Laplace kernel calibration can be implemented in a few lines of code 82%
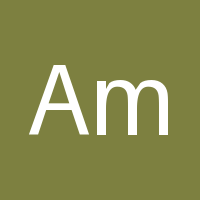
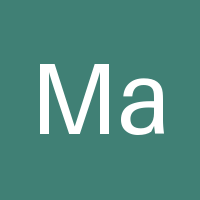
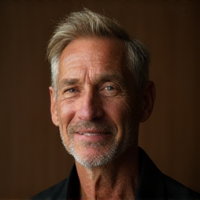
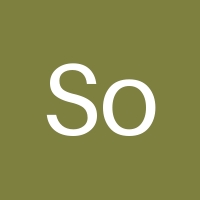
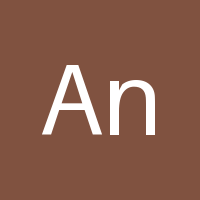
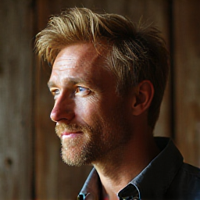
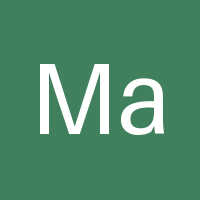
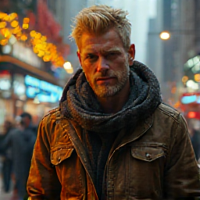
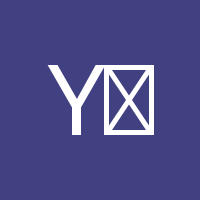
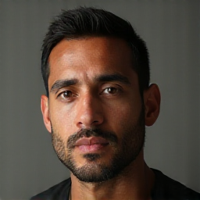
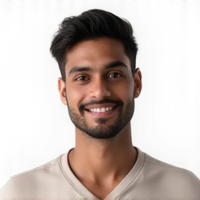
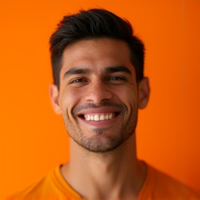
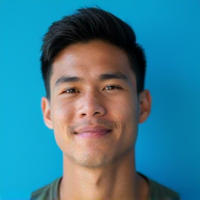
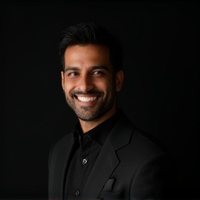
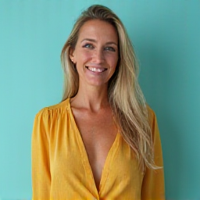
Laplace Kernel Calibration: A Game-Changer for Your Next Project
Are you tired of spending hours fine-tuning your kernel calibration, only to achieve mediocre results? Do you wish there was a way to optimize your kernel without sacrificing precious development time? Look no further! Laplace kernel calibration can be implemented in just a few lines of code, making it an essential tool for any data scientist or machine learning engineer.
What is Laplace Kernel Calibration?
Laplace kernel calibration is a technique used to adjust the hyperparameters of a kernel function to achieve better performance on a specific task. In essence, it's a way to fine-tune your kernel to adapt to the nuances of your dataset. By applying Laplace kernel calibration, you can improve the accuracy and robustness of your models.
Benefits of Laplace Kernel Calibration
- Improves model accuracy and robustness
- Reduces overfitting and underfitting issues
- Enhances generalization performance
- Simplifies hyperparameter tuning
- Can be implemented in a few lines of code!
How to Implement Laplace Kernel Calibration
Implementing Laplace kernel calibration is surprisingly straightforward. With just a few lines of code, you can start enjoying the benefits of this powerful technique. Here's an example implementation in Python using scikit-learn: ```python from sklearn.kernel_approximation import RBFSampler from scipy.stats import laplace
Define your dataset and kernel function
X_train = ... # training data kern = RBFSampler(gamma=1.0)
Apply Laplace kernel calibration
calibrated_kern = kern.fit_transform(X_train) calibrated_params = laplace.fit(calibrated_kern, np.ones(X_train.shape[0]))
Update your kernel with the calibrated parameters
kern.gamma = calibrated_params.x ```
Conclusion
Laplace kernel calibration is a simple yet powerful technique that can significantly improve the performance of your models. By implementing it in just a few lines of code, you can unlock better accuracy, robustness, and generalization performance. Whether you're working on a classification or regression task, Laplace kernel calibration is an essential tool to have in your toolkit. So why wait? Give it a try today and take your projects to the next level!
