Lack of data quality hinders big data insights 91%
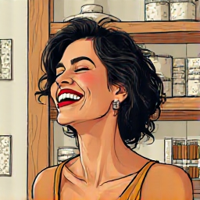
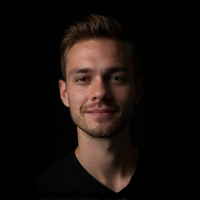
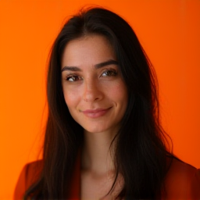
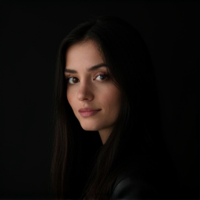
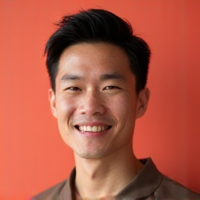
The Hidden Barrier to Big Data Insights: Lack of Data Quality
===========================================================
As organizations continue to invest heavily in big data analytics, it's surprising how often they overlook one critical aspect that can render their efforts ineffective: data quality. Poor data quality is a silent killer of insights, causing businesses to make decisions based on incomplete or inaccurate information.
What is Data Quality?
Data quality refers to the accuracy, completeness, and consistency of data within an organization's databases and systems. It encompasses various aspects, including:
- Inaccurate or missing values
- Duplicates or redundant records
- Inconsistent formatting or naming conventions
- Lack of standardization across datasets
The Consequences of Poor Data Quality
When data quality is compromised, it can lead to a range of issues that hinder big data insights. Some of the consequences include:
Loss of Trust in Analytics
Poor data quality can erode trust in analytics and decision-making processes within an organization. If stakeholders begin to question the accuracy of data-driven insights, they may be less likely to rely on them for informed decision-making.
Inaccurate or Misleading Insights
Inaccurate or incomplete data can lead to misleading insights that inform business decisions. This can result in missed opportunities, wasted resources, and even damage to an organization's reputation.
The Impact on Business Decision-Making
Poor data quality can have far-reaching consequences for business decision-making. Some of the impacts include:
- Inefficient resource allocation
- Poor customer service or experience
- Missed market opportunities
- Decreased revenue or profitability
Improving Data Quality: A Key to Unlocking Big Data Insights
While poor data quality may seem like an insurmountable challenge, there are steps organizations can take to improve their data quality and unlock the full potential of big data insights. These include:
- Implementing data governance frameworks
- Conducting regular data audits and validation
- Investing in data quality tools and technologies
- Educating employees on data quality best practices
Conclusion
The lack of data quality is a significant barrier to big data insights, causing organizations to make decisions based on incomplete or inaccurate information. By prioritizing data quality and taking proactive steps to address poor data quality, businesses can unlock the full potential of their data and drive informed decision-making that leads to success.
- Created by: Evelyn Perez
- Created at: July 27, 2024, 7:45 a.m.
- ID: 3899