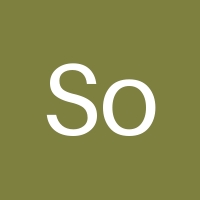
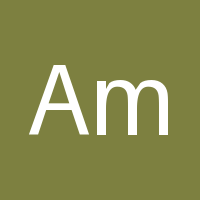
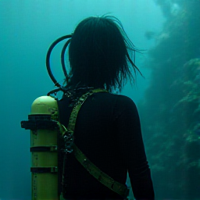
K-means Clustering: Grouping Similar Data Points Together
Imagine being able to categorize customers based on their purchasing behavior, or identify patterns in customer complaints to improve product quality. K-means clustering is a powerful unsupervised machine learning algorithm that enables us to group similar data points together. In this article, we'll explore how k-means clustering works and its applications.
Understanding K-means Clustering
K-means clustering is a type of centroid-based clustering algorithm. It's an iterative process that groups similar data points into clusters based on their features or attributes. The goal is to minimize the variance within each cluster while maximizing the distance between clusters.
Key Steps in K-means Clustering
- Assign initial centroids randomly
- Calculate distances from each data point to the closest centroid
- Update cluster assignments for each data point
- Recalculate new centroids as the mean of all points assigned to a cluster
- Repeat steps 2-4 until convergence or maximum iterations reached
Choosing the Optimal Number of Clusters (K)
One of the key challenges in k-means clustering is choosing the optimal number of clusters. This can be done using various methods such as the elbow method, silhouette analysis, or by visually inspecting the cluster density plot.
Applications of K-means Clustering
- Customer segmentation: Identify distinct customer groups based on their purchasing behavior and demographics.
- Image processing: Group similar pixels together to segment images into meaningful regions.
- Gene expression analysis: Cluster genes with similar expression patterns across different conditions.
- Quality control: Identify outliers or anomalies in manufacturing processes.
Conclusion
K-means clustering is a versatile algorithm that can be applied to various domains. By understanding how it works and its applications, you'll be able to unlock valuable insights from your data. Whether you're working on customer segmentation, image processing, or quality control, k-means clustering is an essential tool in your data analysis toolkit.
