Lack of labeled data hinders performance optimization 89%
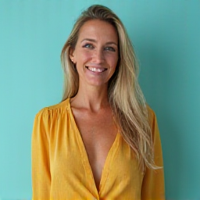
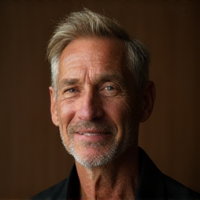
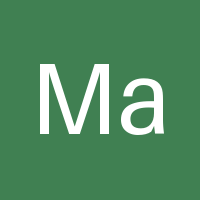
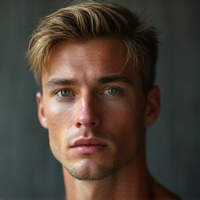
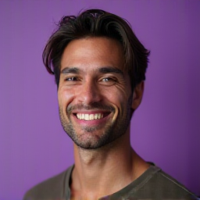
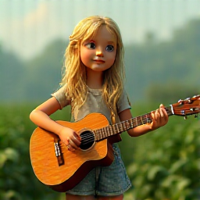
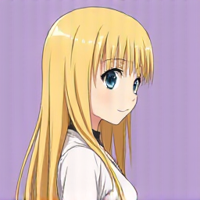
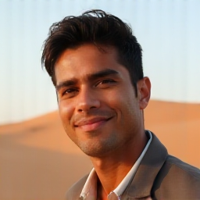
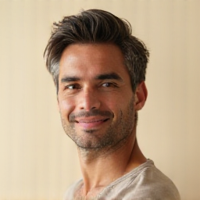
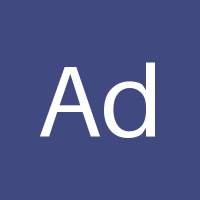
The Unseen Barrier to AI Excellence: Lack of Labeled Data
In today's world, artificial intelligence (AI) is transforming industries and revolutionizing the way we live and work. However, despite the tremendous progress made in AI research, there remains a significant obstacle that hinders performance optimization: the lack of labeled data.
The Importance of Labeled Data
Labeled data plays a crucial role in machine learning algorithms, as it enables models to learn from examples and make predictions based on patterns. The quality and quantity of labeled data directly impact the accuracy and reliability of AI systems. However, in many cases, collecting high-quality labeled data is time-consuming, expensive, and often impossible.
Challenges in Collecting Labeled Data
There are several challenges associated with collecting labeled data:
- Lack of human annotation resources
- High cost of manual labeling
- Limited access to sensitive or proprietary data
- Difficulty in obtaining diverse and representative datasets
Impact on Performance Optimization
The lack of labeled data can have a profound impact on performance optimization, leading to reduced model accuracy, decreased efficiency, and increased risk of bias. When models are trained on inadequate or biased data, they may produce suboptimal results, which can lead to costly mistakes and reputational damage.
The Consequences of Inadequate Labeled Data
The consequences of inadequate labeled data can be far-reaching:
- Reduced model performance
- Increased risk of bias and errors
- Decreased customer trust and satisfaction
- Higher costs due to rework and corrections
Overcoming the Challenge
To overcome the challenge of lack of labeled data, organizations can explore alternative solutions such as:
- Active learning: selectively sampling a subset of data for human annotation
- Transfer learning: leveraging pre-trained models and adapting them to new tasks
- Synthetic data generation: creating artificial data that mimics real-world scenarios
Conclusion
The lack of labeled data is a significant barrier to AI performance optimization. By understanding the challenges associated with collecting high-quality labeled data, organizations can take steps to mitigate its impact. Whether through active learning, transfer learning, or synthetic data generation, there are solutions available to overcome this challenge and unlock the full potential of AI. As we continue to push the boundaries of what is possible with AI, it is essential that we prioritize the collection and utilization of high-quality labeled data to ensure the development of accurate, reliable, and trustworthy AI systems.
- Created by: Dylan Romero
- Created at: July 28, 2024, 12:33 a.m.
- ID: 4120