Labeled data enables accurate model performance in supervised learning 83%
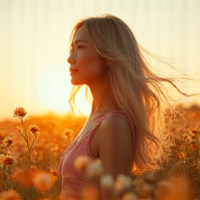
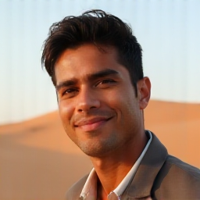
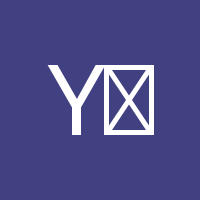
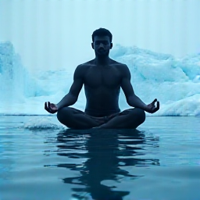
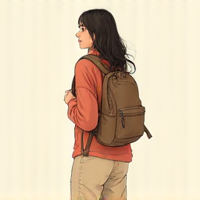
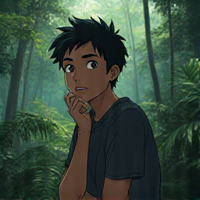
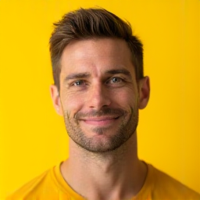
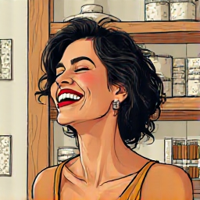
Labeled Data: The Key to Unlocking Accurate Model Performance
In the world of machine learning, data is king. But it's not just about having access to large amounts of data; it's also about ensuring that the data is accurate and relevant to the task at hand. In supervised learning, which involves training models on labeled data to make predictions or take actions, high-quality labels are essential for achieving accurate model performance.
Why Labeled Data Matters
Labeled data is a crucial component in supervised learning because it allows models to learn from examples of correct outputs. When a dataset has accurate and relevant labels, the model can use this information to improve its prediction accuracy over time. In contrast, working with unlabeled or poorly labeled data can lead to poor model performance, which can have serious consequences in applications such as medical diagnosis, autonomous vehicles, or financial forecasting.
Challenges of Working with Unlabeled Data
While some algorithms are capable of learning from unlabeled data through self-supervised or unsupervised learning techniques, these approaches often come with their own set of challenges. For instance:
- Lack of interpretability: Models trained on unlabeled data can be difficult to understand and interpret.
- Difficulty in evaluating performance: It's hard to measure the accuracy of a model when there is no clear notion of what constitutes a correct output.
- Inefficient use of resources: Training models on large amounts of unlabeled data can be computationally expensive and time-consuming.
The Importance of Human Labeling
To overcome these challenges, human labeling remains an essential step in preparing high-quality datasets for supervised learning. While machine learning algorithms have made significant progress in recent years, they still require human oversight to ensure that labels are accurate and relevant. In many cases, the cost of hiring humans to label data is offset by the benefits of improved model performance, reduced training time, and increased accuracy.
Best Practices for Collecting Labeled Data
To get the most out of labeled data in supervised learning, it's essential to follow best practices when collecting and labeling data. Here are a few tips:
- Collect high-quality data: Ensure that the data is relevant, accurate, and complete.
- Use domain expertise: Leverage the knowledge and expertise of professionals in the field to label data accurately.
- Implement quality control measures: Regularly review labels to ensure accuracy and consistency.
Conclusion
In conclusion, labeled data is a critical component in achieving accurate model performance in supervised learning. While working with unlabeled data can be challenging, human labeling remains an essential step in preparing high-quality datasets for training models. By following best practices for collecting and labeling data, organizations can unlock the full potential of their machine learning models and achieve better outcomes in various applications.
- Created by: Benjamin Kelly
- Created at: July 27, 2024, 11:21 p.m.
- ID: 4082