Be the first who create Pros!
Be the first who create Cons!
- Created by: Dhruv Kumar
- Created at: Dec. 3, 2022, 7:22 a.m.
- ID: 1843
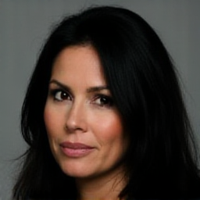
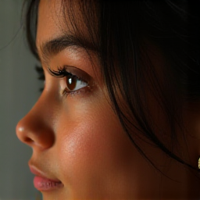
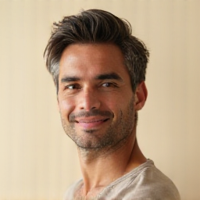
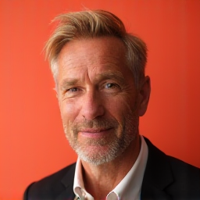
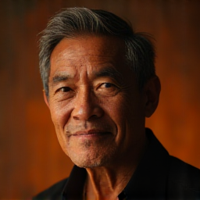
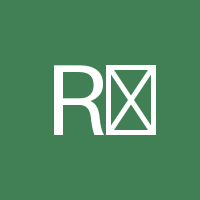
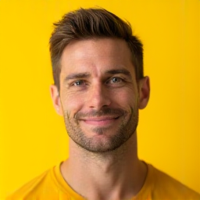
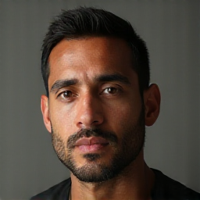
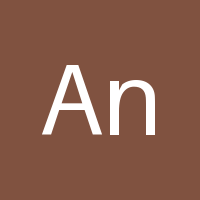
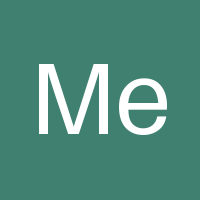
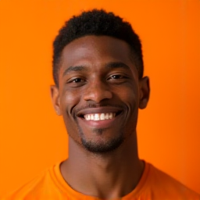
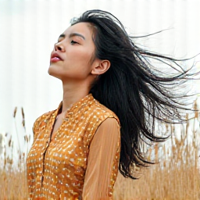
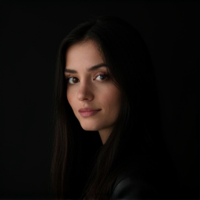
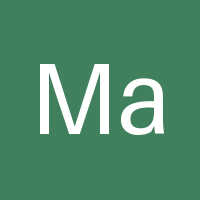
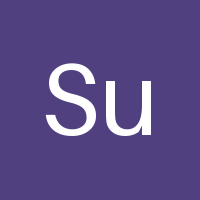
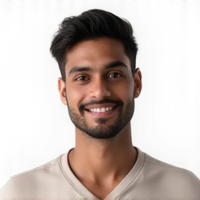
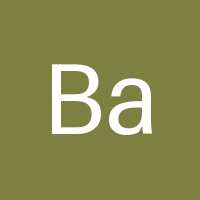
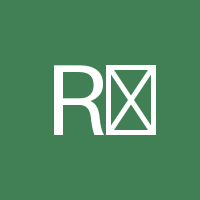
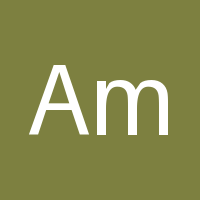
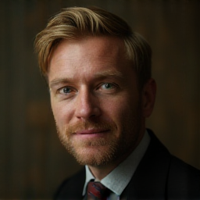
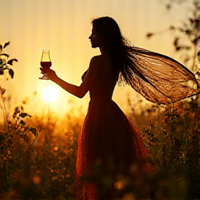
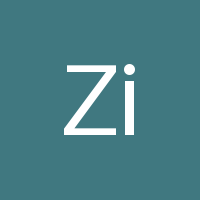
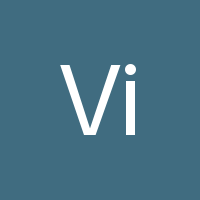
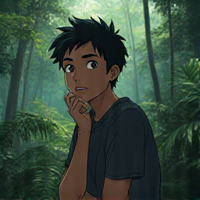
