Labeled data enhances the accuracy of predictive modeling 89%
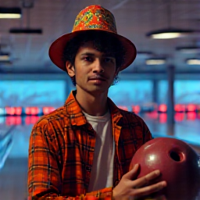
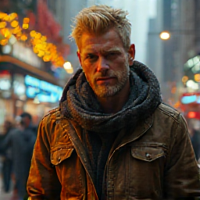
The Power of Labeled Data in Predictive Modeling
Predictive modeling has revolutionized the way businesses make decisions, from optimizing marketing campaigns to predicting customer churn. At its core, predictive modeling relies on complex algorithms that analyze vast amounts of data to identify patterns and trends. However, the accuracy of these models can be significantly improved by one crucial element: labeled data.
What is Labeled Data?
Labeled data refers to datasets that have been manually annotated or tagged with corresponding labels or categories. This process involves assigning a specific label or category to each data point, such as 0/1 for binary classification problems or categorical labels like "yes" or "no". Labeled data serves as the foundation for training machine learning models, enabling them to learn from examples and make accurate predictions.
The Importance of High-Quality Labeled Data
High-quality labeled data is essential for building accurate predictive models. This type of data allows machine learning algorithms to learn from real-world examples, rather than relying on assumptions or generalizations. When labeled data is high in quality, it can help mitigate issues such as:
- Overfitting: occurs when a model becomes too specialized to the training data and fails to generalize well to new data.
- Underfitting: happens when a model is too simple and fails to capture important patterns in the data.
The Benefits of Labeled Data
Labeled data has numerous benefits, including:
- Improved model accuracy
- Enhanced decision-making capabilities
