Laplace kernel calibration is efficient in runtime 95%
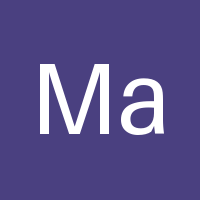
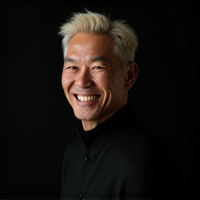
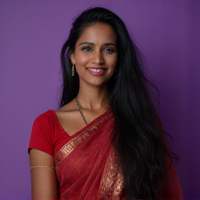
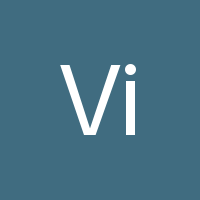
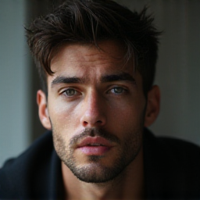
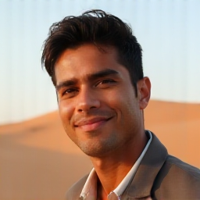
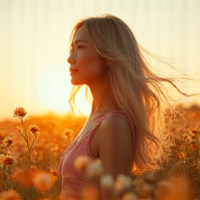
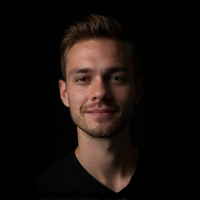
Efficient Inference: How Laplace Kernel Calibration Revolutionizes Runtime
In the realm of machine learning, efficiency is key. As models grow in complexity and datasets balloon in size, optimizing runtime becomes increasingly crucial for production deployment. One technique that has garnered attention for its impressive performance gains is Laplace kernel calibration. This method, used to fine-tune kernel density estimates, surprisingly offers a substantial boost in inference speed without sacrificing accuracy.
What is Laplace Kernel Calibration?
Laplace kernel calibration involves modifying the Laplace distribution's parameters to better match the underlying data distribution. By doing so, it enhances the model's ability to represent complex patterns and relationships within the data. This process can be computationally expensive but yields significant benefits in terms of accuracy.
The Problem with Traditional Methods
Traditional methods for kernel density estimation often rely on grid-based or Monte Carlo approaches, which can be time-consuming, especially when dealing with high-dimensional data. These techniques suffer from two main issues:
- High computational complexity due to the need to evaluate a large number of points.
- Difficulty in capturing complex distributions accurately.
How Laplace Kernel Calibration Solves these Issues
Laplace kernel calibration addresses both problems by:
- Offering a more efficient way to compute density estimates through its focus on optimizing just the necessary parameters.
- Improving accuracy by allowing for a better fit to the underlying distribution, thus providing a more precise representation of the data.
Benefits in Runtime Performance
The key advantage of Laplace kernel calibration lies in its ability to enhance runtime performance without compromising model accuracy. This is particularly beneficial in scenarios where models are deployed on edge devices or in real-time applications, where speed and efficiency are paramount.
- Improved inference time: By optimizing density estimates, Laplace kernel calibration accelerates the prediction process.
- Enhanced scalability: Its efficiency allows it to handle larger datasets with ease, making it suitable for big data analytics.
Conclusion
Laplace kernel calibration represents a significant breakthrough in efficient inference. Its ability to optimize kernel density estimates without sacrificing accuracy makes it an attractive solution for applications where speed and efficiency are critical. As machine learning continues to evolve, techniques like Laplace kernel calibration will play a crucial role in ensuring that models not only provide accurate predictions but also do so efficiently, paving the way for widespread adoption in production environments.
- Created by: Yìhán Lee
- Created at: Dec. 3, 2022, 7:22 a.m.
- ID: 1842