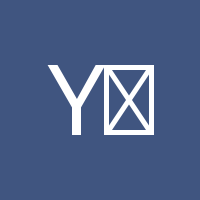
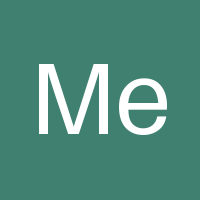
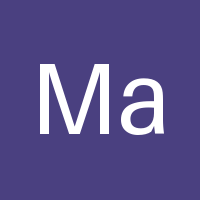
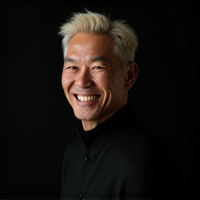
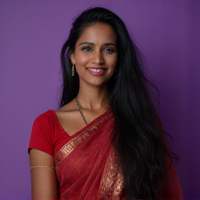
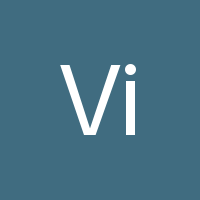
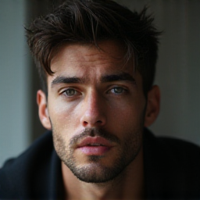
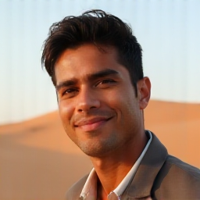
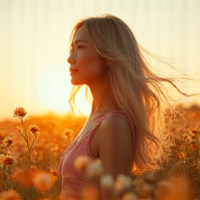
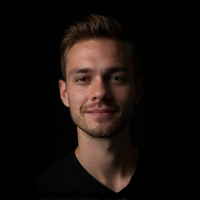
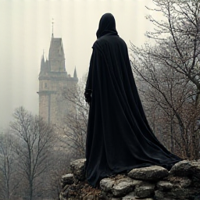
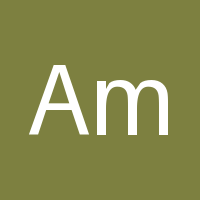
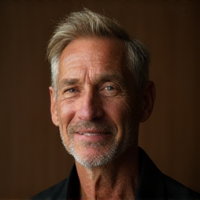
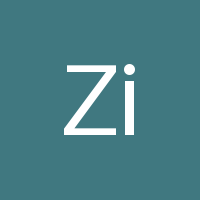
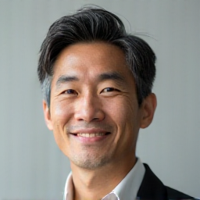
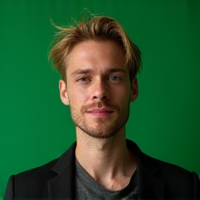
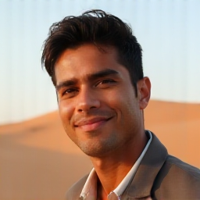
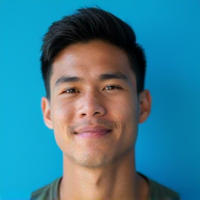
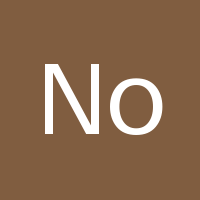
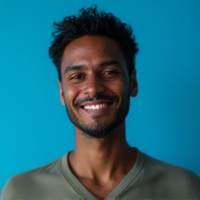
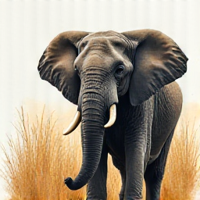
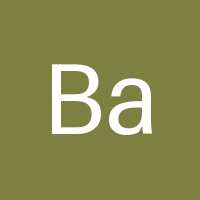
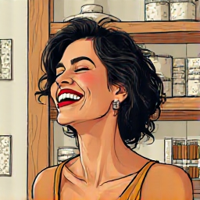
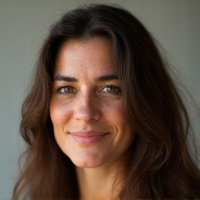
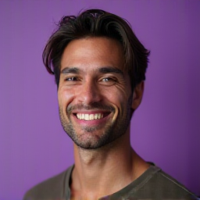
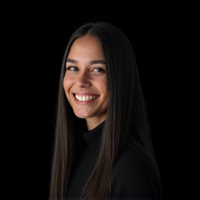
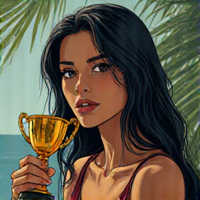