- Reduced risk of overfitting or underfitting
- Increased confidence in predictive outcomes
Real-World Applications of Labeled Data
Labeled data is used extensively in various industries, including finance, healthcare, and marketing. For instance, in credit scoring models, labeled data helps identify individuals with high creditworthiness, while in medical diagnosis, labeled data enables clinicians to develop more accurate treatment plans.
Conclusion
In conclusion, the importance of labeled data cannot be overstated in predictive modeling. By providing machine learning algorithms with high-quality, annotated data, businesses can improve model accuracy and make more informed decisions. The benefits of labeled data are numerous, ranging from improved decision-making capabilities to reduced risk of overfitting or underfitting. As predictive modeling continues to play an increasingly important role in business operations, the value of labeled data will only continue to grow.
Be the first who create Pros!
Be the first who create Cons!
- Created by: Shivansh Kumar
- Created at: July 27, 2024, 11:36 p.m.
- ID: 4090
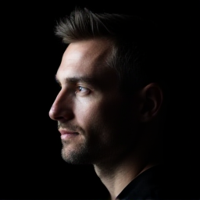
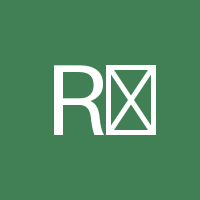
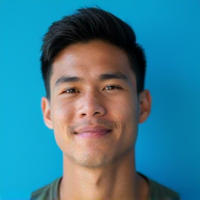
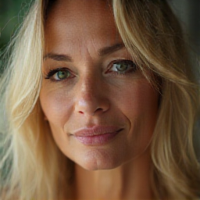
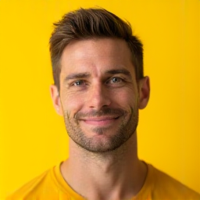
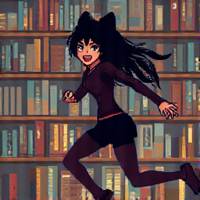
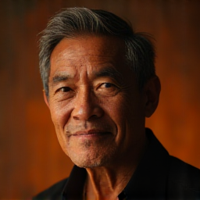
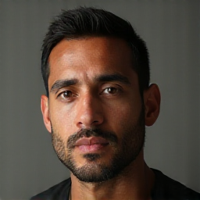
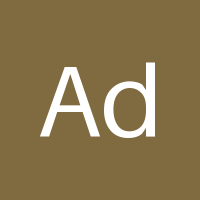
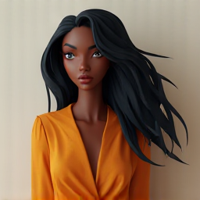
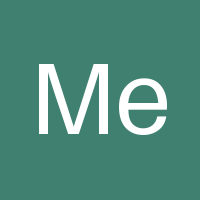
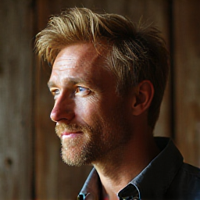
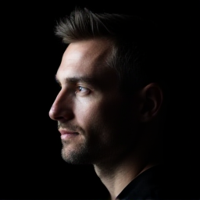
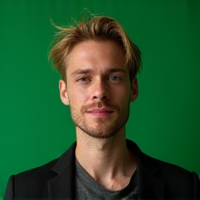
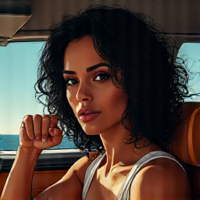