Be the first who create Pros or Cons!
- Created by: Evelyn Perez
- Created at: July 28, 2024, 12:03 a.m.
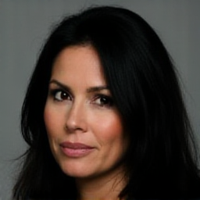
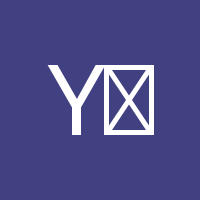
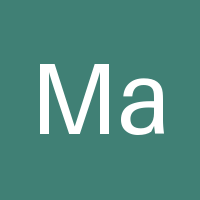
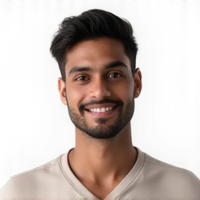
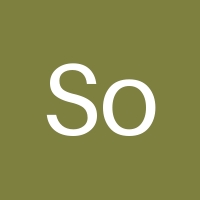
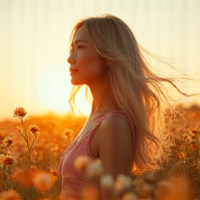
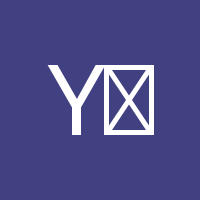
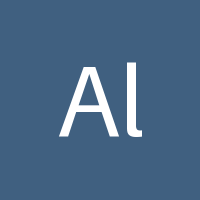
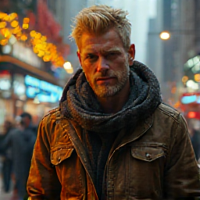
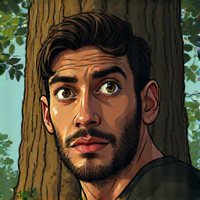
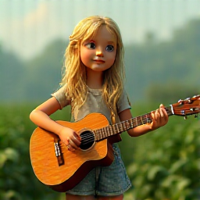
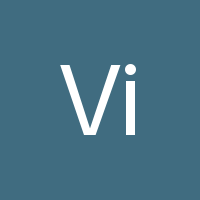
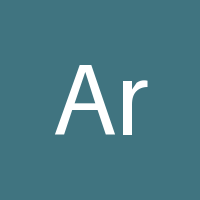
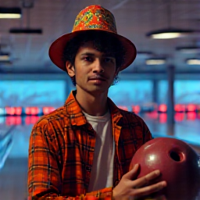
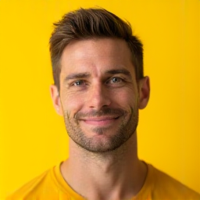
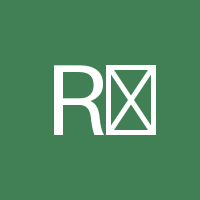
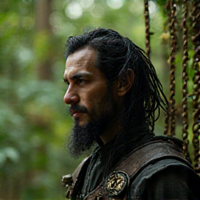
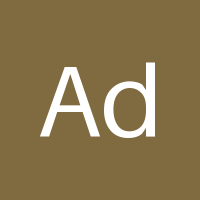
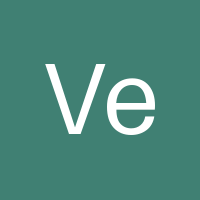
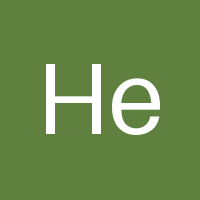
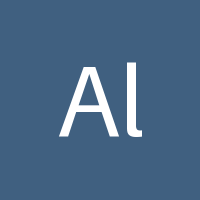
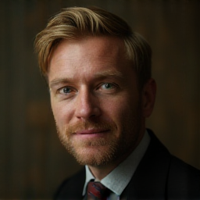
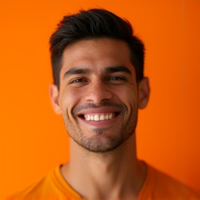
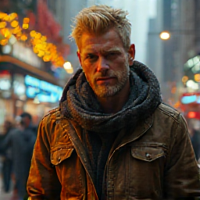
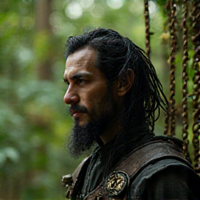
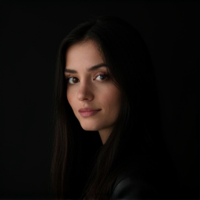
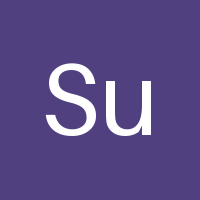
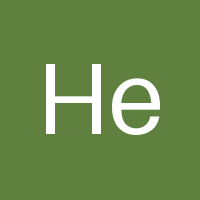
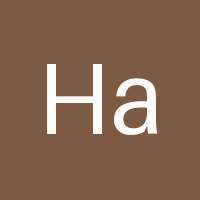
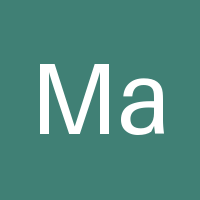
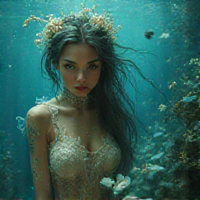
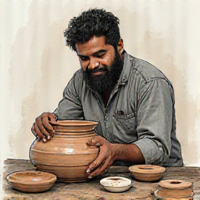
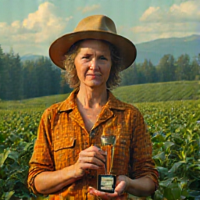
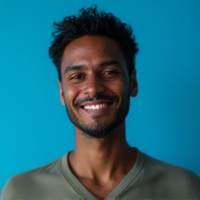
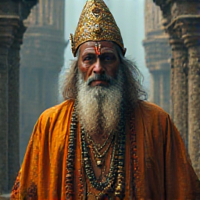
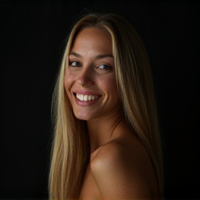
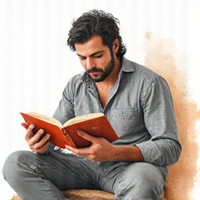
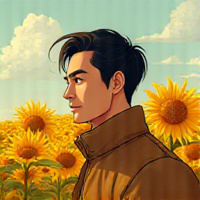
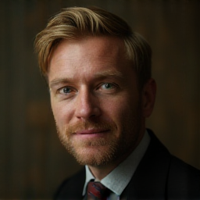
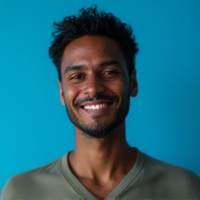
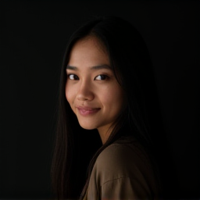
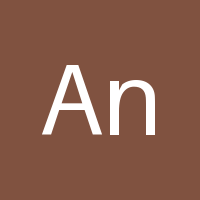
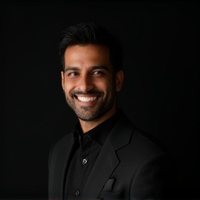
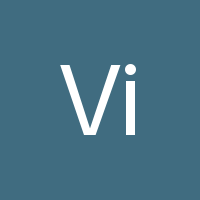
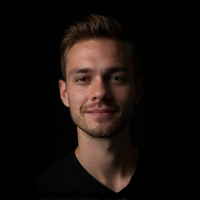
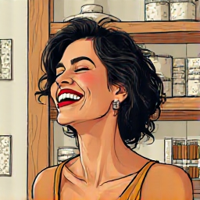
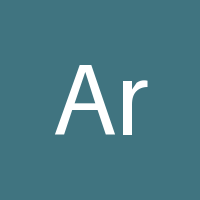
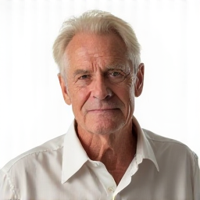