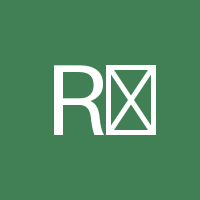
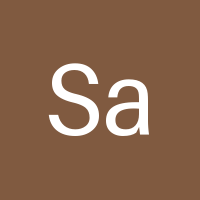
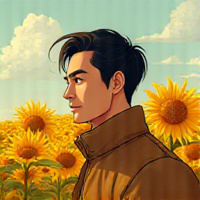
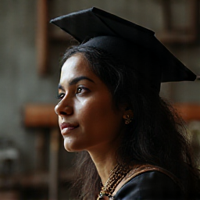
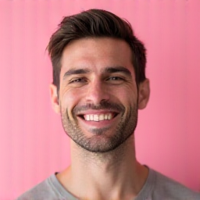
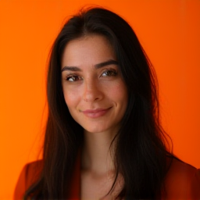
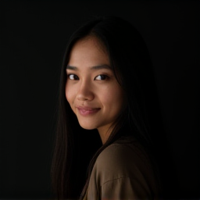
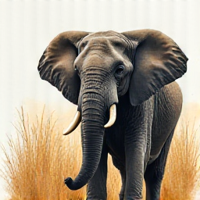
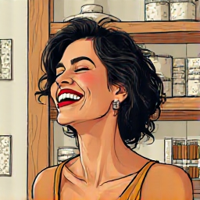
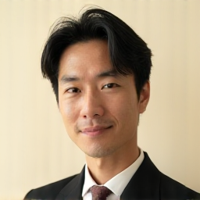
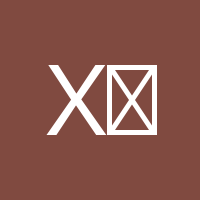
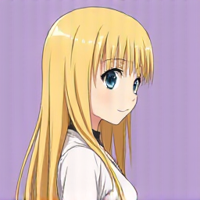
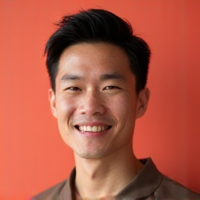
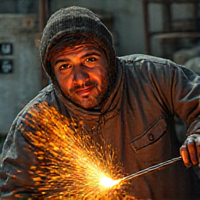
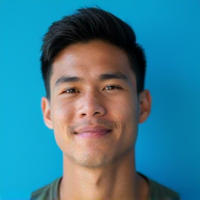
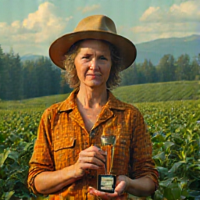
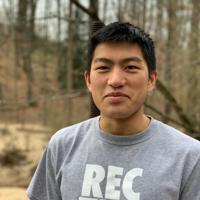
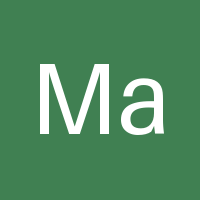
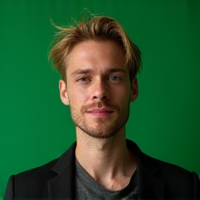
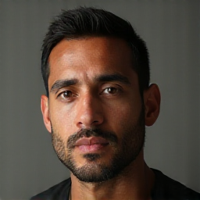
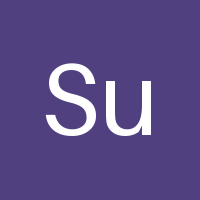
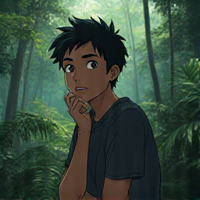
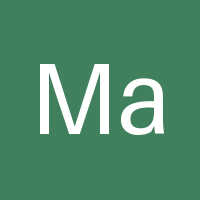
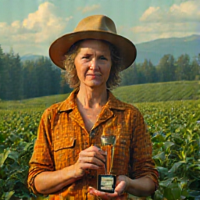
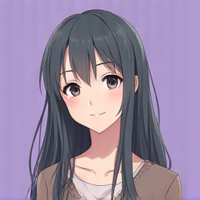
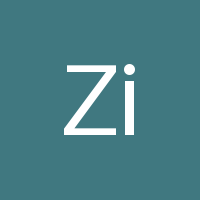
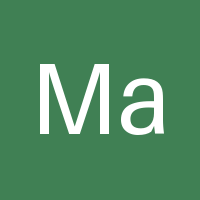
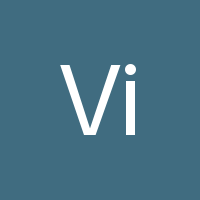