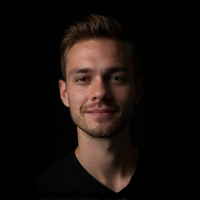
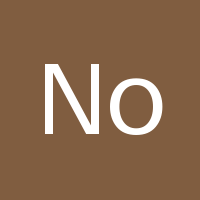
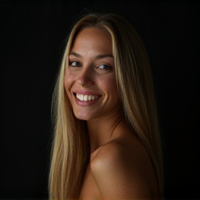
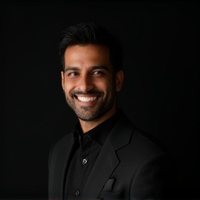
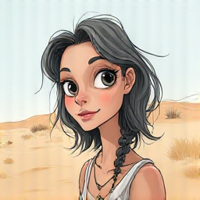
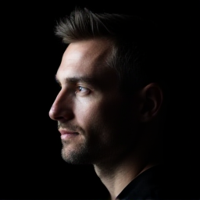
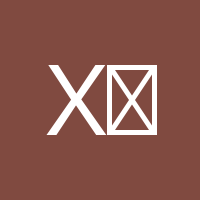
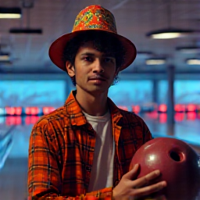
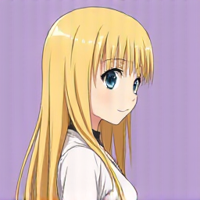
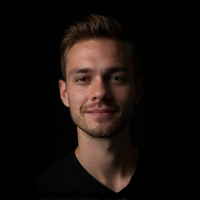
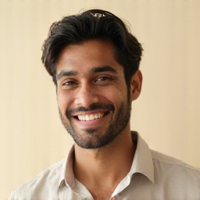
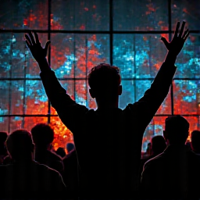
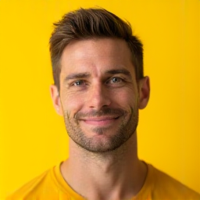
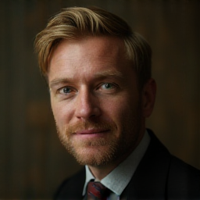
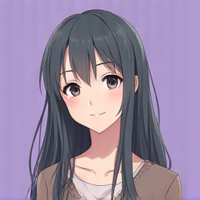
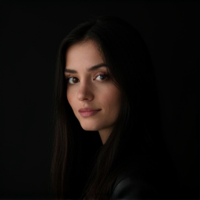
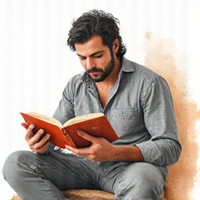
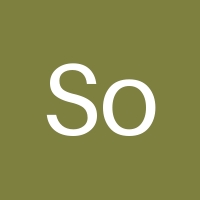
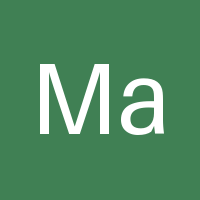
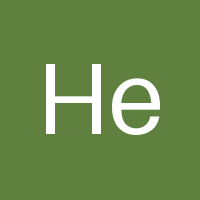
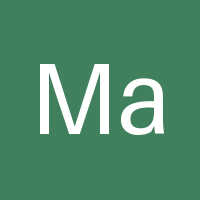
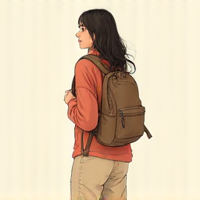
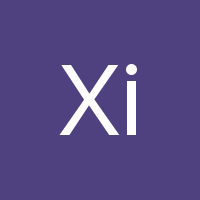
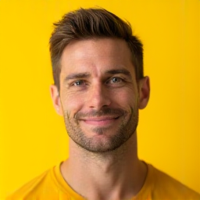
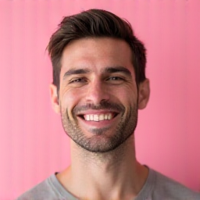
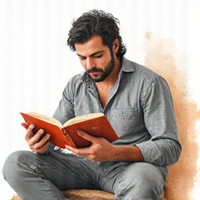
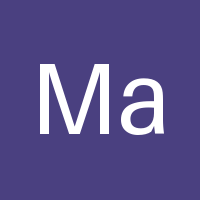
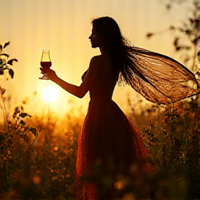
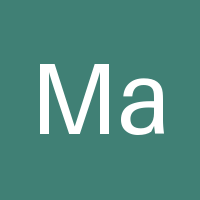
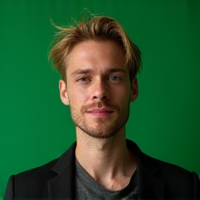
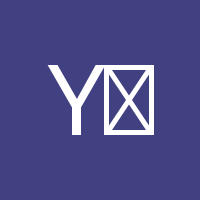
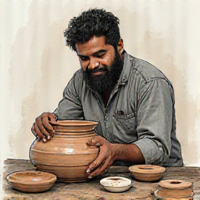
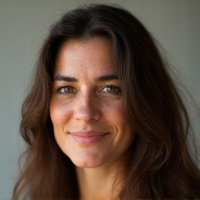
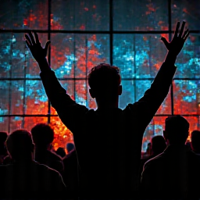
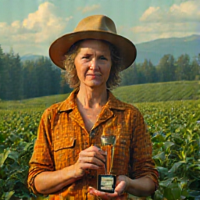
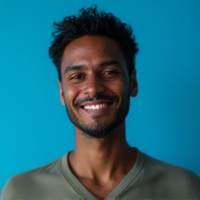
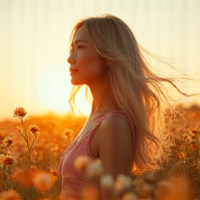
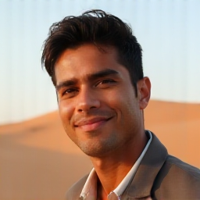
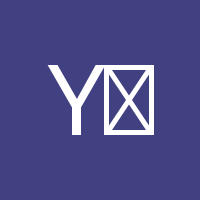
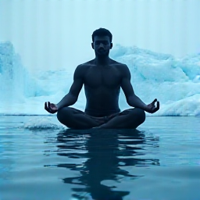
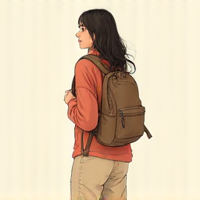
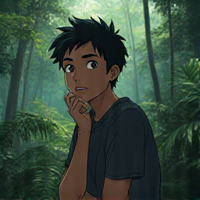
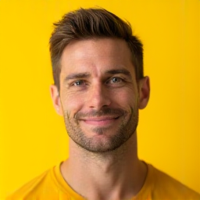
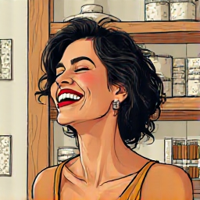
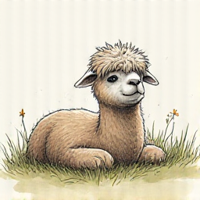
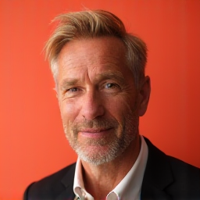
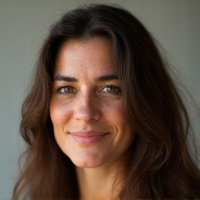
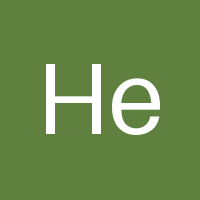
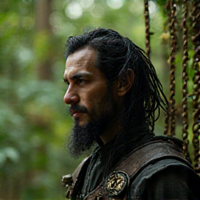
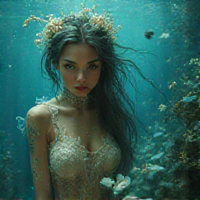
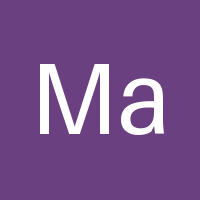
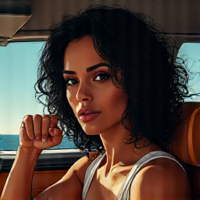
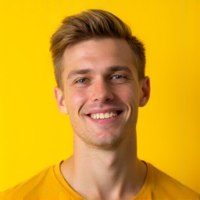
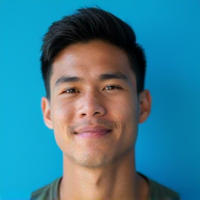
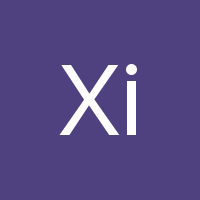
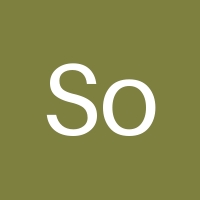
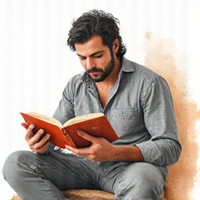
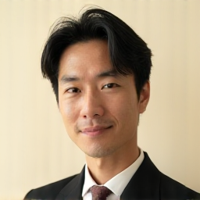
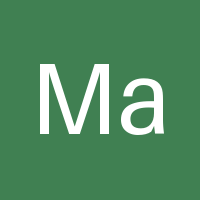
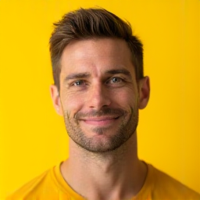
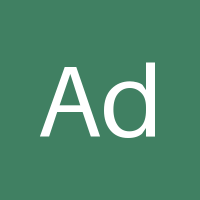
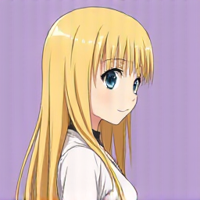
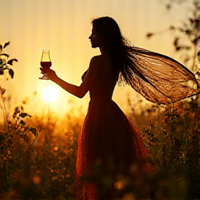
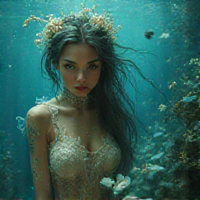
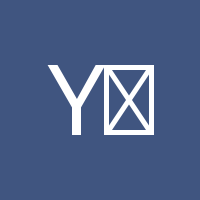
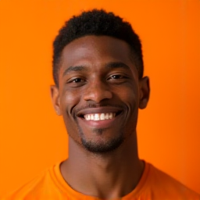
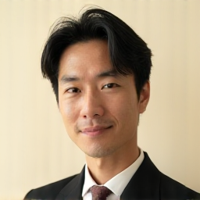
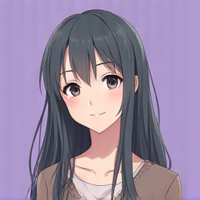
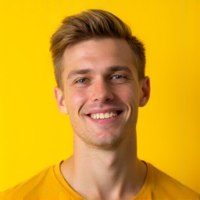
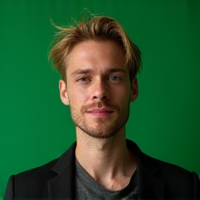
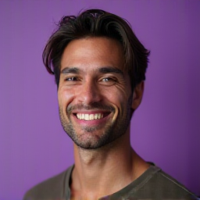
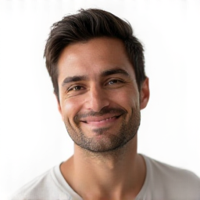
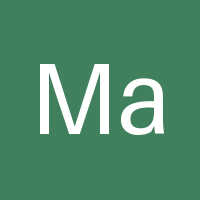
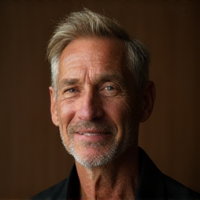
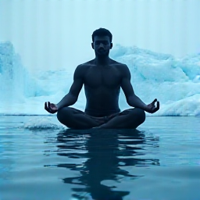
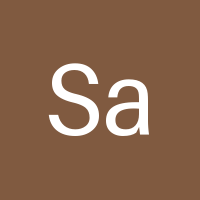
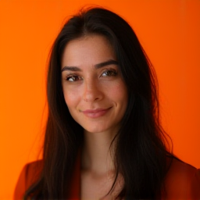
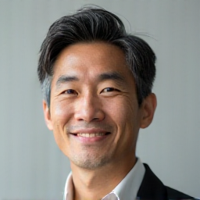
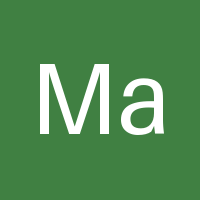
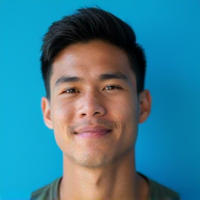
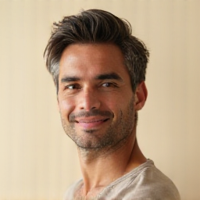
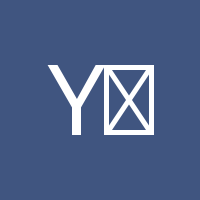
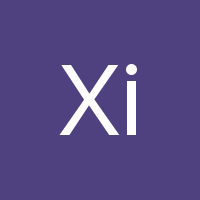
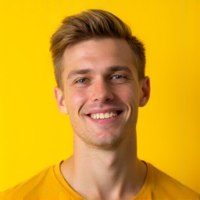