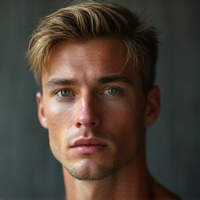
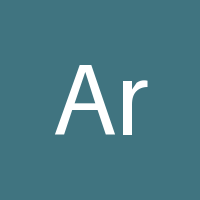
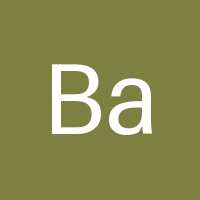
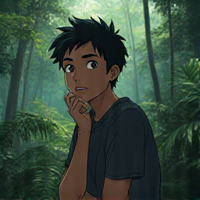
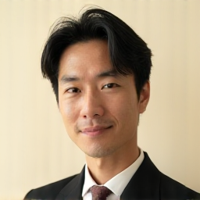
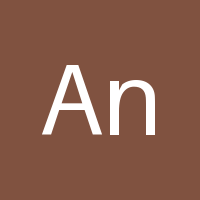
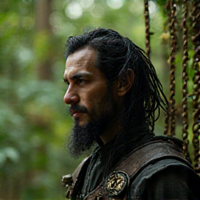
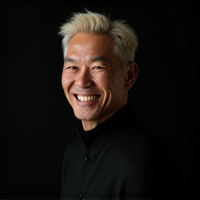
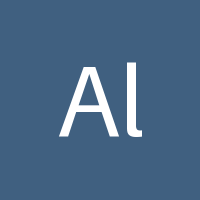
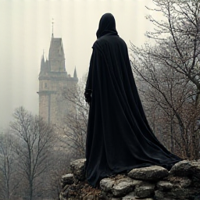
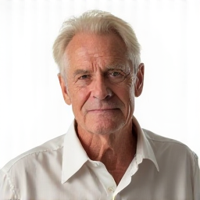
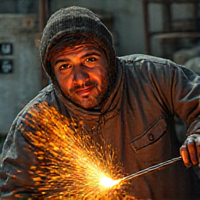
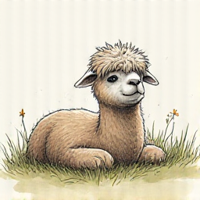
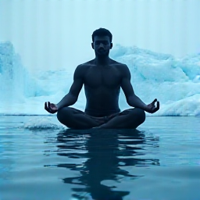
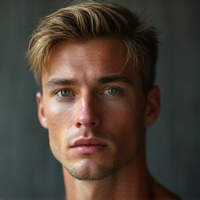
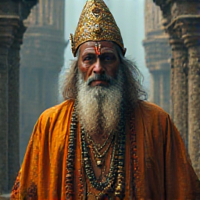
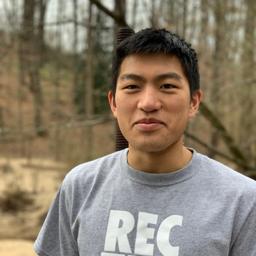
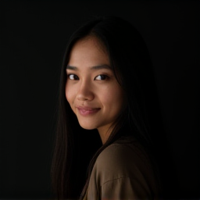
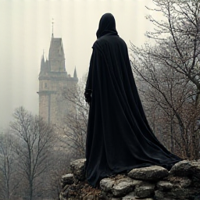
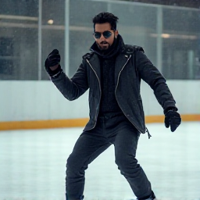
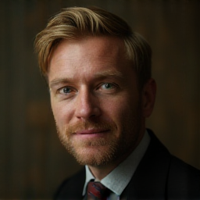
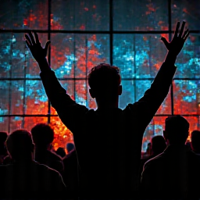
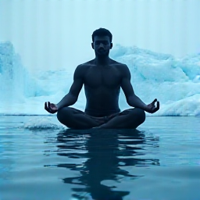
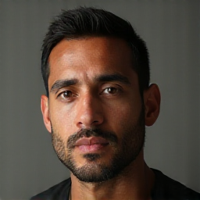
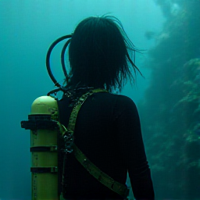
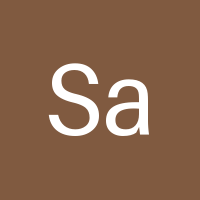
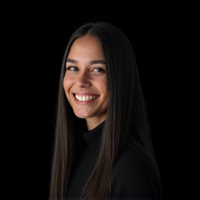